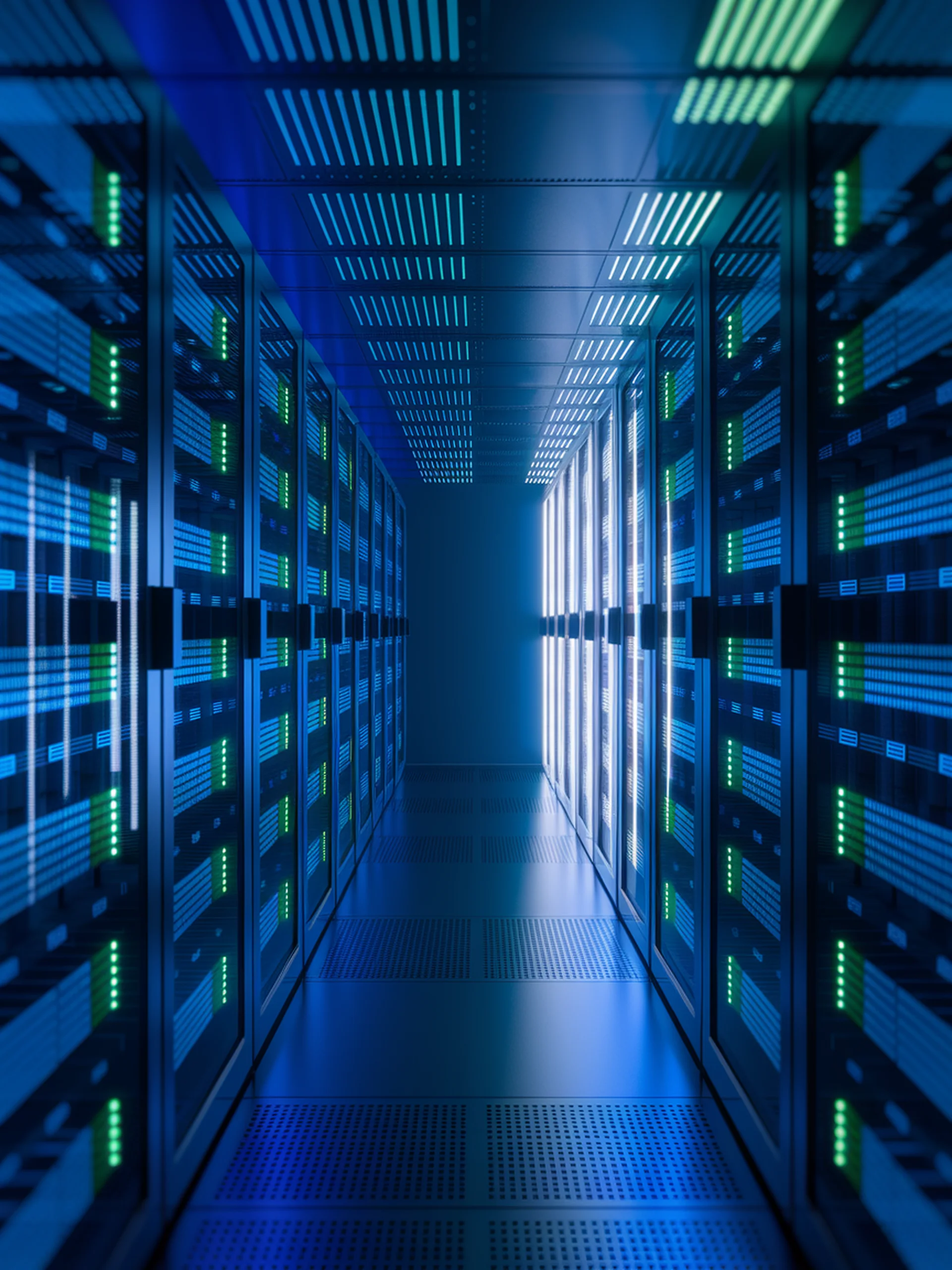
Accelerating LLM Inference with Plato
Efficient parallel decoding without compromising quality
Plato introduces a novel co-designed approach that significantly improves LLM inference efficiency while maintaining high answer quality.
- Overcomes limitations of existing parallel decoding methods like Skeleton-of-Thought that treat semantically linked sub-problems as independent
- Combines innovative algorithms with optimized system architecture for superior performance
- Achieves substantial computational and memory efficiency improvements without sacrificing response quality
- Addresses a critical bottleneck in practical LLM deployment at scale
This research matters for Engineering teams by providing a practical solution to one of the most pressing challenges in LLM deployment: balancing inference speed with output quality in resource-constrained environments.
Plato: Plan to Efficiently Decode for Large Language Model Inference