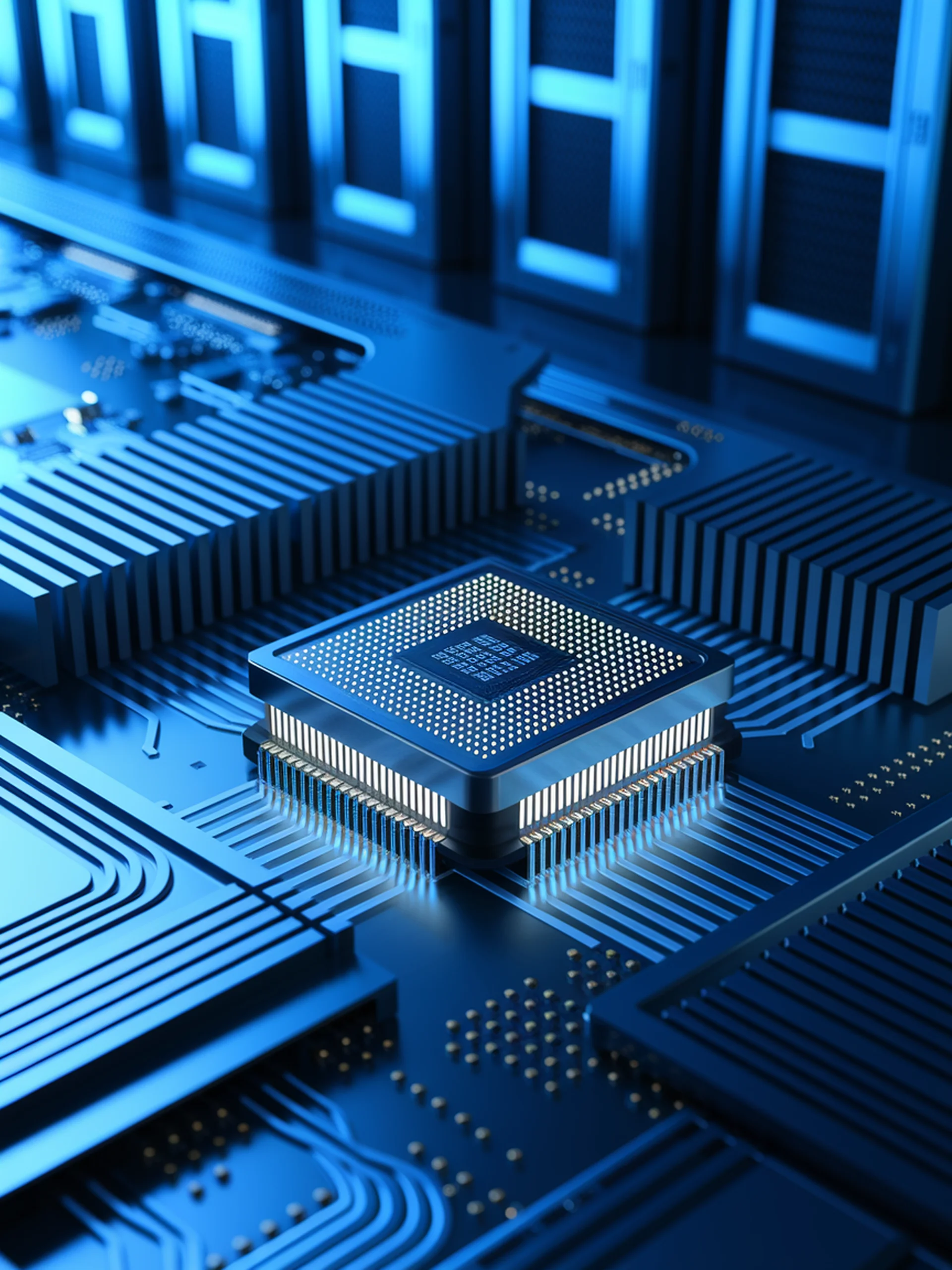
Smarter LLM Compression
Novel quantization technique maintains accuracy while reducing model size
LRQ (Low-Rank Quantization) introduces a post-training method to compress Large Language Models while preserving performance on complex tasks.
- Addresses accuracy drops in traditional quantization approaches
- Uses learned low-rank weight-scaling matrices to optimize compression
- Effectively balances model efficiency with maintained performance
- Particularly valuable for deployment in resource-constrained environments
This engineering breakthrough enables more efficient LLM deployment with lower inference costs, making advanced AI capabilities more accessible across various computing environments.