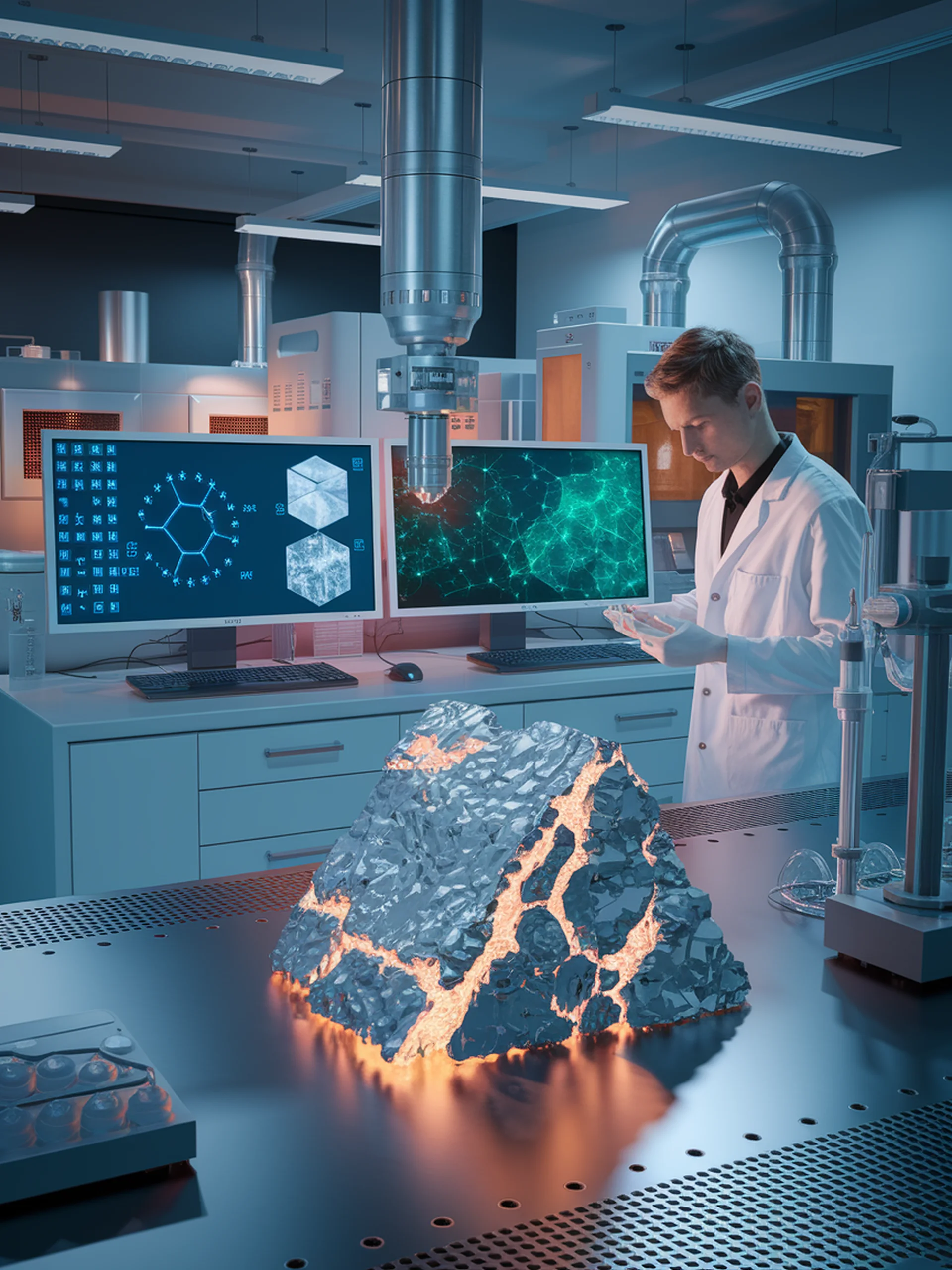
AI-Powered Materials Discovery
Integrating LLMs with Scientific Data for Advanced Alloy Development
This research introduces a novel framework that combines computational data with domain knowledge extracted by LLMs to accelerate high-entropy alloy discovery.
- Leverages large language models to distill knowledge from scientific literature
- Uses evidence theory to systematically fuse multiple knowledge sources
- Prioritizes promising material compositions in a vast design space
- Demonstrates superior performance compared to single-knowledge approaches
This approach significantly enhances materials engineering by reducing experimental costs and time while improving prediction accuracy for new alloy development, demonstrating a practical application of AI in advanced materials research.
Synergistic Fusion of Multi-Source Knowledge via Evidence Theory for High-Entropy Alloy Discovery