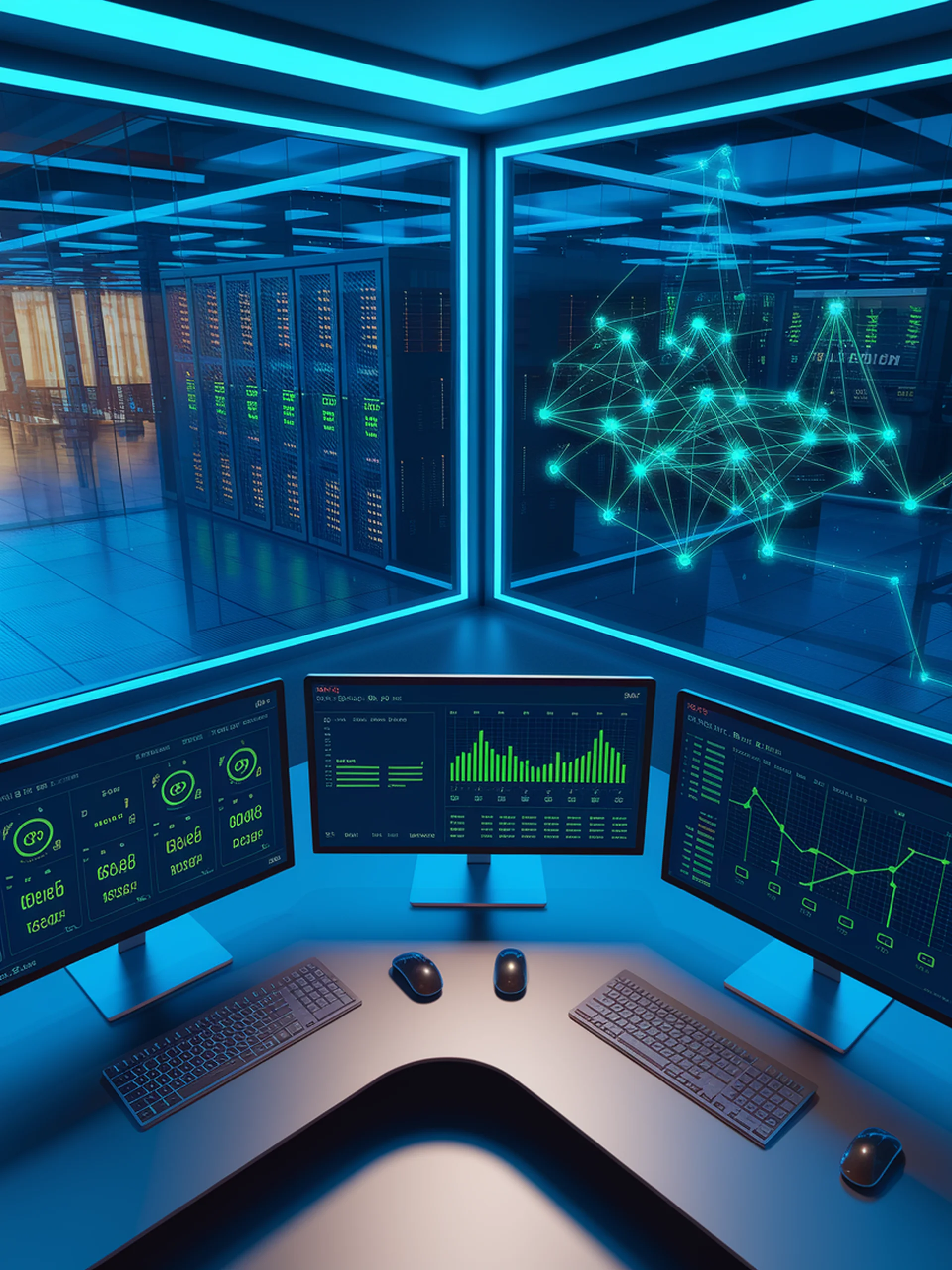
Self-Improving AI for Energy Systems
How LLMs can adaptively generate optimal control policies for microgrids
This research introduces a hierarchical framework where LLMs generate and continuously refine executable code for energy system optimization, rather than making direct decisions.
- Achieves up to 15% cost savings in microgrid scenarios through iterative improvement
- Employs a meta-policy to guide task generation and a base policy for operational actions
- Demonstrates how LLMs can adaptively create specialized algorithms for specific energy contexts
- Provides a blueprint for self-improving AI systems in critical infrastructure
This approach represents a significant advancement for engineering applications by enabling more efficient, reliable, and adaptive energy management systems that continuously improve their performance without constant human intervention.
Towards Adaptive Self-Improvement for Smarter Energy Systems