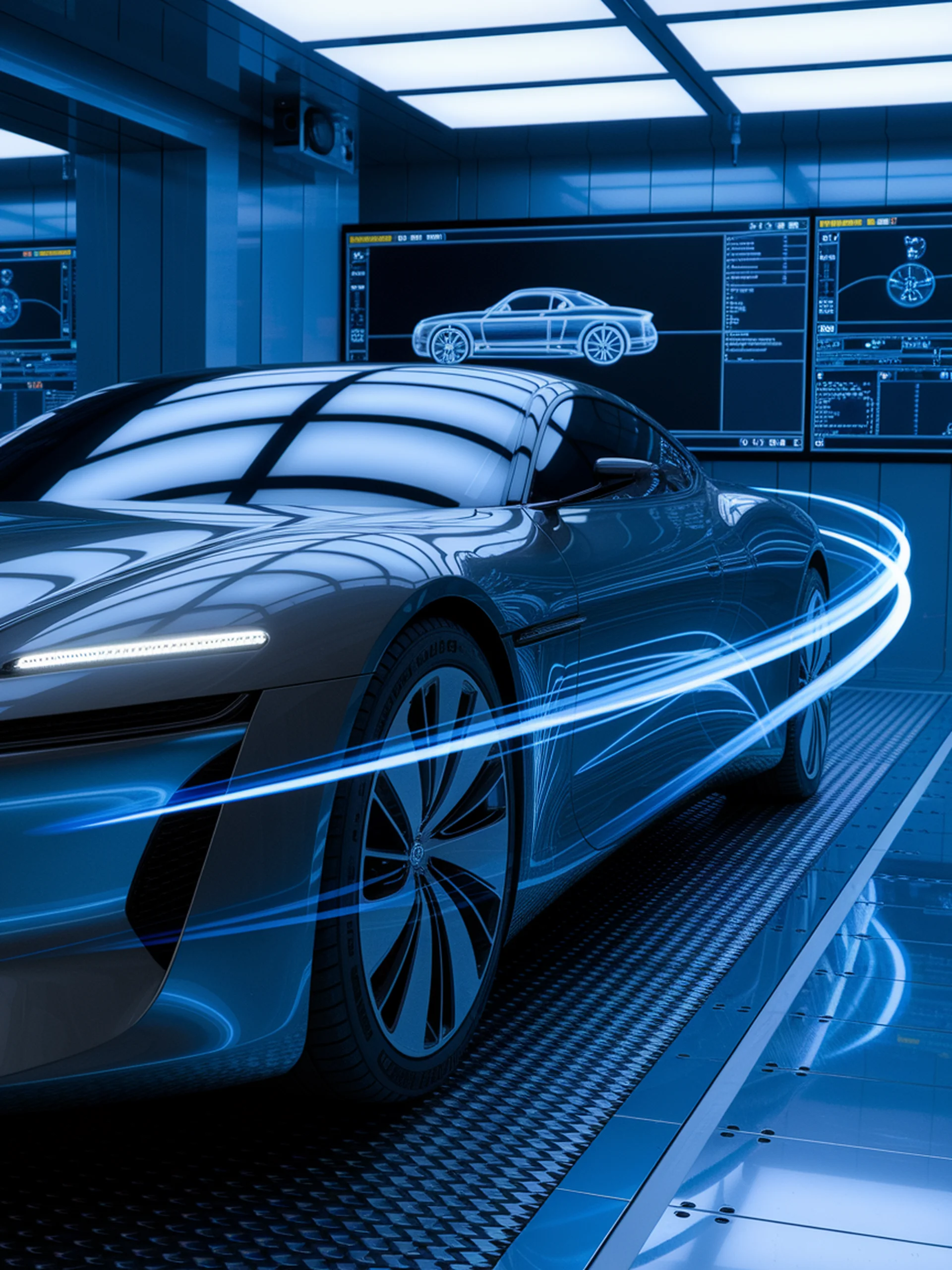
AI-Powered Aerodynamic Prediction
Transformers Revolutionizing Vehicle Drag Coefficient Calculations
This research introduces DrivAer Transformer, a novel approach that combines transformer architecture with 3D vehicle models to predict aerodynamic drag coefficients with high precision and remarkable speed.
- Achieves superior accuracy compared to traditional CFD simulations while dramatically reducing computation time
- Leverages the DrivAerNet++ dataset to overcome previous limitations in training data for complex 3D vehicle models
- Demonstrates how deep learning can effectively process complex geometric data for engineering applications
- Offers practical value for automotive design by accelerating the aerodynamic optimization process
This innovation significantly impacts engineering workflows by allowing faster iteration in vehicle design while maintaining high predictive accuracy, potentially transforming how aerodynamic optimization is integrated into production pipelines.