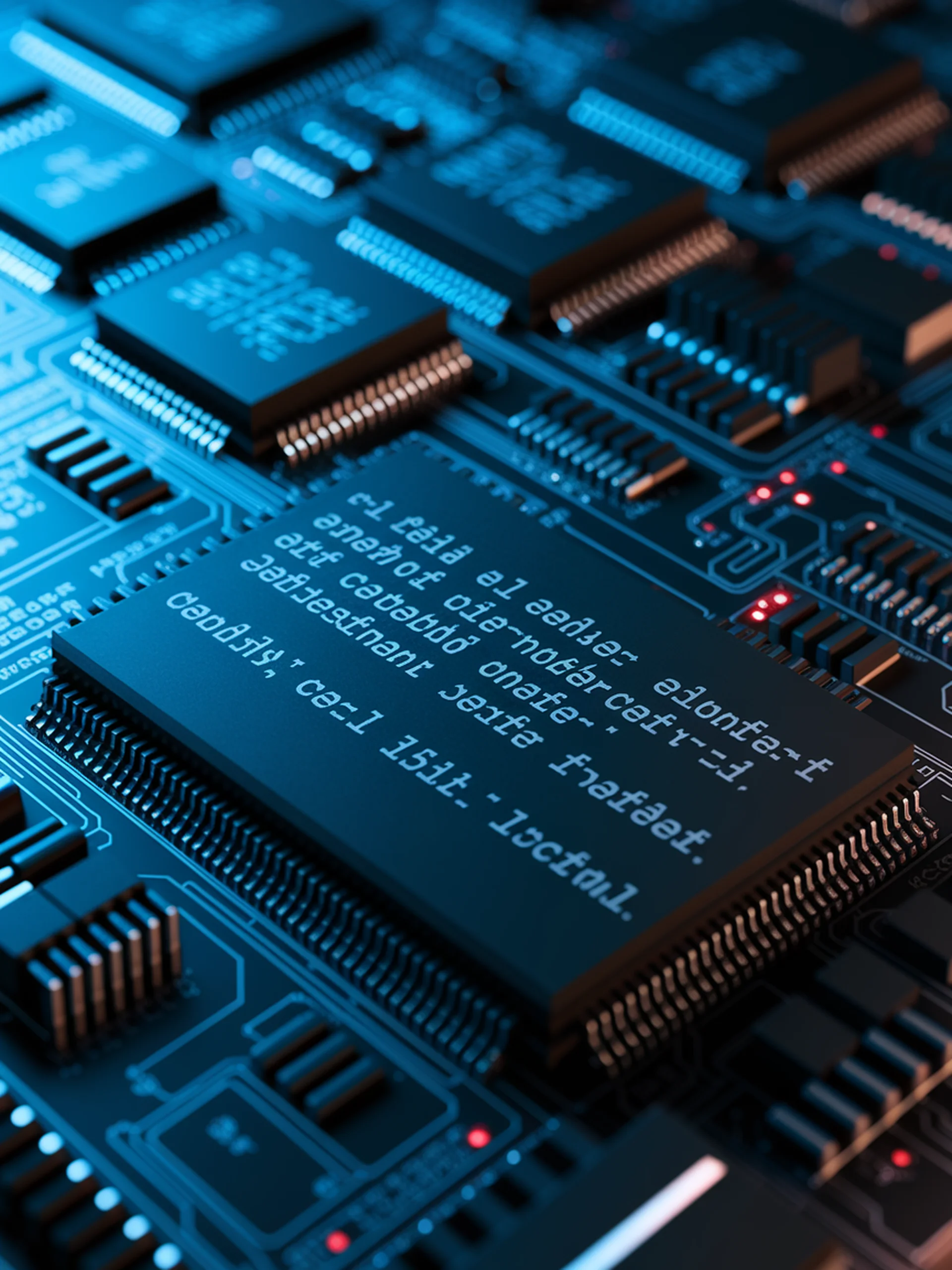
Data Contamination in AI Hardware Design
Evaluating the reliability of LLM-generated Verilog code
This research provides a systematic framework for evaluating data contamination in LLM-generated hardware description code, specifically Verilog.
- Developed VeriContaminated, the first benchmark to assess data contamination in hardware coding
- Analyzed multiple popular LLMs including GPT-4, Claude, and Gemini
- Found evidence suggesting some LLMs may be contaminated with Verilog test cases
- Proposed methodologies to detect and mitigate contamination risks
For engineering teams, this work highlights critical reliability considerations when adopting LLMs for hardware design workflows and provides practical approaches for validating AI-generated hardware code.
VeriContaminated: Assessing LLM-Driven Verilog Coding for Data Contamination