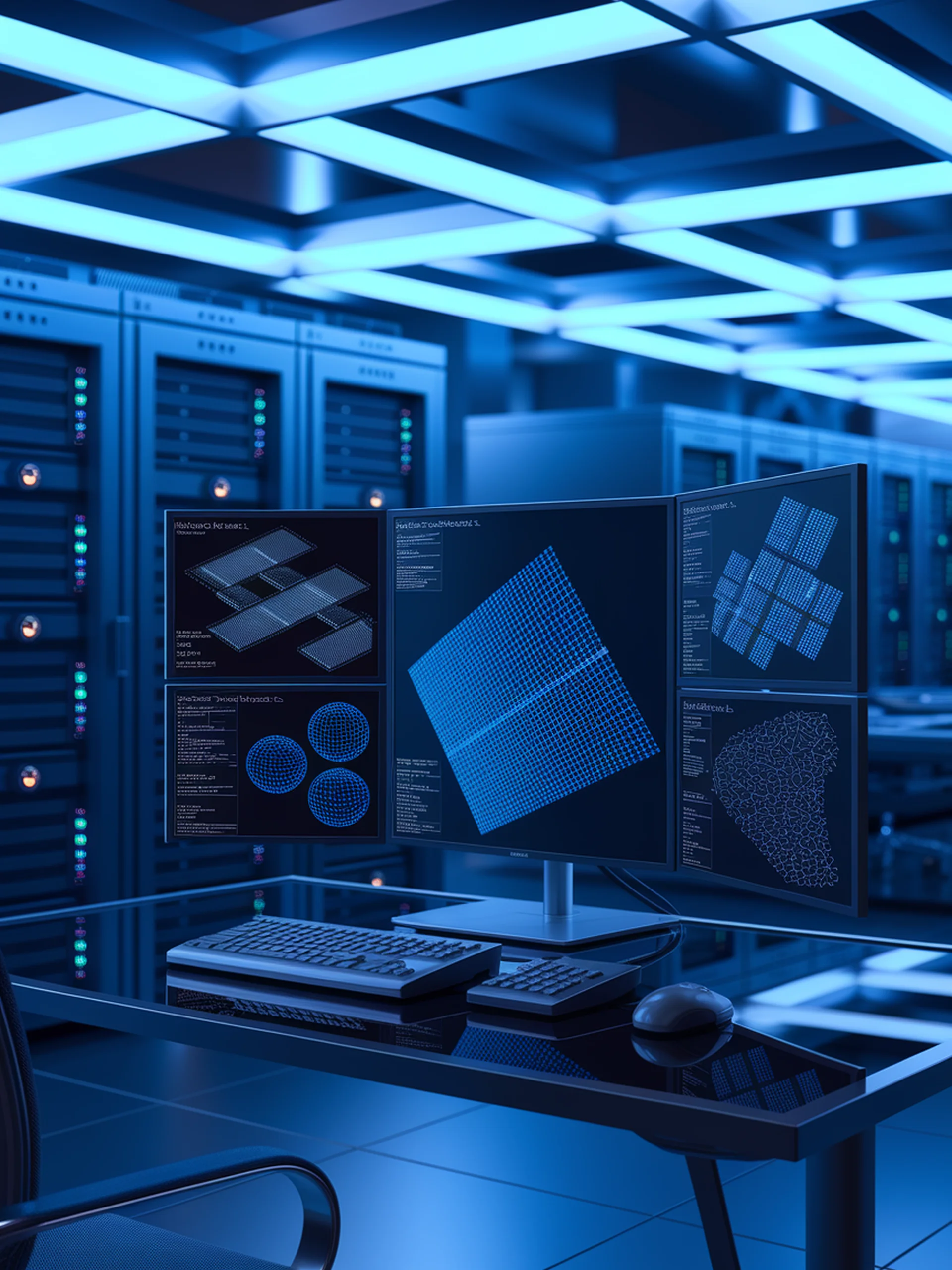
Smarter LLM Compression
Adaptive Low-Rank Compression Using Bayesian Optimization
This research introduces an adaptive feature-based low-rank compression technique for large language models that reduces computational burden while maintaining performance.
- Compresses LLMs by decomposing weight matrices into products of smaller low-rank matrices
- Uses Bayesian optimization to efficiently identify optimal compression configurations
- Achieves significant parameter reduction with minimal performance loss
- Addresses the critical challenge of balancing model scale and computational efficiency
This engineering innovation enables more efficient deployment of large language models across resource-constrained environments, making advanced AI more accessible and practical for business applications.
Adaptive Feature-based Low-Rank Compression of Large Language Models via Bayesian Optimization