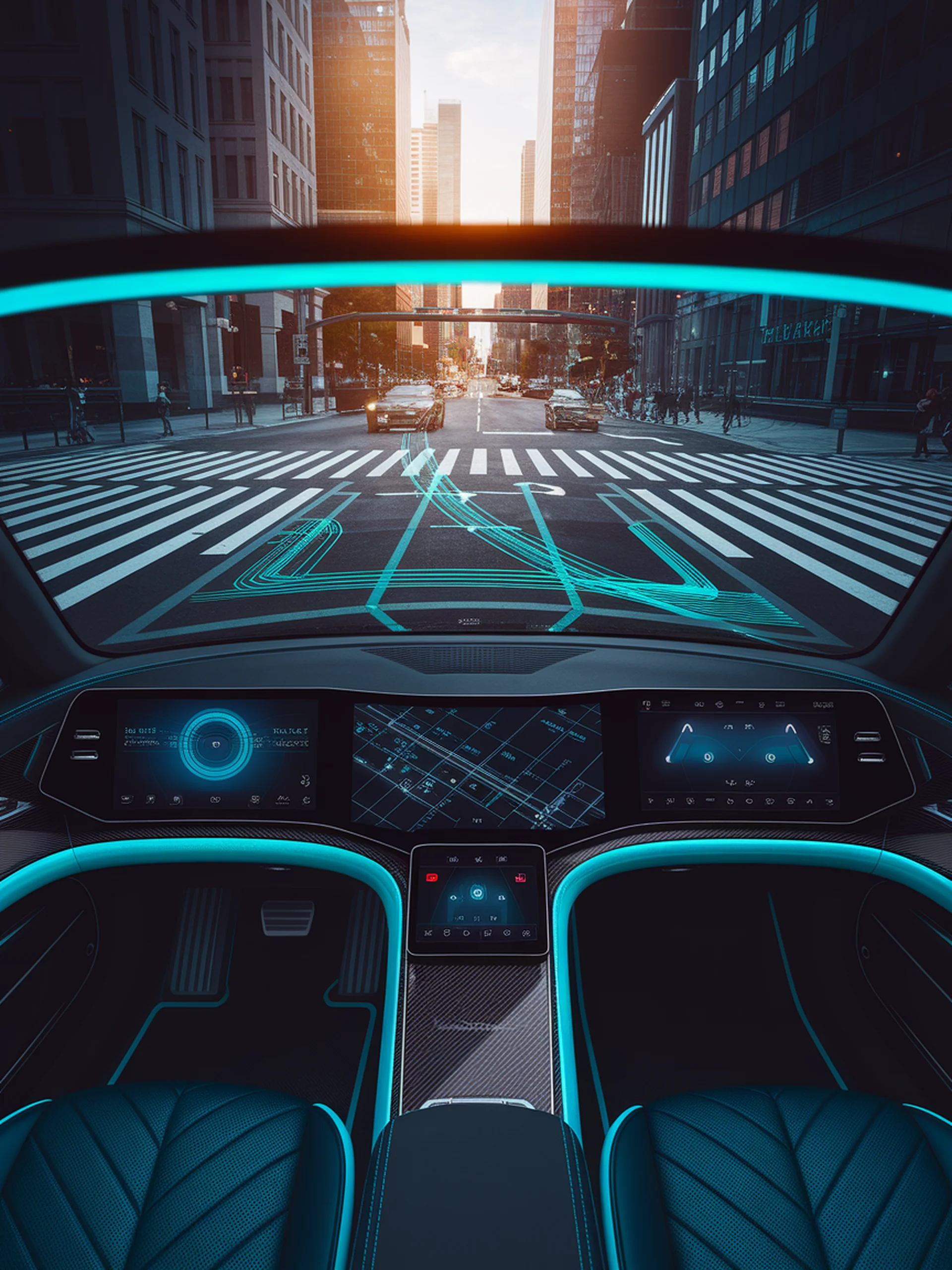
Unlocking Autonomous Driving's Potential
Leveraging Unlabeled Data to Enhance Language-Driven Vehicle Control
This research introduces a semi-supervised learning approach that reduces dependency on costly labeled data for vision-based language models in autonomous driving.
- Employs template-based prompts to extract valuable scene information from unlabeled driving data
- Significantly reduces annotation costs while maintaining or improving model performance
- Creates a more scalable approach to autonomous vehicle training
- Demonstrates how natural language understanding can enhance driving intelligence
For engineering teams, this represents a breakthrough in resource efficiency for autonomous systems development, potentially accelerating deployment timelines while maintaining safety standards.
Unlock the Power of Unlabeled Data in Language Driving Model