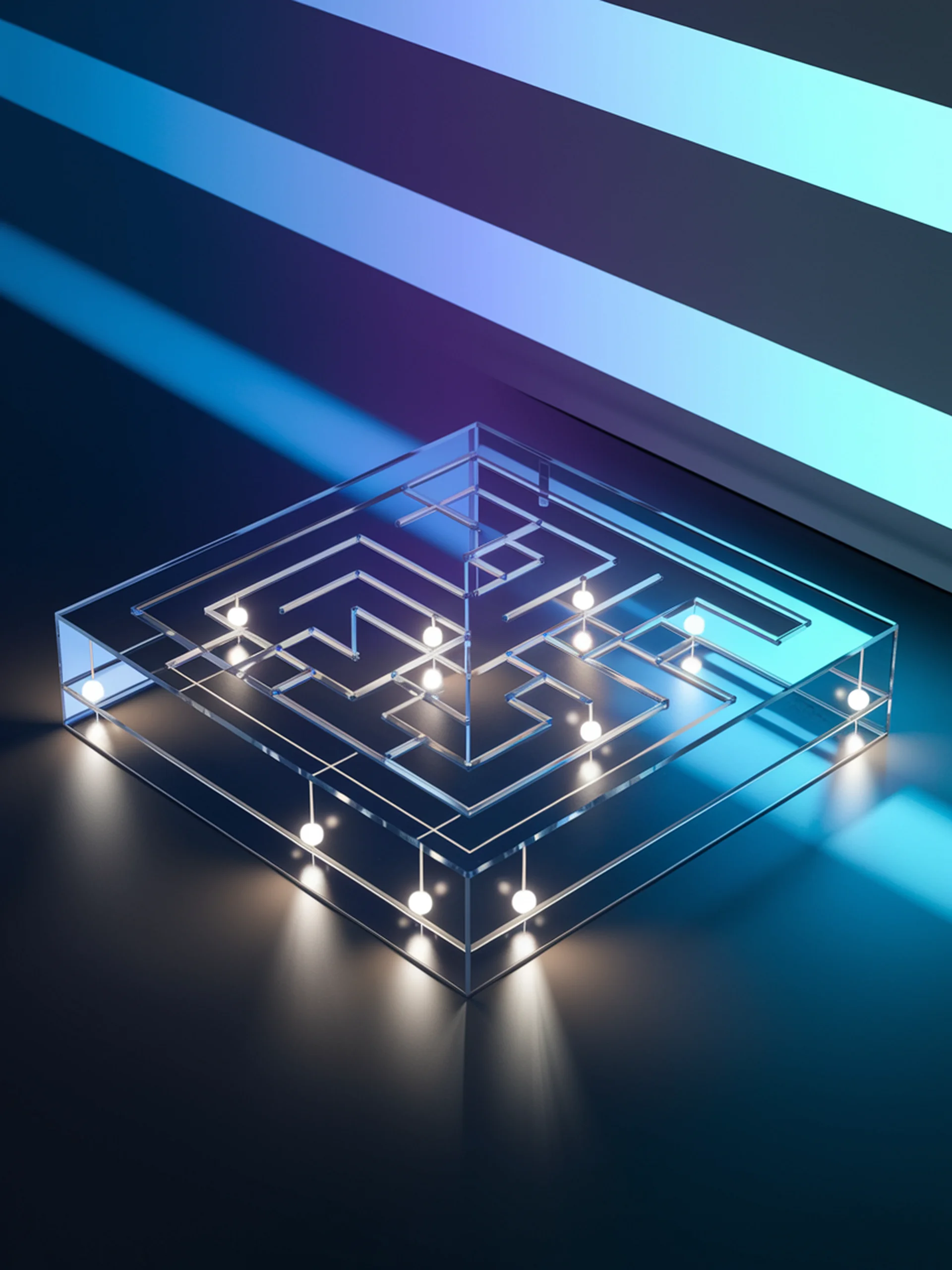
Making LLMs Transparent by Design
Concept Bottleneck LLMs for Interpretable AI
CB-LLMs introduce a framework that builds interpretability directly into language models, rather than relying on after-the-fact explanations, improving transparency and trustworthiness.
- Creates models that explain why they make predictions using meaningful concepts
- Improves safety and trust by allowing visibility into model reasoning
- Achieves strong performance on both text classification and generation tasks
- Enables identification of harmful content through transparent reasoning paths
This research matters for security professionals because it offers a path to AI systems that can be audited, understood, and verified—essential requirements for high-risk applications where unexplainable decisions are unacceptable.