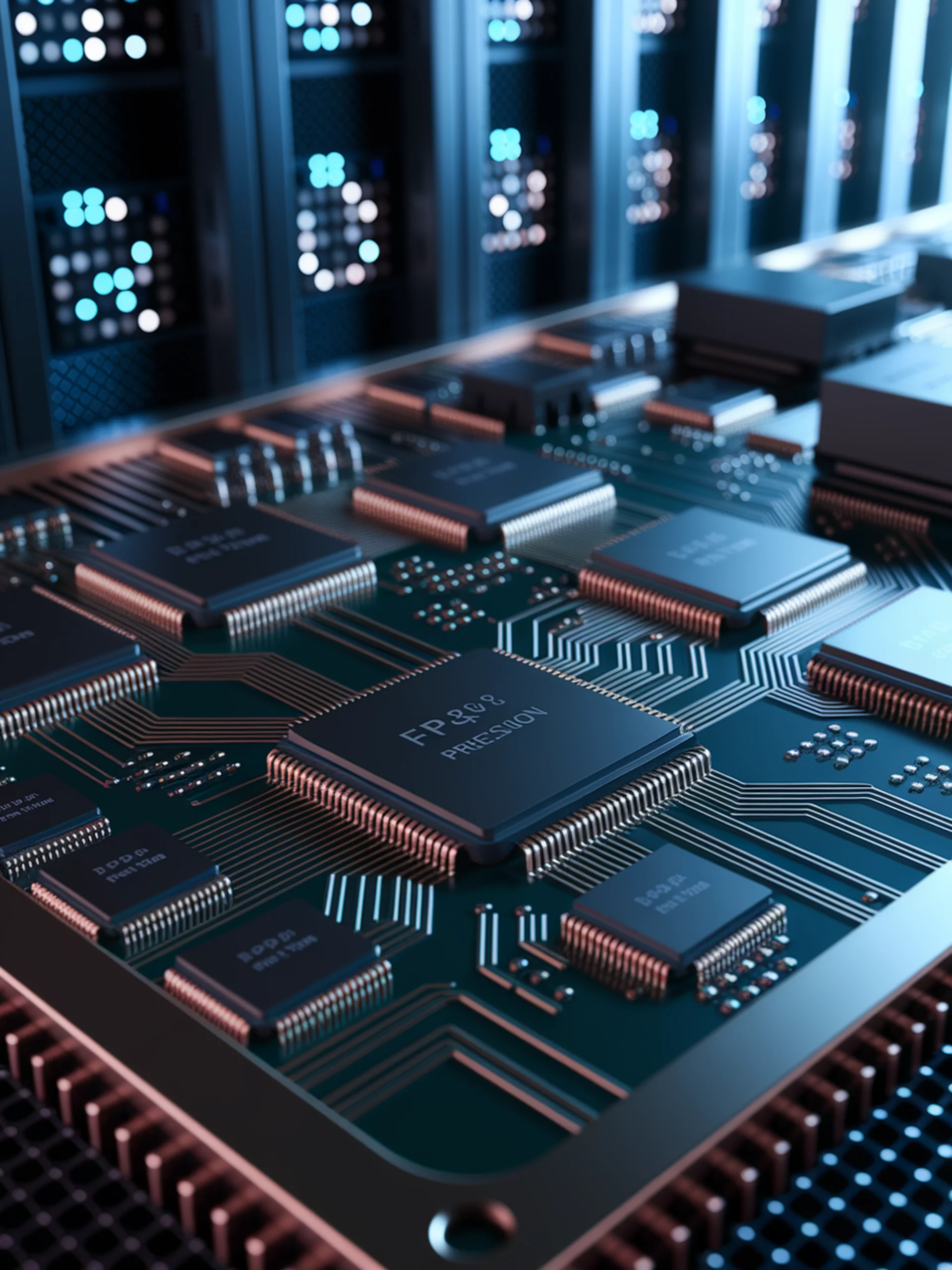
Optimizing LLM Training with FP8 Precision
Quantifying stability impacts of reduced-precision arithmetic
This research investigates how FP8 reduced-precision affects large language model training stability, helping organizations optimize computation without sacrificing model quality.
- FP8 precision can achieve comparable model quality to BF16 with proper stability considerations
- Researchers identified specific mathematical operations that cause instability in reduced-precision training
- The study provides practical guidelines for implementing FP8 training safely
- Potential for significant computational efficiency gains when deploying LLMs at scale
For engineering teams, this work offers a path to more cost-effective and energy-efficient LLM development while maintaining training stability—a critical advancement as model sizes continue to grow.
To FP8 and Back Again: Quantifying Reduced Precision Effects on LLM Training Stability