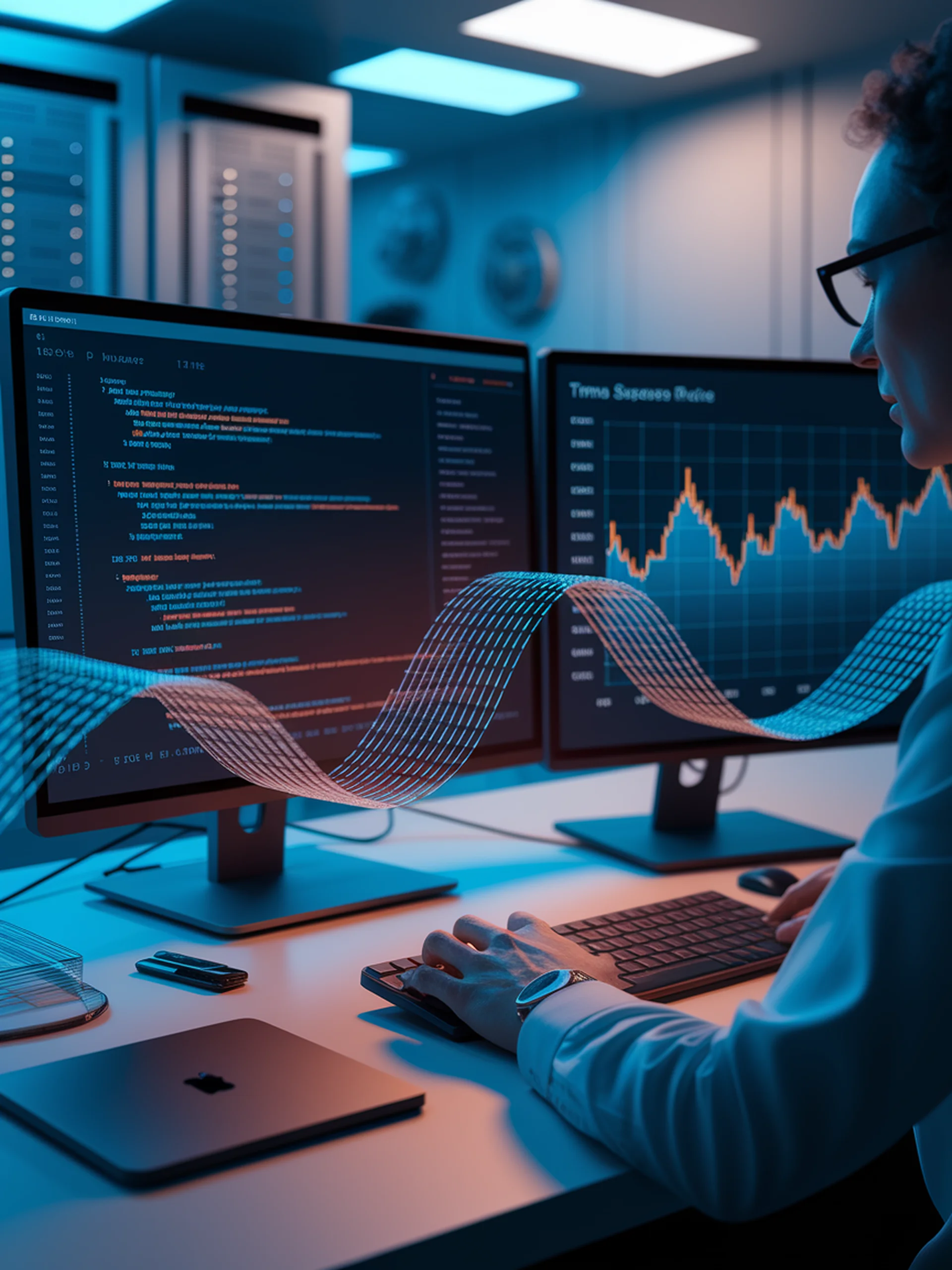
Bridging the Gap: LLMs for Time Series Forecasting
A novel cross-modal fine-tuning approach (CALF) that aligns language and numerical data distributions
CALF introduces a framework that effectively aligns LLMs with time series data, overcoming the distribution gap between textual and numerical inputs for improved forecasting accuracy.
Key Innovations:
- Addresses the distribution mismatch between language and time series data modalities
- Implements a cross-modal alignment technique that preserves both textual and numerical capabilities
- Demonstrates superior performance with limited temporal data compared to traditional forecasting methods
- Maintains LLM's reasoning abilities while adapting to numerical forecasting tasks
This research significantly advances engineering applications in predictive analytics, offering a pathway to leverage powerful language models for critical numerical forecasting challenges in manufacturing, energy, logistics, and other time-sensitive domains.
CALF: Aligning LLMs for Time Series Forecasting via Cross-modal Fine-Tuning