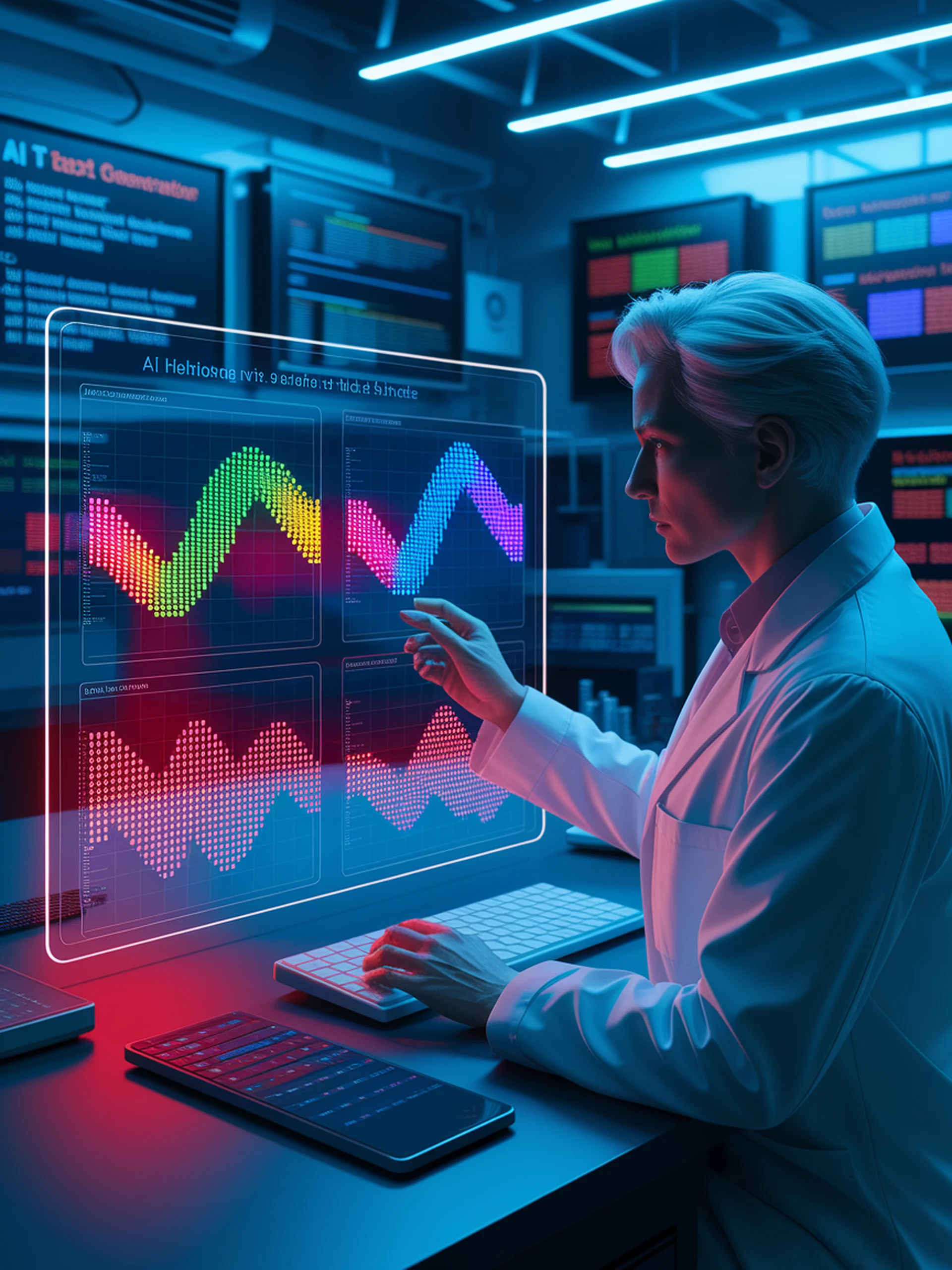
Detecting Hallucinations in LLMs
A novel approach to measuring distribution shifts for hallucination detection
HalluShift introduces a new methodology to identify when Large Language Models generate incorrect information while still producing coherent-looking responses.
- Analyzes internal dynamics of LLMs during text generation to detect hallucinations
- Demonstrates that hallucination events create measurable distribution shifts in model behavior
- Provides a detection framework that works across different models and domains
- Offers a more robust approach to identifying potential misinformation in AI-generated content
This research is critical for security as it addresses one of the main vulnerabilities in deploying LLMs for sensitive applications, reducing the risk of AI systems inadvertently spreading misinformation or being exploited through prompt engineering.
HalluShift: Measuring Distribution Shifts towards Hallucination Detection in LLMs