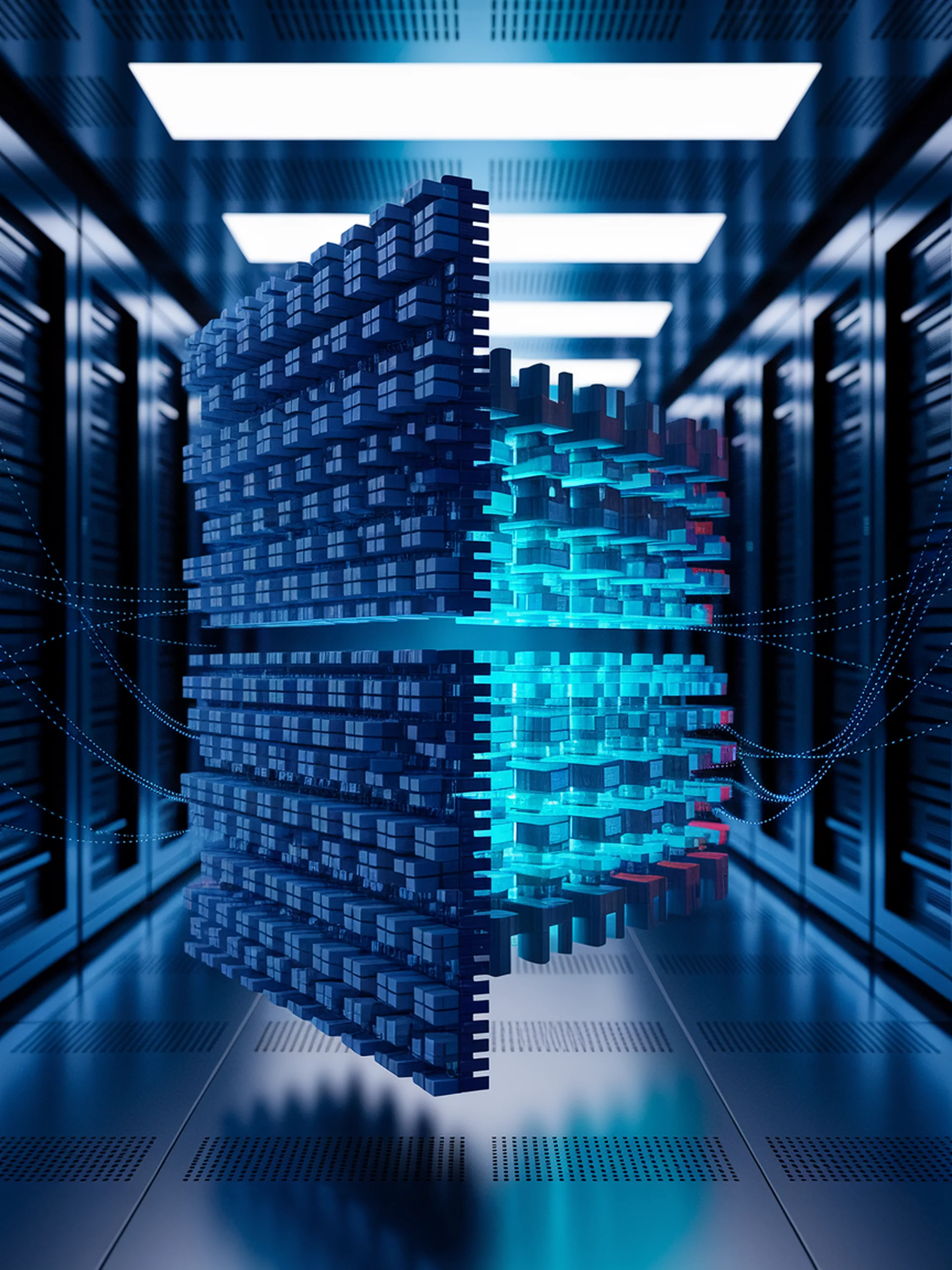
Smarter SVD for LLM Compression
Optimizing model size without sacrificing performance
This research introduces SVD-LLM, a novel approach to compress large language models through optimized Singular Value Decomposition techniques.
- Addresses key limitations in traditional SVD compression by selectively preserving important singular values
- Implements a post-truncation update strategy to recover performance after compression
- Achieves significantly better performance-to-size ratio than standard SVD methods
- Enables practical deployment of powerful language models on resource-constrained devices
For engineering teams, this breakthrough means more efficient AI deployments without the traditional performance tradeoffs, potentially reducing infrastructure costs while maintaining model capabilities.
SVD-LLM: Truncation-aware Singular Value Decomposition for Large Language Model Compression