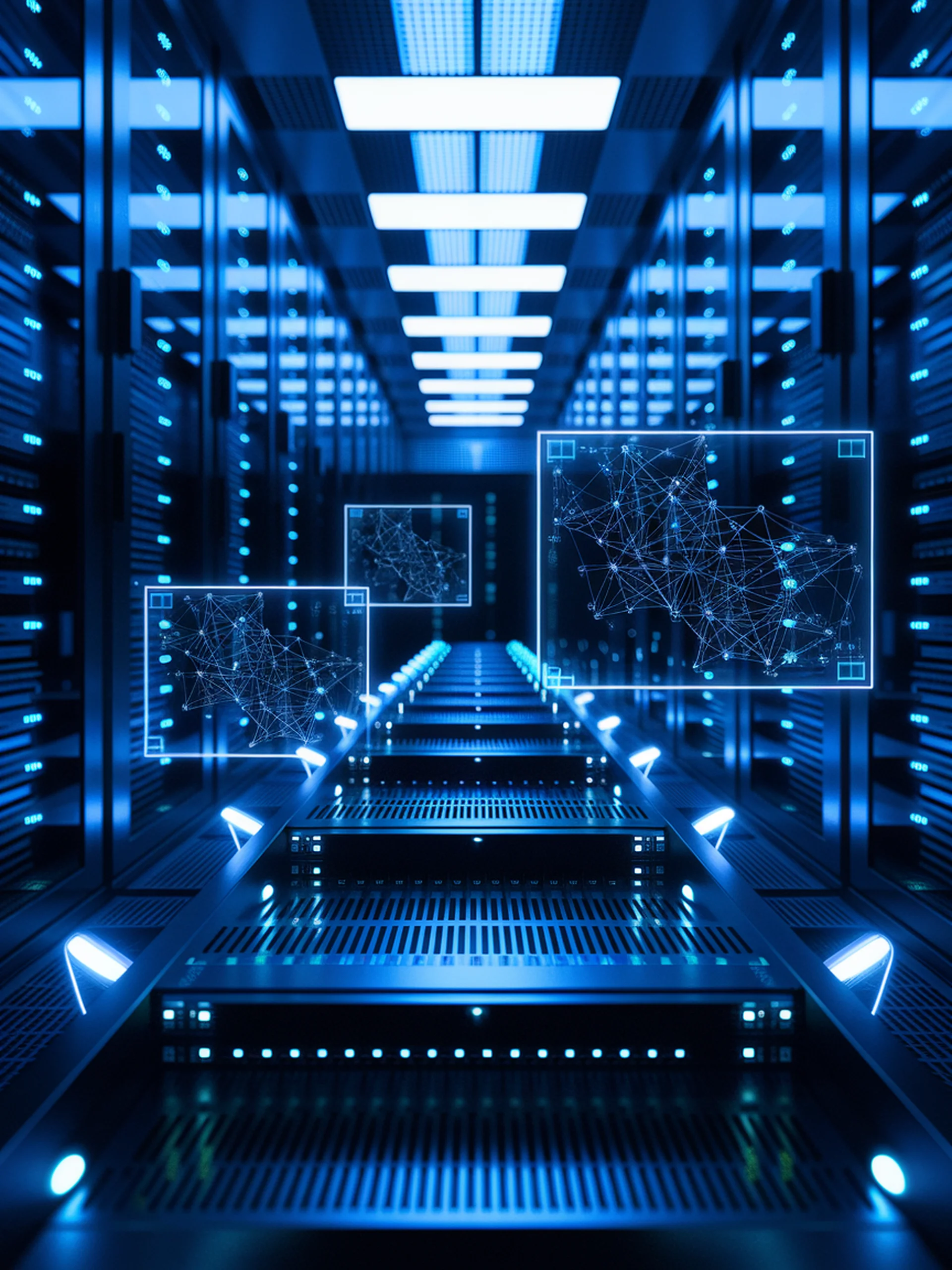
Unlocking Efficient Attention in LLMs
How Sparse Attention Achieves Sub-Quadratic Complexity
Sparse Attention techniques reduce computational complexity in large language models by selectively ignoring smaller attention matrix values while maintaining performance.
- Enables more efficient LLM deployment by reducing the quadratic complexity of standard attention
- Provides theoretical foundations for widely-used techniques like pruning KV cache
- Demonstrates practical approaches for maintaining quality while substantially reducing computational load
- Benefits engineering teams by making LLMs more deployable in resource-constrained environments
This research helps AI engineers develop more efficient models that require less computing power and memory while maintaining effectiveness.
How Sparse Attention Approximates Exact Attention? Your Attention is Naturally $n^C$-Sparse