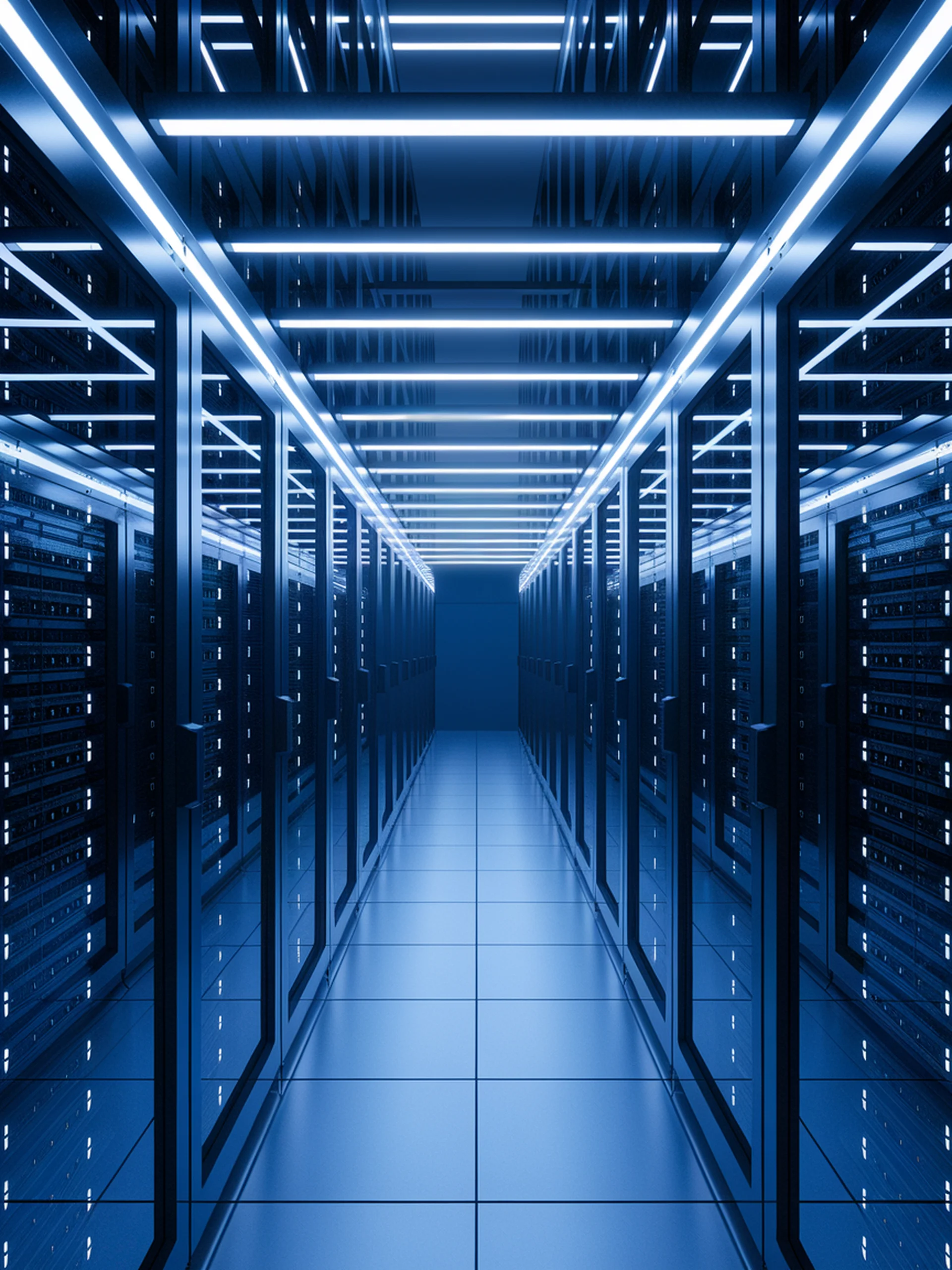
Extending LLM Context Windows Exponentially
A Training-Free Solution for Long-Sequence Processing
This research introduces a novel cascading Key-Value (KV) cache approach that exponentially extends the effective context window of large language models without additional training.
Key Innovations:
- Achieves 4-8x longer context windows without performance degradation
- Implements smart token preservation strategies instead of naive eviction
- Requires zero model retraining while maintaining inference quality
- Significantly improves performance on tasks requiring long-term memory
This engineering breakthrough enables practical deployment of LLMs for real-world applications requiring extensive context, such as complex document analysis, long-form content generation, and secure passkey retrieval, all while maintaining computational efficiency.
Training-Free Exponential Context Extension via Cascading KV Cache