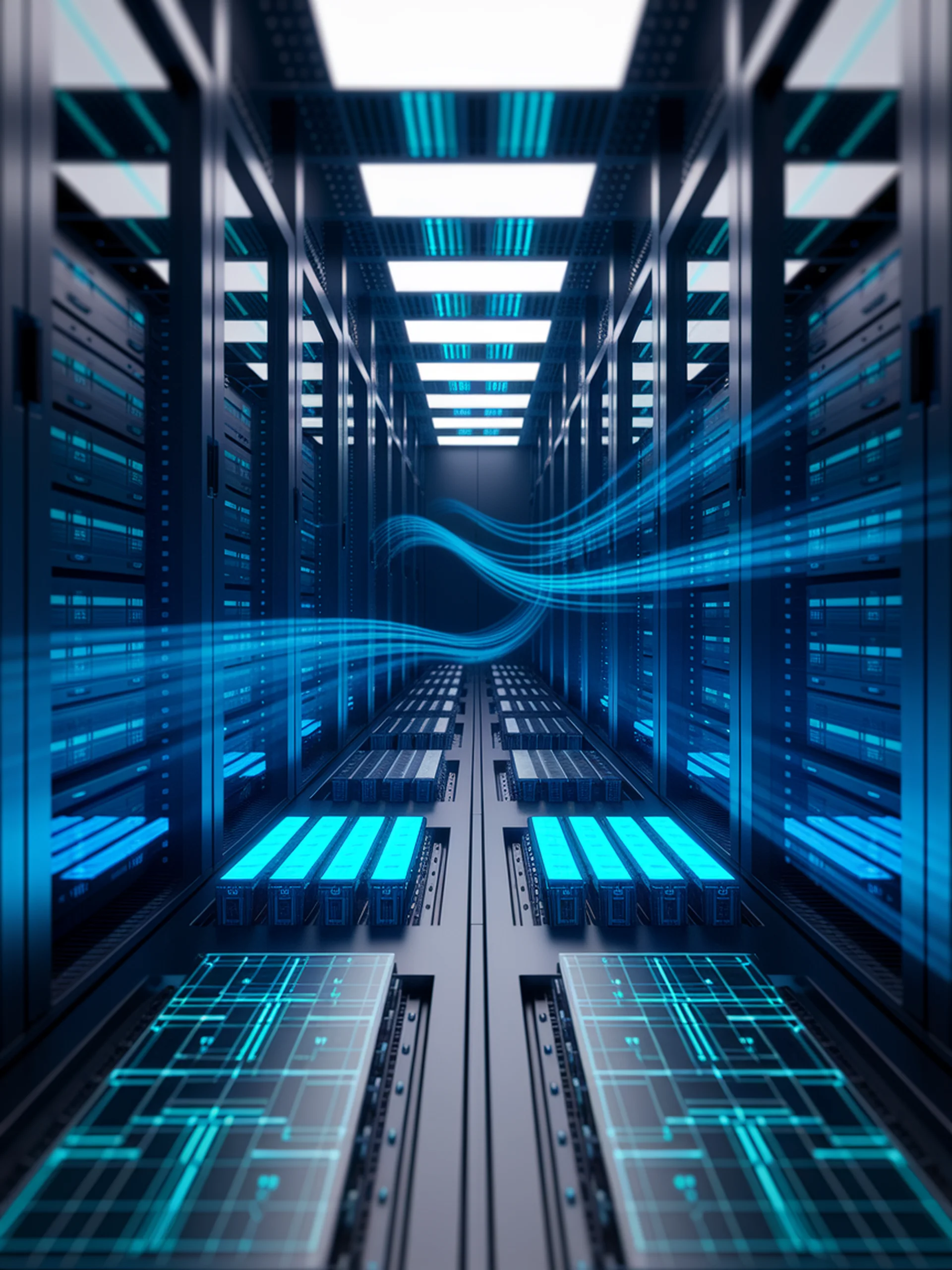
Smarter Memory Management for LLMs
D2O: A Dynamic Approach to Handling Long Contexts
D2O introduces a novel KV cache compression method that dynamically optimizes memory usage while preserving generation quality for large language models processing long context.
- Reduces memory demands by 67-83% while maintaining output quality
- Employs discriminative operations to intelligently determine which context to retain
- Achieves up to 2× speedup in inference without compromising effectiveness
- Compatible with existing LLM architectures without retraining
This engineering breakthrough enables processing of significantly longer sequences with existing hardware resources, making advanced LLM applications more practical and cost-effective for businesses.
D2O: Dynamic Discriminative Operations for Efficient Long-Context Inference of Large Language Models