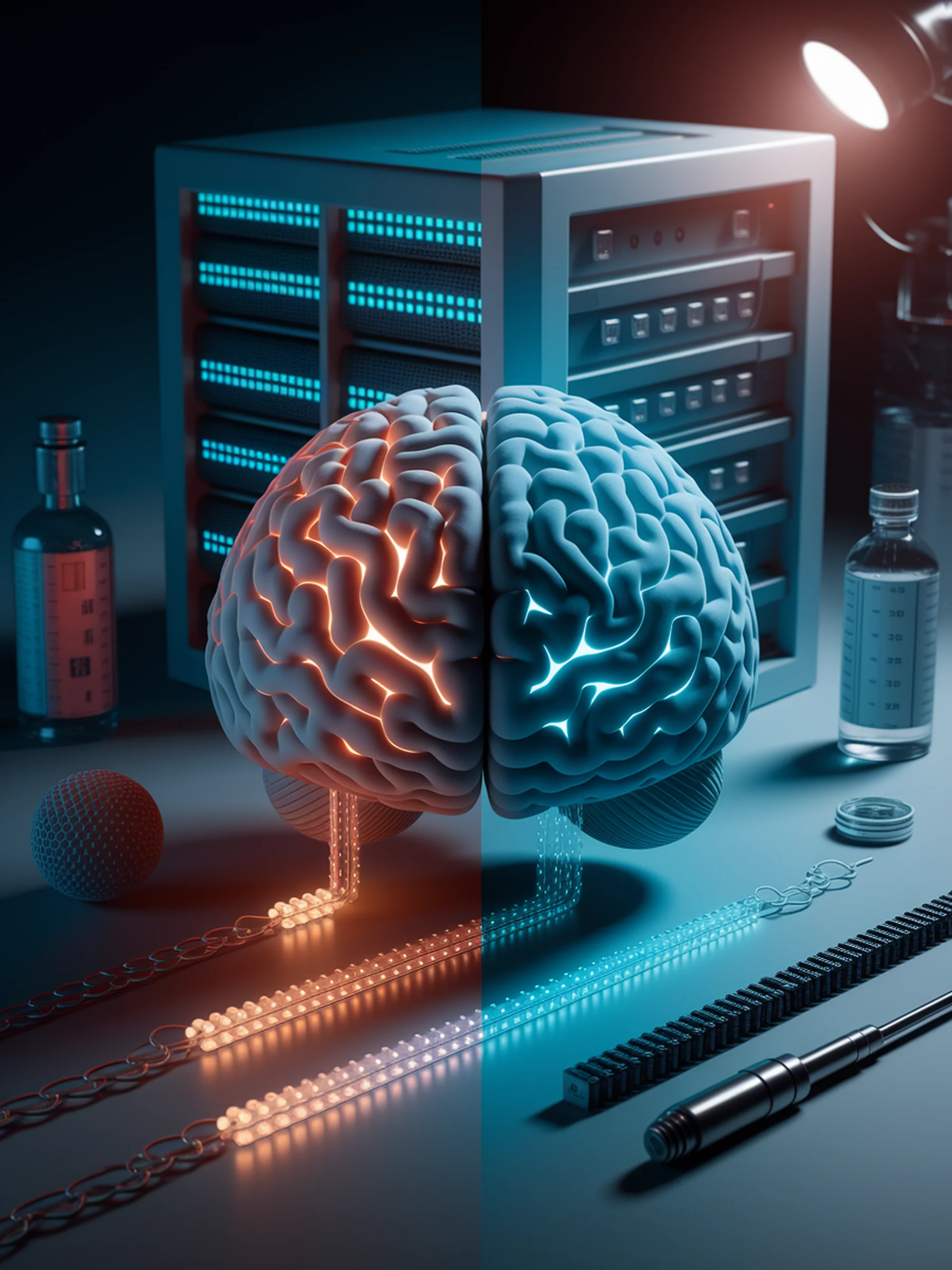
The Hidden Memory Problem in LLMs
Understanding skewed memorization patterns and their security implications
This research quantifies how LLMs memorize training data in highly skewed patterns, posing significant privacy and security risks.
- Uneven memorization: Training data is not equally memorized, with some content reproduced at much higher rates
- Length correlation: Longer sequences show exponentially higher memorization probabilities
- Dataset insights: Memorization increases with training duration but decreases with larger datasets
- Practical metrics: Introduces new ways to measure and decompose memorization to identify risky content
These findings matter for security professionals developing safeguards against unintended data exposure in AI systems. Understanding memorization patterns helps create more privacy-preserving models while maintaining performance.
Skewed Memorization in Large Language Models: Quantification and Decomposition