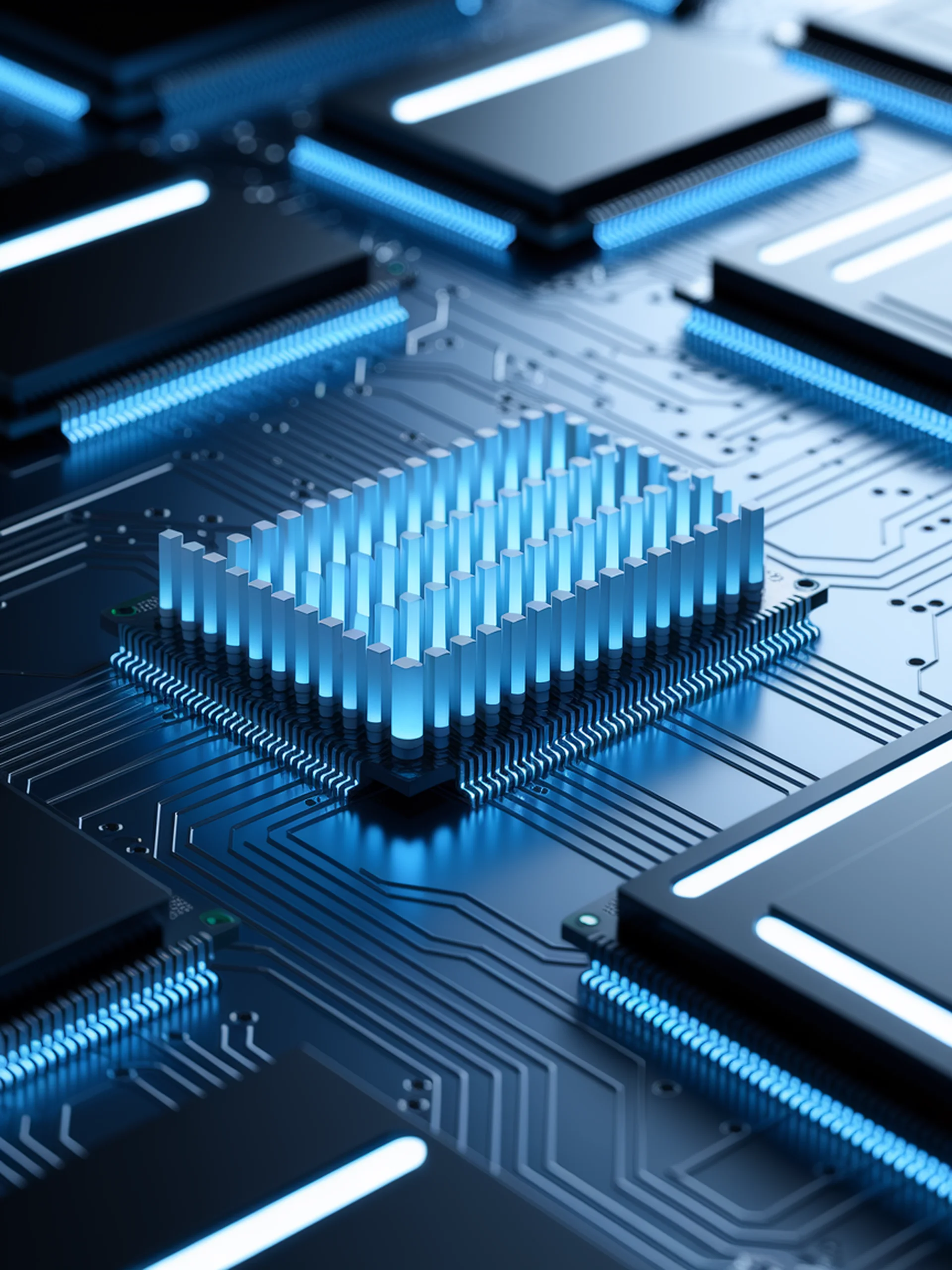
Smarter LLM Compression
Isolating Outliers for Efficient Low-bit Quantization
This research introduces novel techniques to significantly reduce LLM memory requirements while maintaining performance, especially critical for mobile and resource-constrained environments.
- Per-IC Quantization: Separates and processes input channels differently to handle activation outliers
- AdaDim: Adaptively determines optimal channel dimensions for quantization
- Practical Results: Achieves effective sub-4-bit quantization with minimal quality loss
- Memory Efficiency: Reduces inference memory footprint substantially compared to standard methods
This engineering breakthrough enables deploying powerful language models on devices with limited resources, opening new possibilities for on-device AI applications without sacrificing quality.