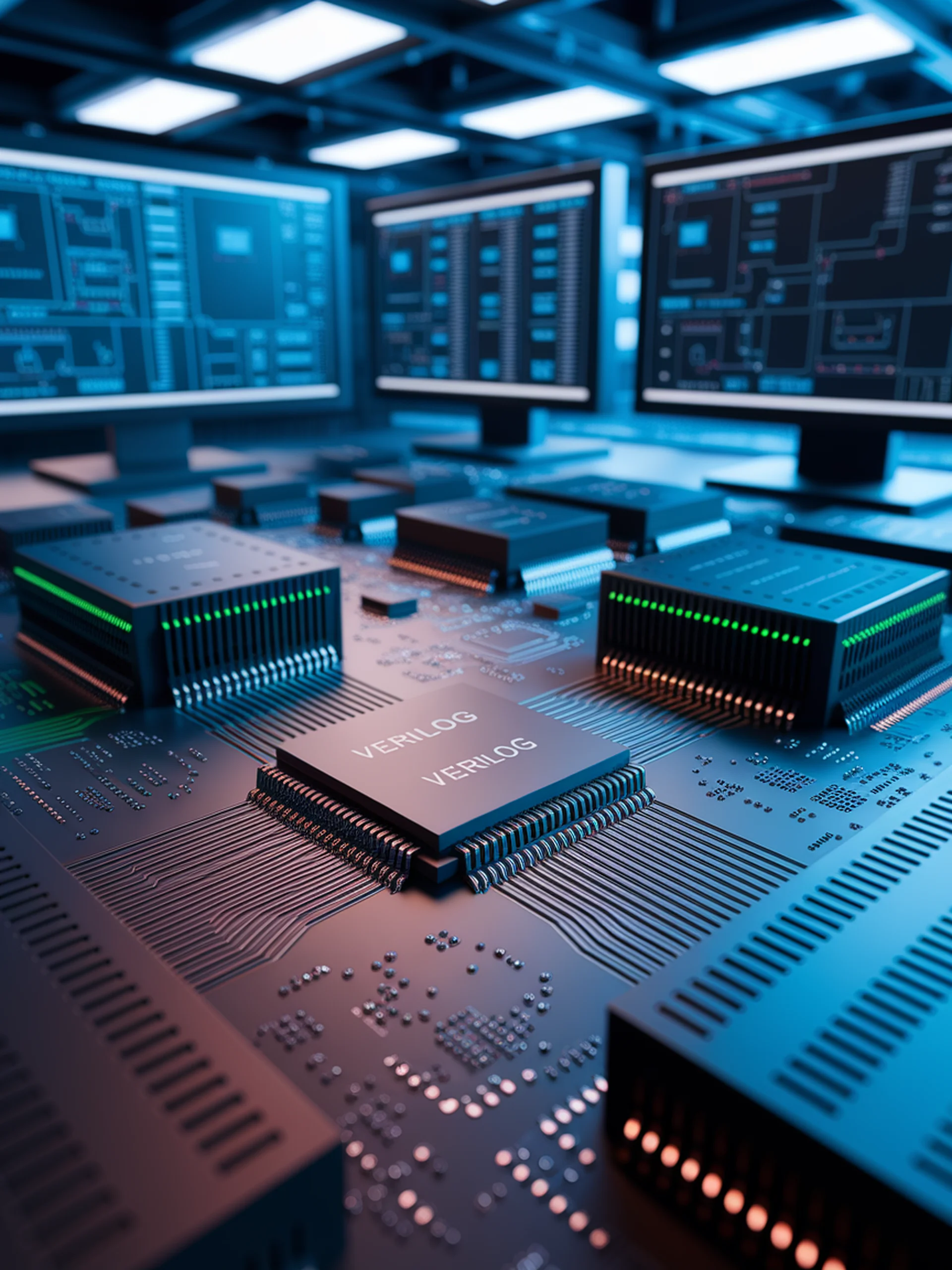
Boosting Verilog Code Generation with LLMs
Overcoming training data limitations through code-structure-guided reinforcement learning
This research advances RTL design automation by developing a specialized approach for generating Verilog code with Large Language Models despite limited training data.
- Introduces a novel code-structure-guided reinforcement learning technique for Verilog generation
- Addresses the significant challenge of limited public Verilog code compared to software code
- Enhances LLM capability to capture Verilog-specific syntax and design patterns
- Demonstrates improved quality of automatically generated hardware designs
This innovation has significant implications for hardware engineering teams by potentially accelerating RTL design processes, reducing development time, and enabling more efficient hardware development workflows.
Large Language Model for Verilog Generation with Code-Structure-Guided Reinforcement Learning