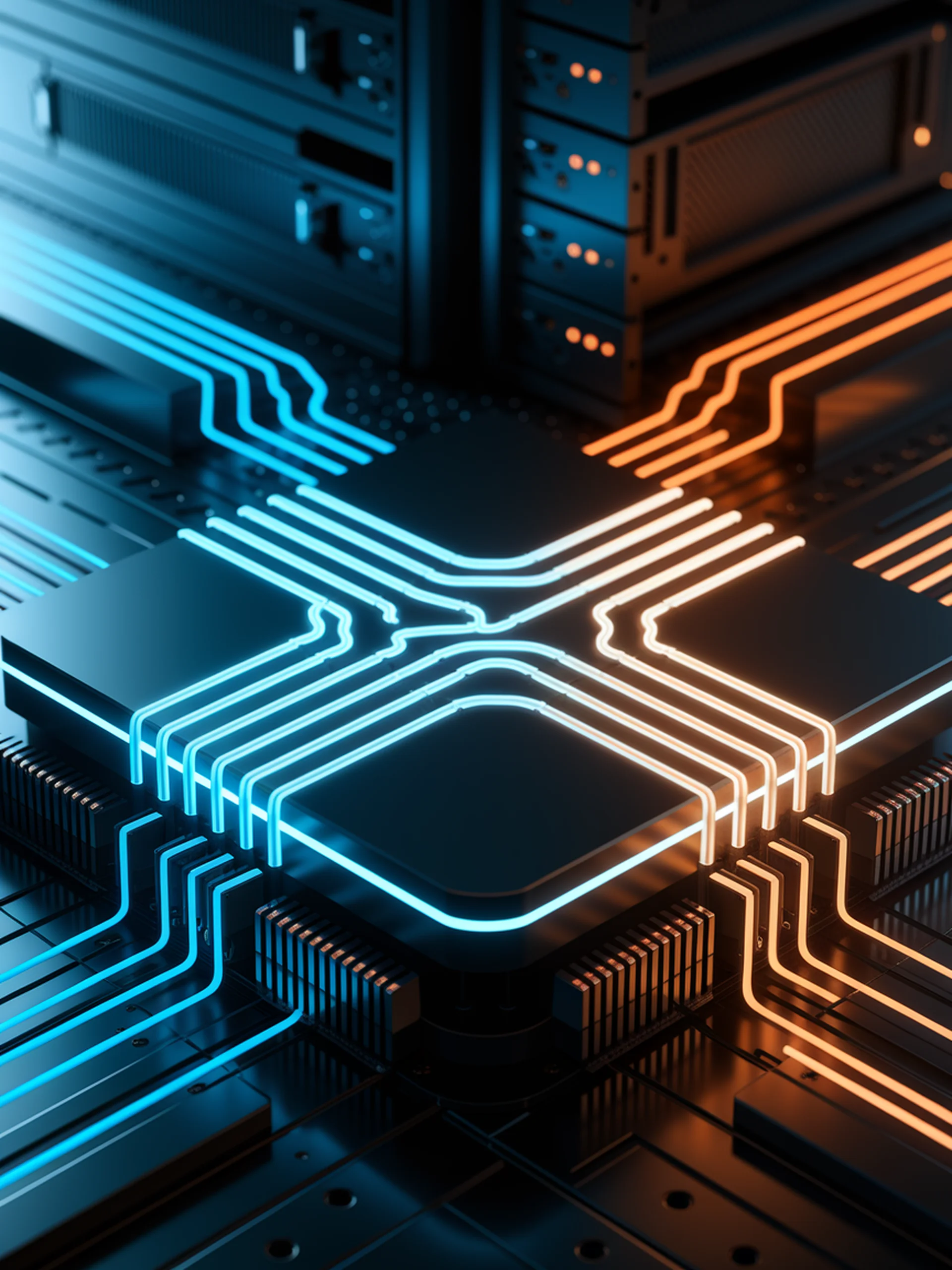
Accelerating Tree-structured LLM Inference
A novel approach to efficiently handle shared computations in complex LLM tasks
DeFT introduces a new technique for optimizing LLM inference when multiple generation paths share common token prefixes, significantly improving computational efficiency.
- Addresses inefficient memory access patterns in tree-structured LLM applications like few-shot prompting and multi-step reasoning
- Implements Flash Tree-attention to enable proper reuse of cached computations across shared prefixes
- Achieves substantial performance gains by optimizing GPU memory access and utilization
- Provides a practical solution for high-performance, tree-structured inference scenarios
This research matters for engineering teams deploying LLMs at scale, offering concrete methods to reduce computational overhead and improve throughput without sacrificing model quality.
DeFT: Decoding with Flash Tree-attention for Efficient Tree-structured LLM Inference