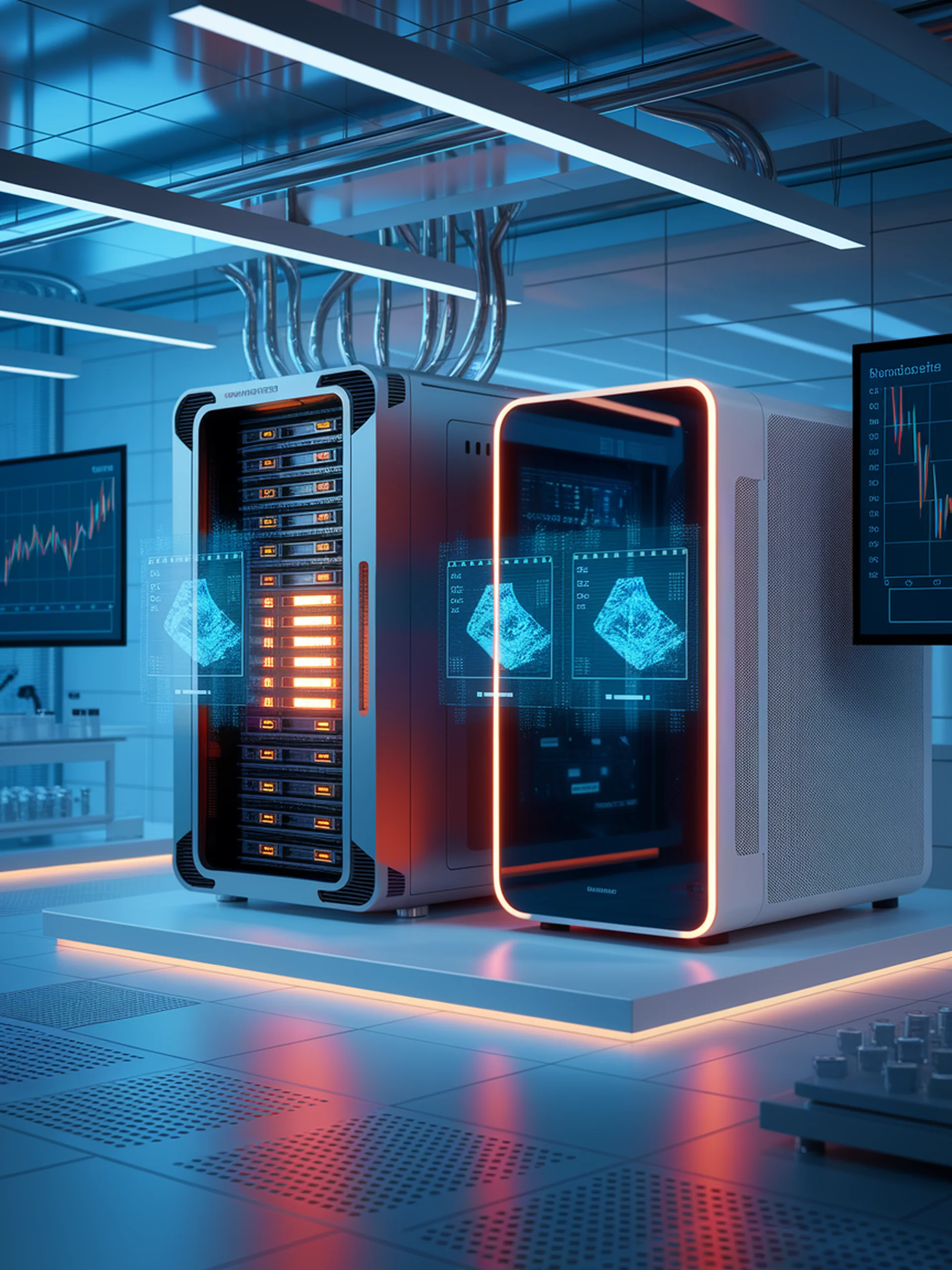
Balancing Efficiency in Multimodal LLMs
A novel approach that optimizes both data and computational resources
EE-MLLM introduces an innovative architecture that eliminates the traditional trade-off between data and computational efficiency in multimodal large language models.
- Addresses limitations in both self-attention and cross-attention approaches
- Achieves superior performance with fewer training samples
- Reduces computational requirements while maintaining high accuracy
- Provides a more sustainable approach to building powerful vision-language models
This engineering breakthrough matters because it enables more resource-efficient deployment of multimodal AI systems, making advanced visual reasoning capabilities accessible with lower infrastructure costs and environmental impact.
EE-MLLM: A Data-Efficient and Compute-Efficient Multimodal Large Language Model