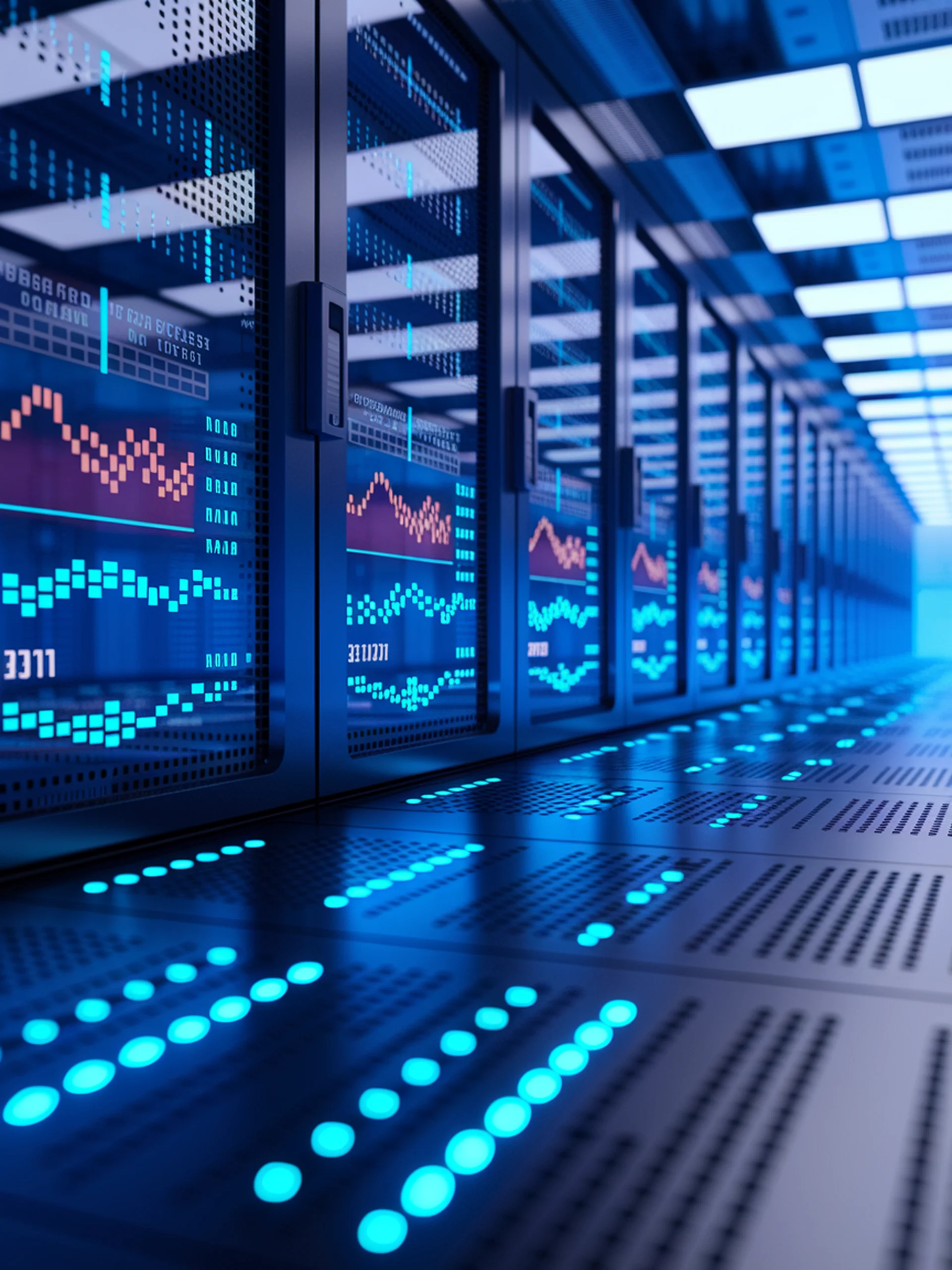
Optimizing LLM Performance for Business Applications
Intelligent Performance Tuning for Large Language Model Services
SCOOT introduces a novel approach to optimize Service-Level Objectives (SLOs) in LLM inference engines through adaptive parameter tuning.
- Customizes parameter configurations based on specific service requirements
- Employs Bayesian optimization with efficient search space pruning
- Achieves superior performance compared to default configurations
- Enhances user satisfaction while improving resource efficiency
This research provides engineering teams with a systematic method to optimize LLM performance in production environments, helping businesses deliver more responsive AI services while controlling infrastructure costs.
SCOOT: SLO-Oriented Performance Tuning for LLM Inference Engines