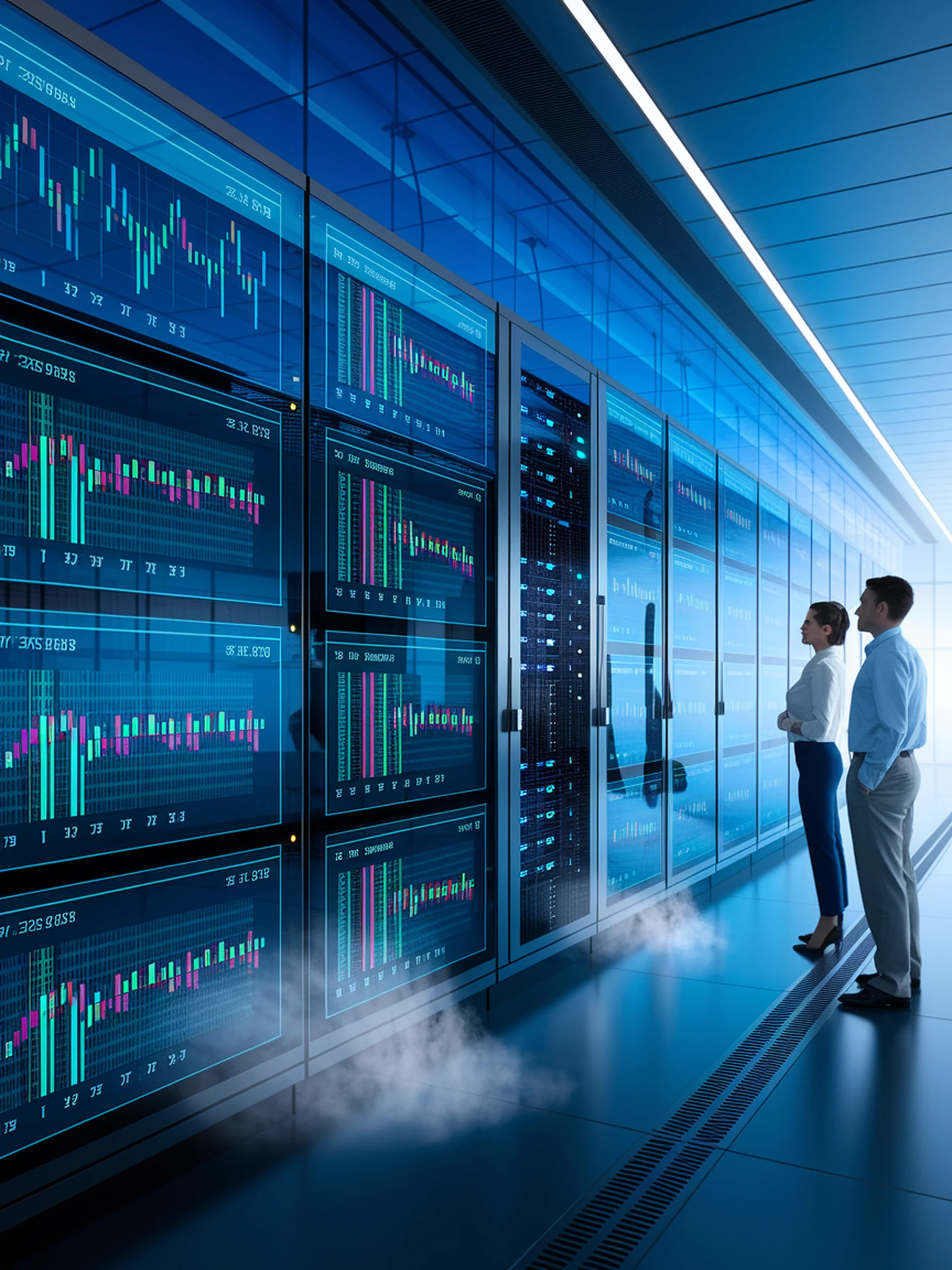
Optimizing LLM Inference Speed
Unlocking Efficient Speculative Decoding Techniques
Speculative decoding significantly accelerates Large Language Model inference by using smaller draft models to generate tokens that are later verified by the target LLM.
Key findings:
- The draft model choice critically impacts performance gains
- Systematic benchmarking across 350+ experiments with LLaMA-65B and OPT-66B
- Reveals optimal draft model selection strategies for maximum throughput
- Engineering trade-offs between model size, speed, and verification accuracy
This research matters for engineering teams seeking to deploy efficient LLM systems at scale, offering practical guidelines to balance inference speed and computational resource usage.