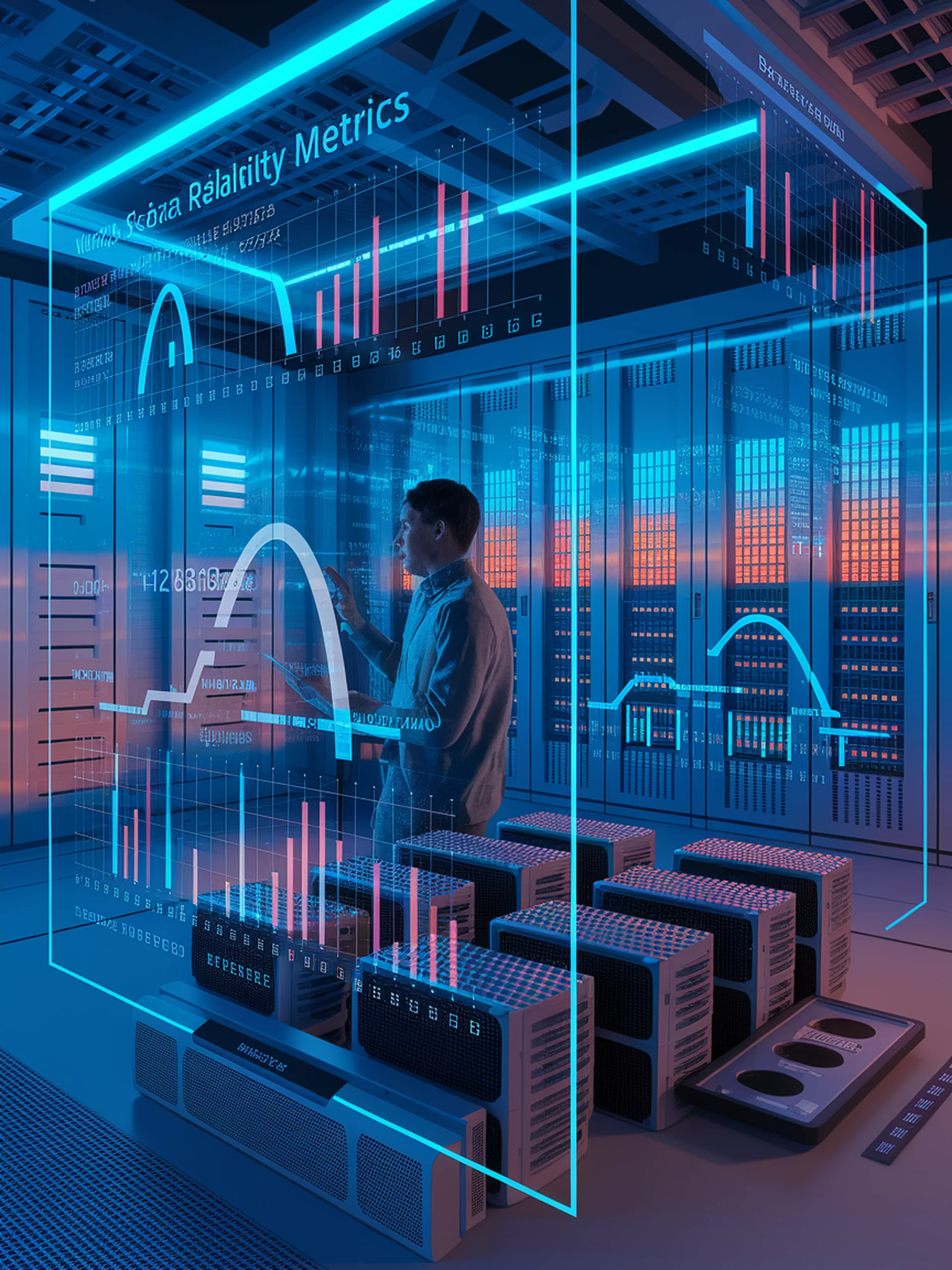
Trust at Scale: Evaluating LLM Reliability
A framework for assessing how much we can trust AI judgments
This research introduces a rigorous framework for evaluating the reliability of LLM judgments using statistical methods derived from psychometrics.
- Demonstrates that LLMs can provide inconsistent judgments even in deterministic settings
- Introduces McDonald's omega as a reliability metric for AI systems
- Reveals that multiple samples from LLMs significantly improve judgment reliability
- Highlights critical security implications for systems relying on single LLM outputs
For security professionals, this research provides essential insights into quantifying and mitigating risks when deploying LLMs in high-stakes decision-making scenarios. The framework enables more robust reliability testing before deployment.