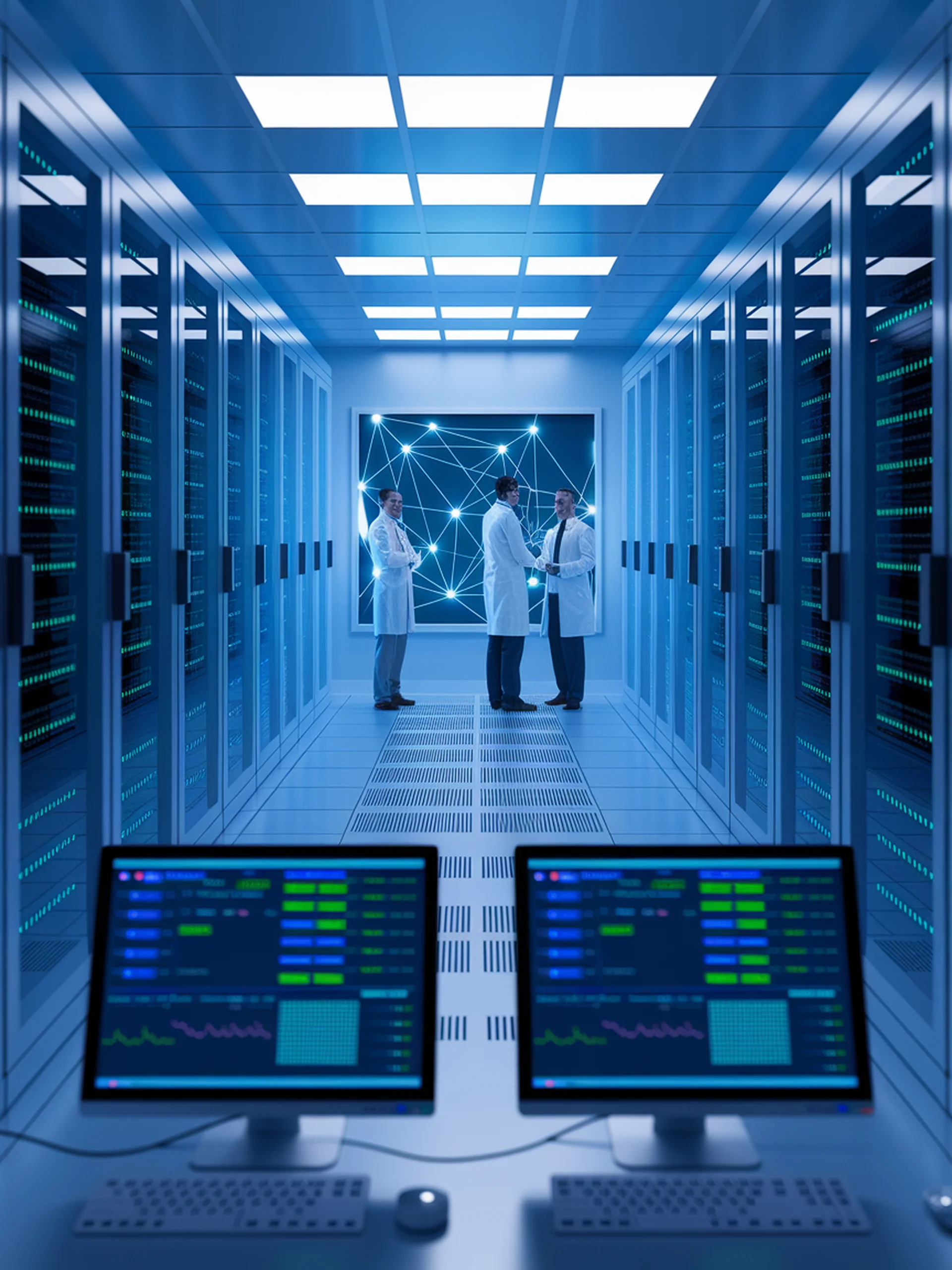
Protecting Patient Data in Collaborative AI
Using LoRA to reduce unintended data memorization in federated learning
This research addresses a critical privacy challenge in federated learning for LLMs: preventing models from memorizing and potentially exposing sensitive training data.
Key findings:
- LLMs trained via federated learning can inadvertently memorize and reproduce sensitive data when prompted
- Low-Rank Adaptation (LoRA) significantly reduces unintended memorization compared to full fine-tuning
- The approach maintains model utility while enhancing privacy protection
- Especially valuable for medical applications where patient data confidentiality is paramount
For healthcare organizations, this research offers a practical approach to collaborate on AI development while better safeguarding patient information and meeting compliance requirements.
Mitigating Unintended Memorization with LoRA in Federated Learning for LLMs