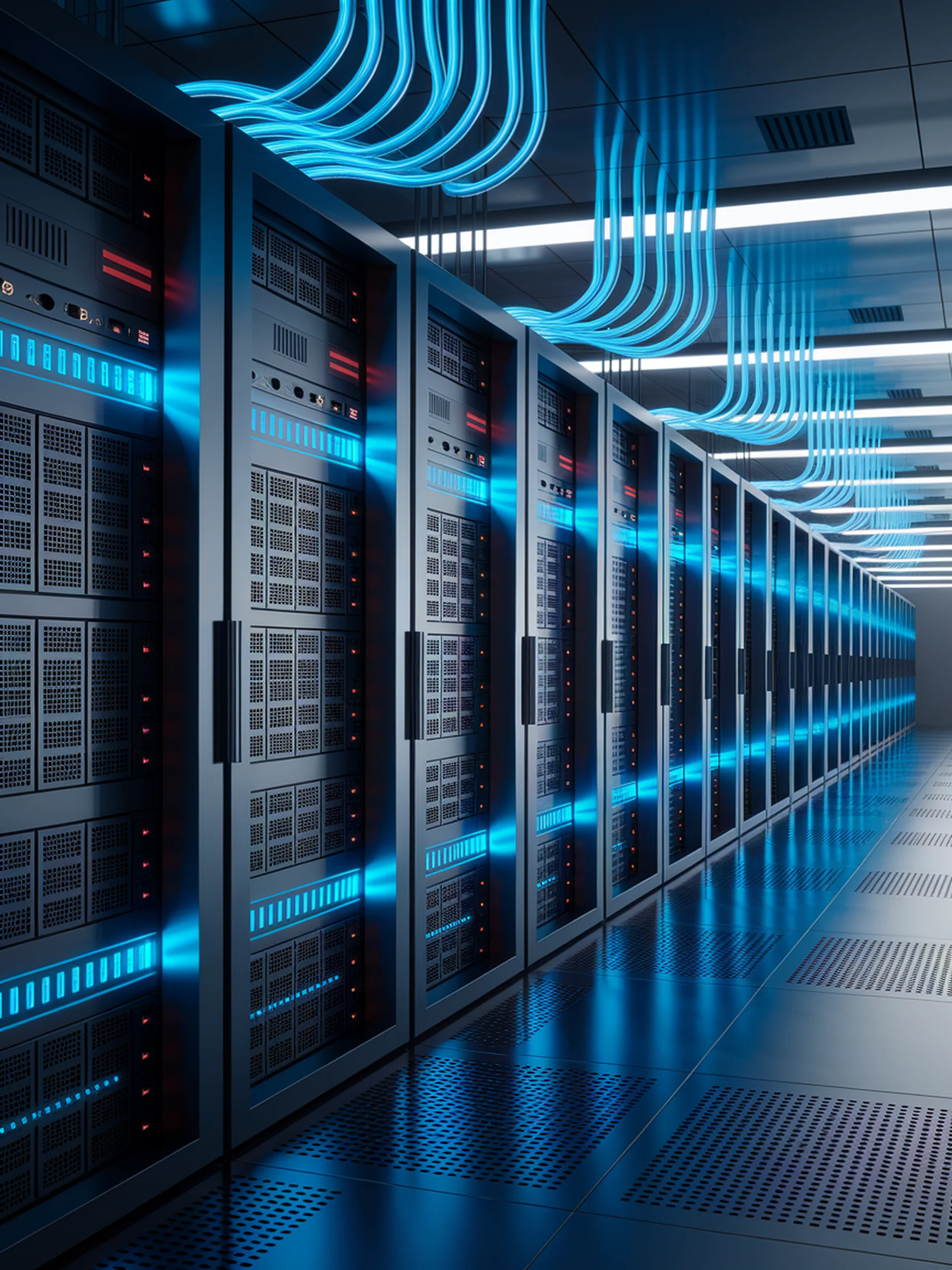
Memory Optimization for LLM Inference
Network-Accelerated Memory Offloading for Scalable GPU Deployments
AQUA introduces a novel approach to handling memory constraints for large language models by efficiently offloading memory across networked GPUs.
- Enables preemptive scheduling of prompts in time slices to maintain responsiveness during request bursts
- Eliminates traditional admission control limitations that cause unresponsiveness
- Delivers 2.8-3.5× higher throughput compared to state-of-the-art memory offloading techniques
- Achieves performance within 10-15% of all-in-GPU inference even with memory constraints
This research addresses a critical engineering challenge for cloud LLM deployments, allowing services to handle sudden traffic spikes without degradation in user experience or responsiveness.
AQUA: Network-Accelerated Memory Offloading for LLMs in Scale-Up GPU Domains