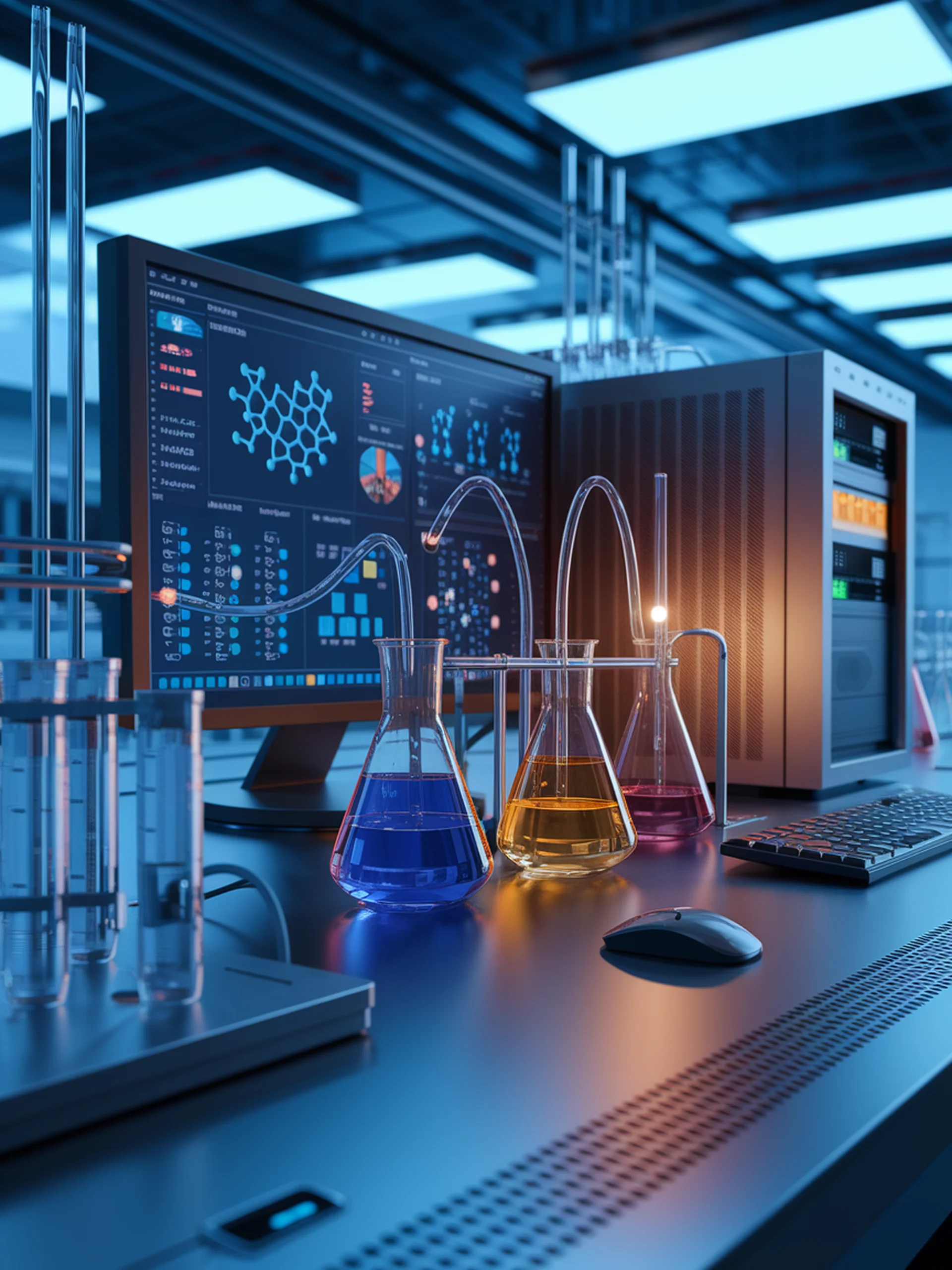
CoT Reasoning in Chemical Engineering
Enhancing predictions from limited experimental data
This research demonstrates how Chain-of-Thought (CoT) reasoning can transform chemical engineering data analysis with just 30 experimental data points.
- Integrates traditional surrogate models (Gaussian processes, random forests) with powerful LLMs like DeepSeek-R1
- Creates a hierarchical reasoning approach that overcomes limitations of both traditional methods and standard LLMs
- Enables more accurate predictions and insights from sparse experimental data
- Provides a locally-deployable solution addressing both computational efficiency and data privacy concerns
This innovation matters for engineering by offering a practical, efficient approach to extract maximum value from limited experimental datasets while maintaining data security.