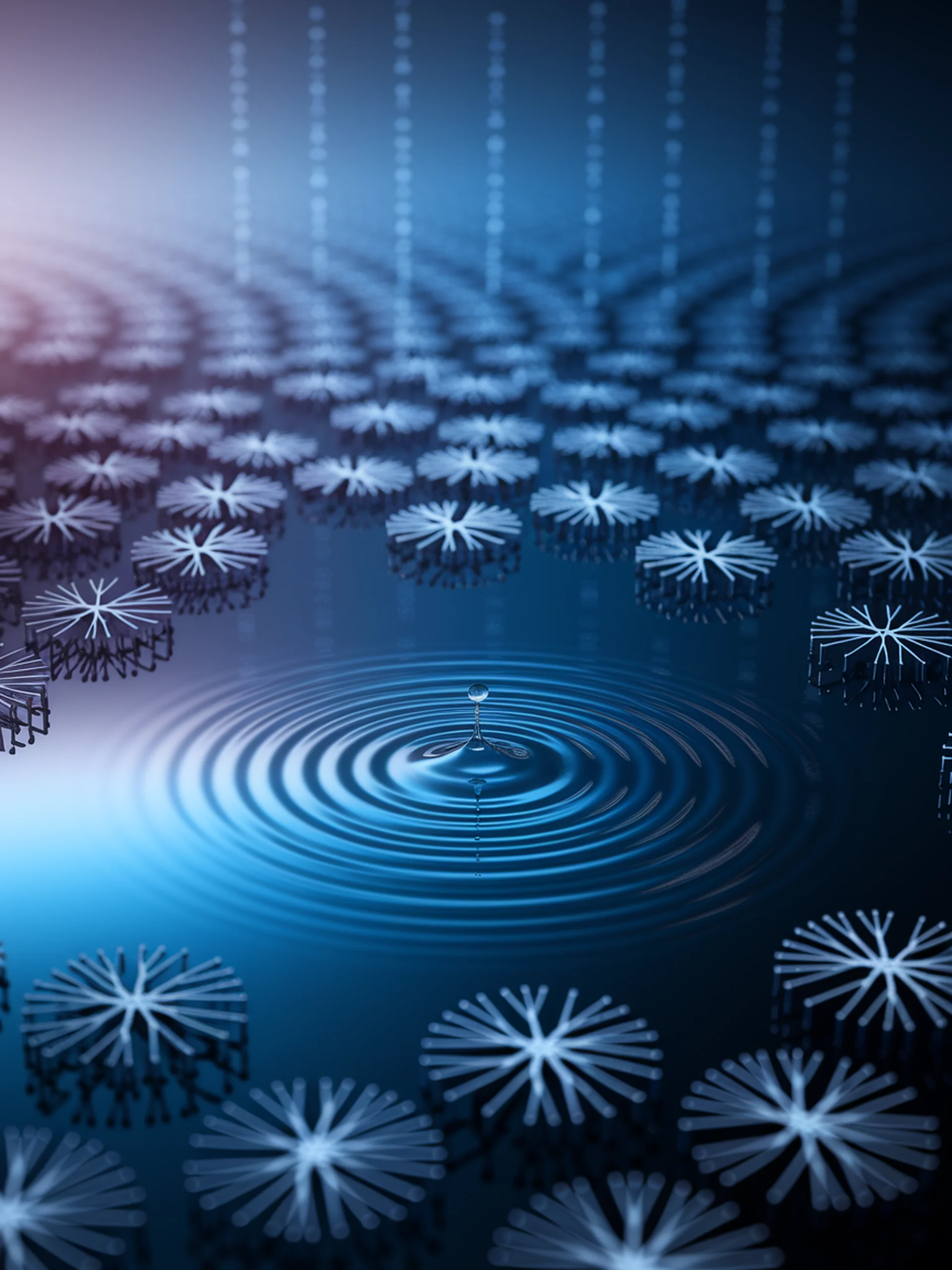
The Memory Ripple Effect in LLMs
How new information spreads through language models — and how to control it
This research reveals how learning new facts in Large Language Models creates unintended knowledge contamination, affecting unrelated contexts through a "priming effect".
- New information can cause models to inappropriately apply that knowledge elsewhere
- The contamination follows patterns that can be systematically studied
- Researchers developed techniques to dilute unwanted knowledge propagation
- This work demonstrates methods to mitigate hallucination and factual errors
For security professionals, this research provides critical insights into controlling knowledge boundaries in LLMs, reducing potential security risks from contaminated model outputs, and developing more trustworthy AI systems.