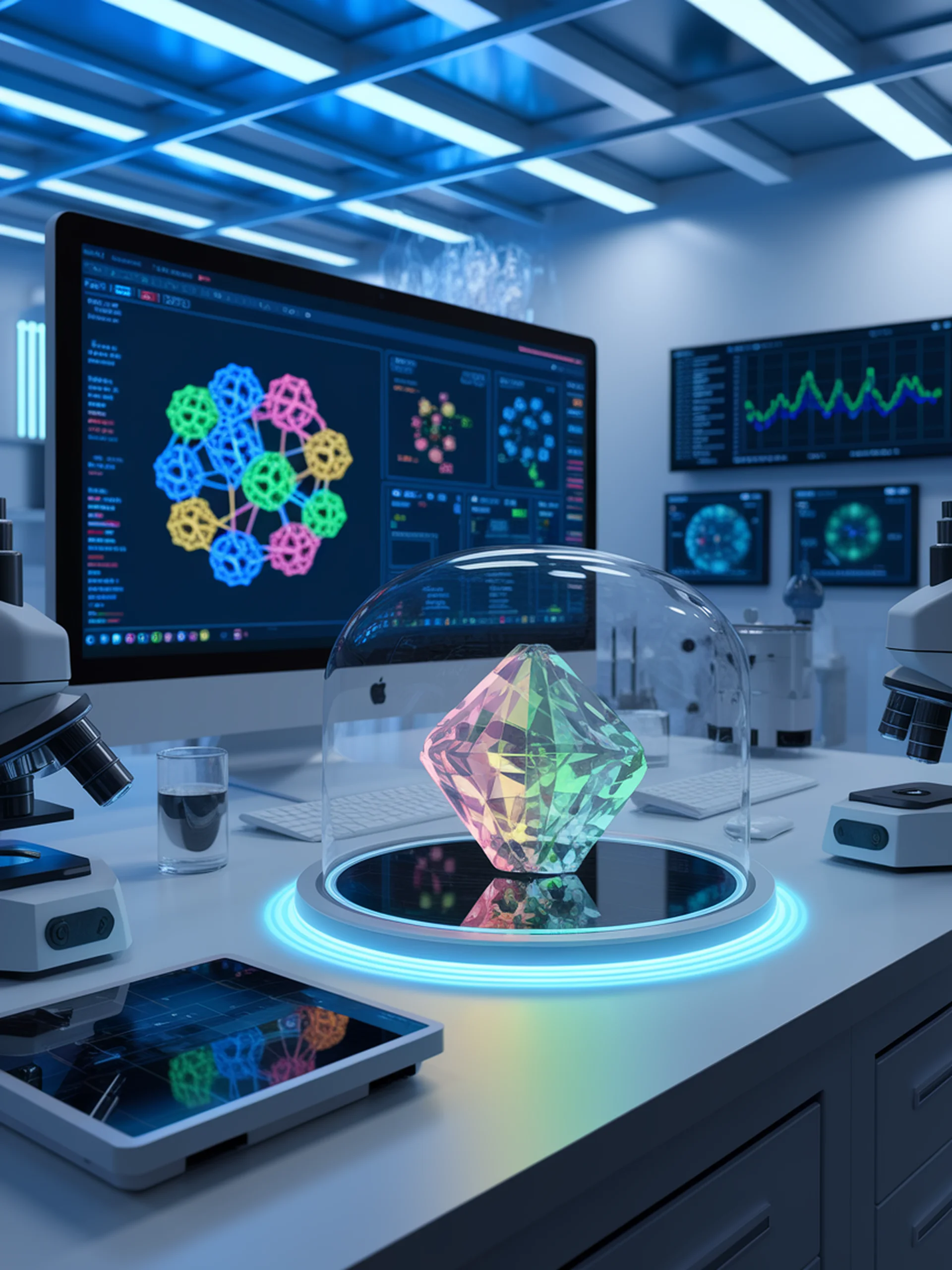
Reinforcement Learning Transforms Materials Design
AI-powered discovery of stable, high-performance materials
CrystalFormer-RL applies reinforcement learning to fine-tune transformer models for generating optimized crystal structures with desired properties.
- Uses reward signals like energy stability and property figures of merit
- Combines generative transformers with discriminative prediction models
- Demonstrates enhanced material stability and target property optimization
- Enables efficient exploration of vast chemical design spaces
This research transforms engineering by accelerating the discovery of novel materials with specific properties, potentially revolutionizing fields from electronics to renewable energy without costly trial-and-error experiments.
CrystalFormer-RL: Reinforcement Fine-Tuning for Materials Design