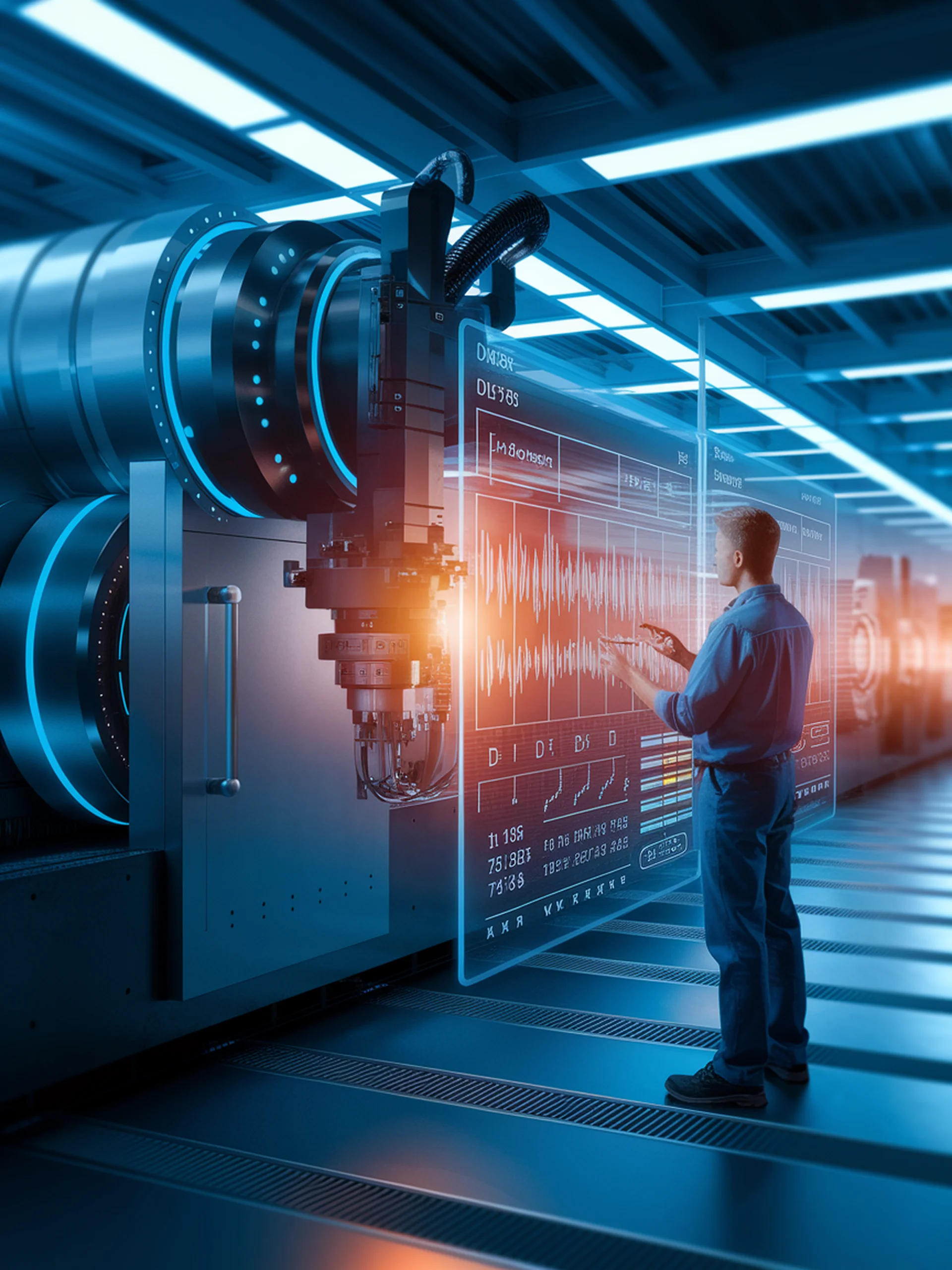
Revolutionizing Industrial Maintenance with AI
Using Causal Inference & LLMs to Predict Machine Lifespan
A novel framework that dramatically improves Remaining Useful Life (RUL) predictions for industrial machinery through causal analysis and transfer learning.
- Introduces the Causal-Informed Data Pruning Framework (CIDPF) that identifies key causal relationships in sensor data
- Leverages Gaussian Mixture Models to screen for anomalies in high-dimensional data
- Employs transfer learning with LLMs to improve prediction accuracy across different machine types
- Delivers more reliable maintenance scheduling and reduced downtime in factory settings
This approach addresses a critical engineering challenge by extracting meaningful signals from complex sensor data, enabling more precise predictive maintenance and extending equipment lifespan.