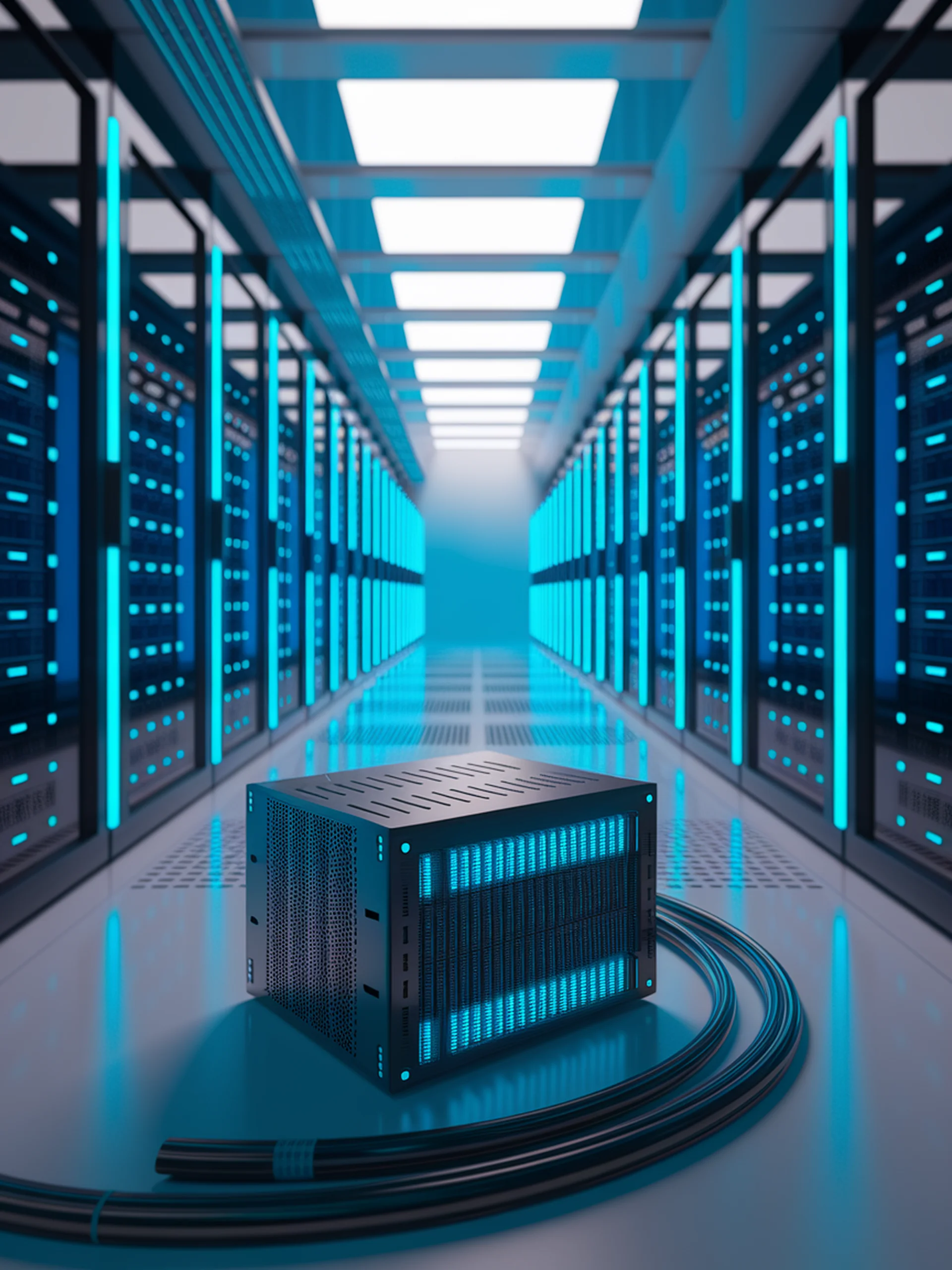
SpinQuant: Optimizing LLMs for Efficiency
Enhancing model quantization with learned rotations
SpinQuant introduces a novel approach to post-training quantization for Large Language Models that significantly reduces memory usage, latency, and power consumption while preserving performance.
- Tackles the critical outlier problem in quantization through intelligent rotation of activation/weight matrices
- Identifies optimal rotation parameterizations that maintain full-precision outputs
- Enables more efficient deployment of LLMs across resource-constrained environments
- Supports practical engineering applications by balancing model performance with computational efficiency
This research addresses a key engineering challenge in LLM deployment, making advanced AI capabilities more accessible and sustainable for real-world applications without quality degradation.