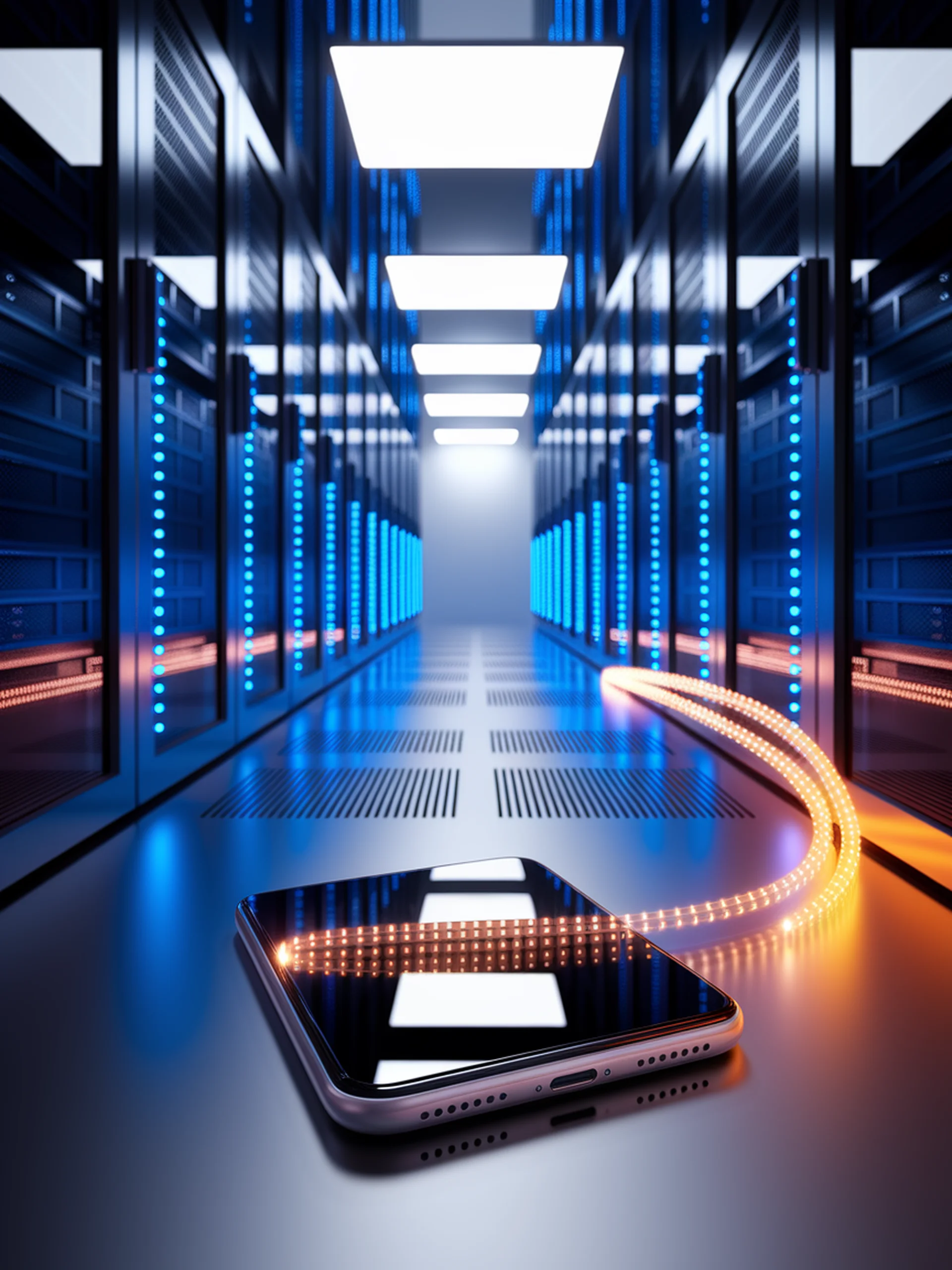
Optimizing Image Transfer for Cloud-Based MLLMs
A novel framework for efficient compressed image latents
This research introduces a framework to efficiently adapt compressed image latents for Multimodal Large Language Models (MLLMs), enabling practical deployment scenarios where devices can send optimized data to cloud-based AI systems.
- Proposes a transform-neck architecture that bridges compressed images with MLLMs
- Introduces a surrogate loss that improves model performance without requiring end-to-end training
- Demonstrates significant bandwidth savings while maintaining competitive performance on downstream tasks
- Offers a practical solution for resource-constrained devices to leverage powerful cloud MLLMs
This engineering breakthrough matters because it addresses a critical bottleneck in deploying AI systems across device-cloud boundaries, making sophisticated multimodal AI more accessible and practical for real-world applications.
Bridging Compressed Image Latents and Multimodal Large Language Models