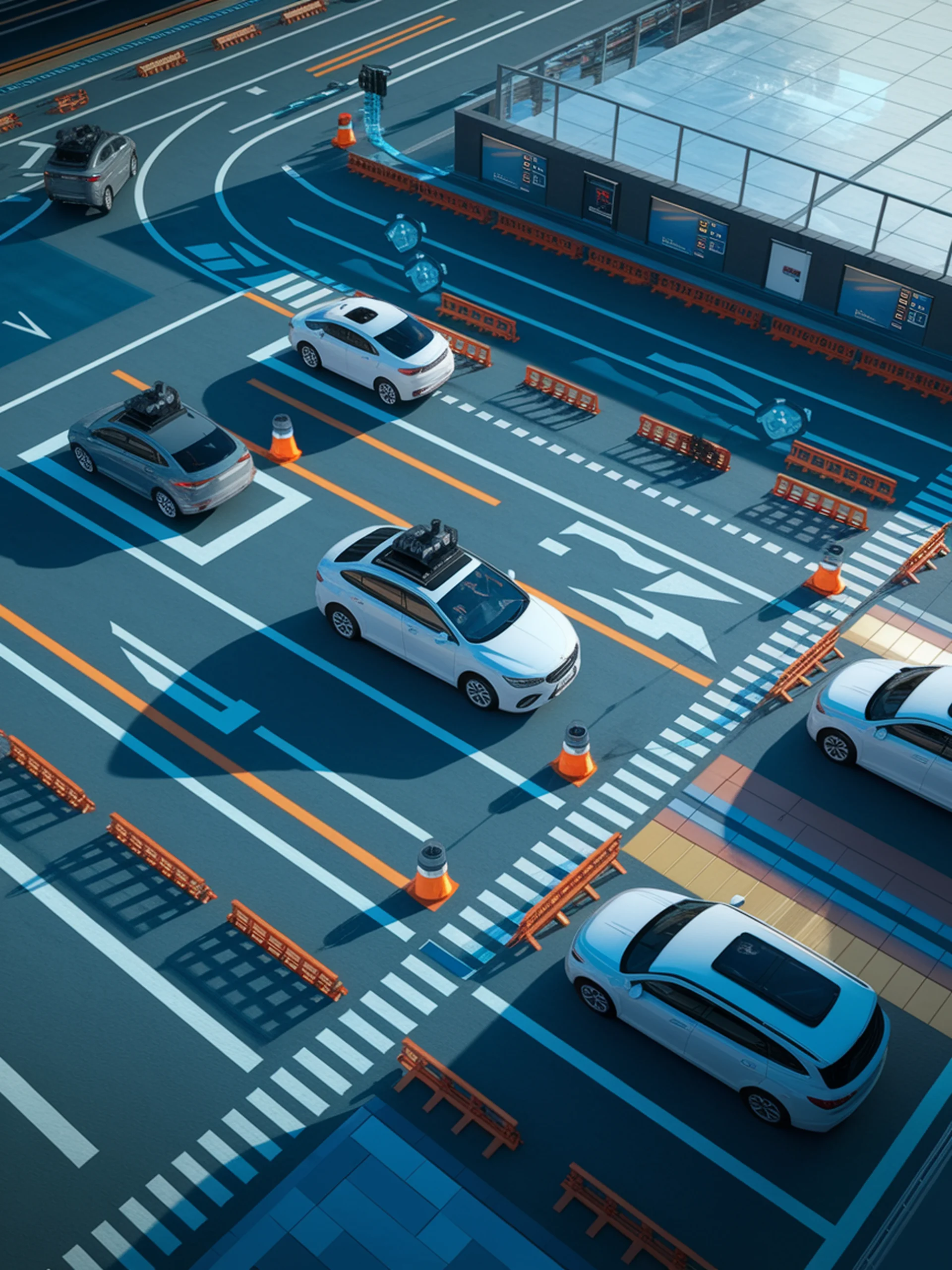
Enhancing Autonomous Vehicle Safety Testing
LLMs for Smarter Adversarial Scenario Generation
This research introduces LLM-attacker, a novel approach that leverages large language models to intelligently generate challenging test scenarios for autonomous driving systems.
- Uses LLMs to identify potential adversarial participants in traffic scenarios
- Implements a closed-loop framework that dynamically adapts testing based on vehicle responses
- Demonstrates superior effectiveness in generating safety-critical events compared to existing methods
- Shows practical applications for improving robustness of autonomous driving systems
This innovation matters because it addresses a critical engineering challenge: creating realistic yet challenging scenarios to test autonomous vehicles before real-world deployment, potentially saving lives by identifying safety vulnerabilities earlier in development.