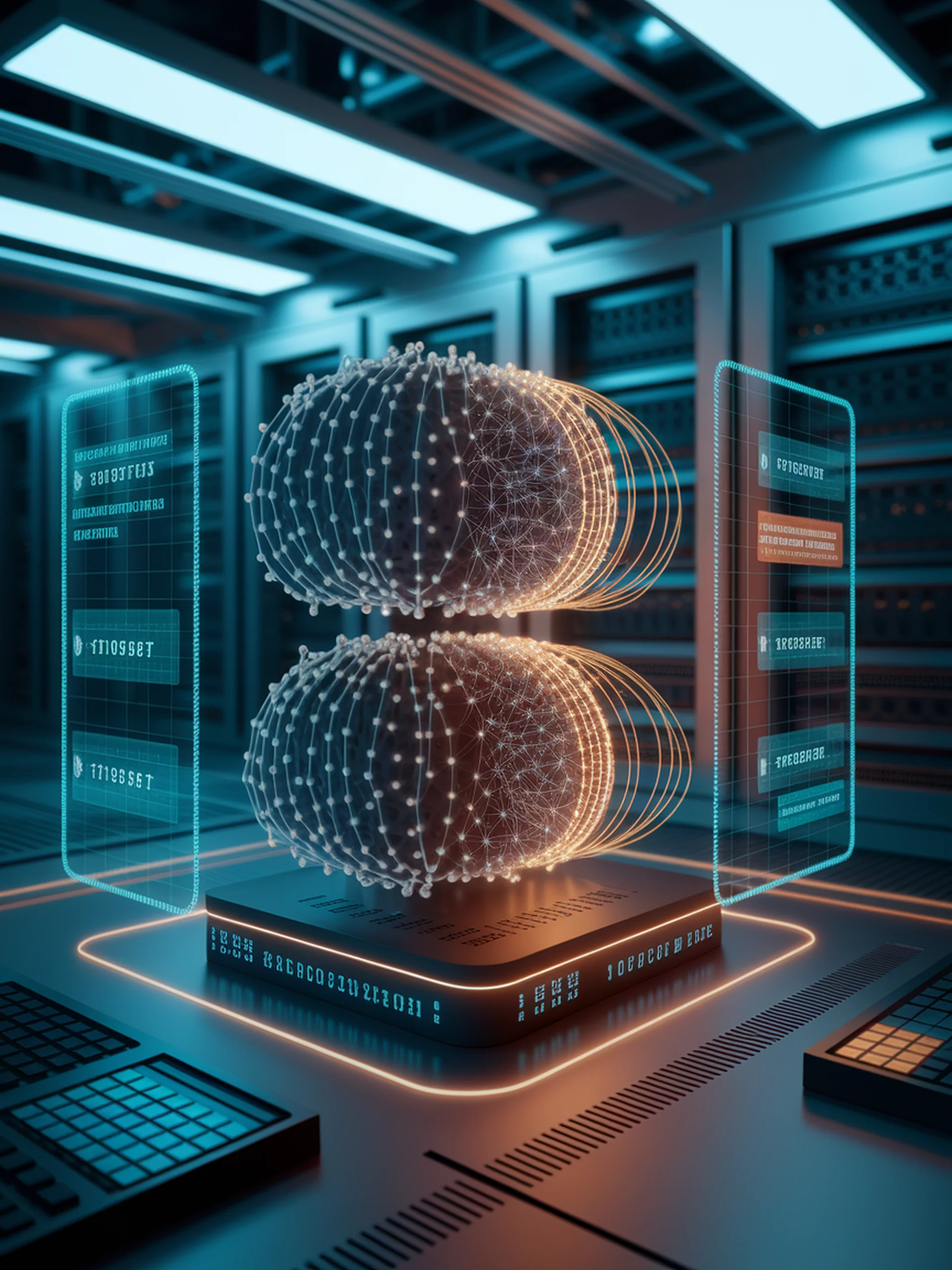
Shrinking Giants: Efficient LLM Compression
A modular approach that reduces model size without sacrificing performance
MoDeGPT introduces a novel modular decomposition framework that compresses Large Language Models while maintaining accuracy and minimizing inference overhead.
- Achieves over 2x compression with negligible accuracy loss
- Uses structured matrix decomposition to preserve model architecture
- Outperforms existing compression methods in accuracy-efficiency tradeoffs
- Demonstrates practical deployment benefits with reduced memory footprint
This engineering breakthrough addresses a critical barrier to LLM deployment on resource-constrained devices, making advanced AI more accessible and cost-effective for real-world applications.
MoDeGPT: Modular Decomposition for Large Language Model Compression