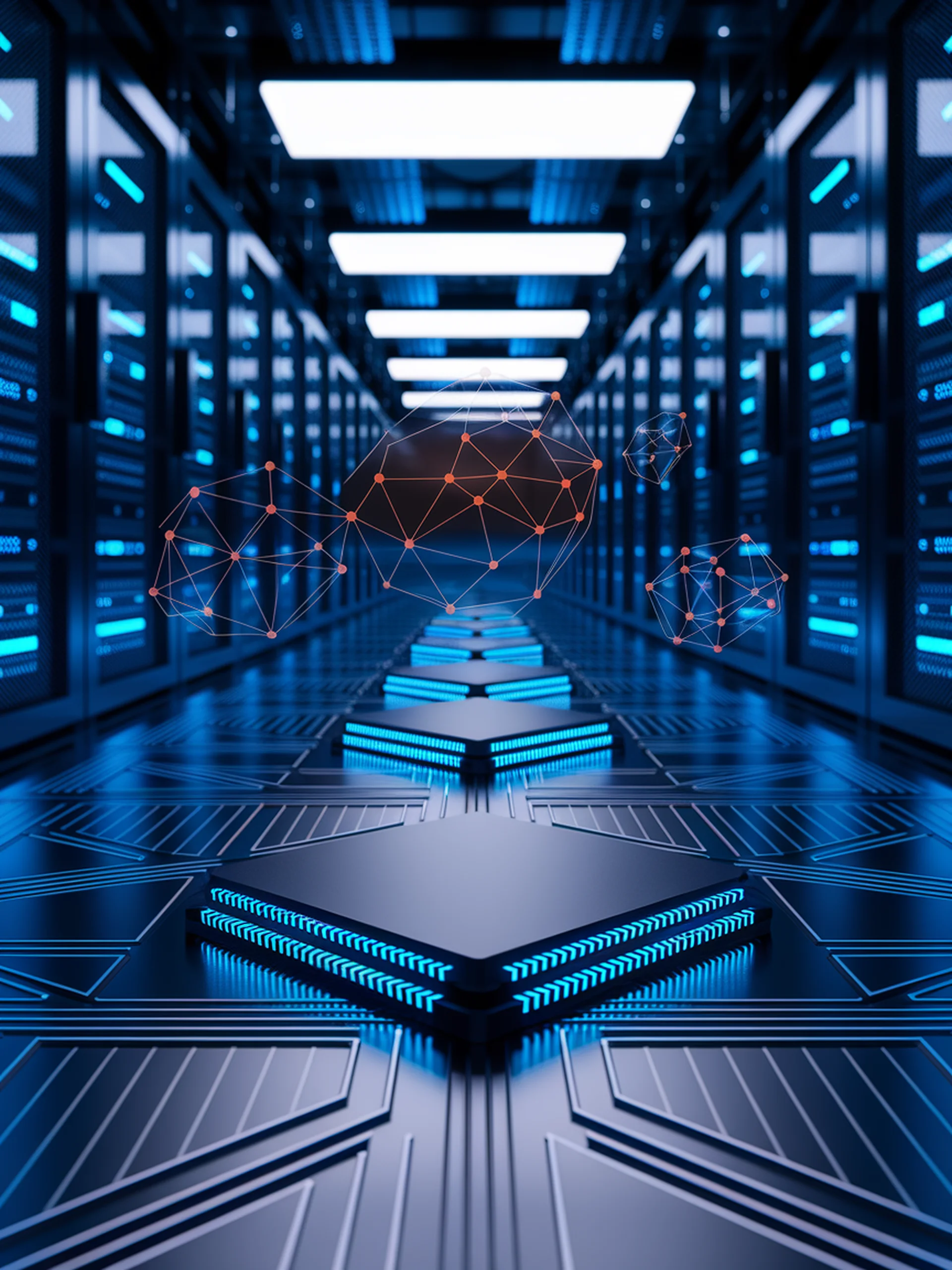
Smarter LLM Compression with CBQ
Leveraging cross-block dependencies for efficient model quantization
CBQ (Cross-Block Quantization) introduces a novel approach to compress Large Language Models while maintaining performance, even at low-bit settings.
- Addresses limitations of current post-training quantization methods by considering dependencies between blocks, not just within them
- Employs homologous reconstruction to preserve model capabilities during compression
- Achieves superior performance compared to existing quantization techniques for LLMs
- Enables more efficient deployment of large models on resource-constrained devices
This engineering advancement matters because it dramatically reduces computational and memory requirements for LLMs without sacrificing quality, making advanced AI more accessible and affordable across platforms.