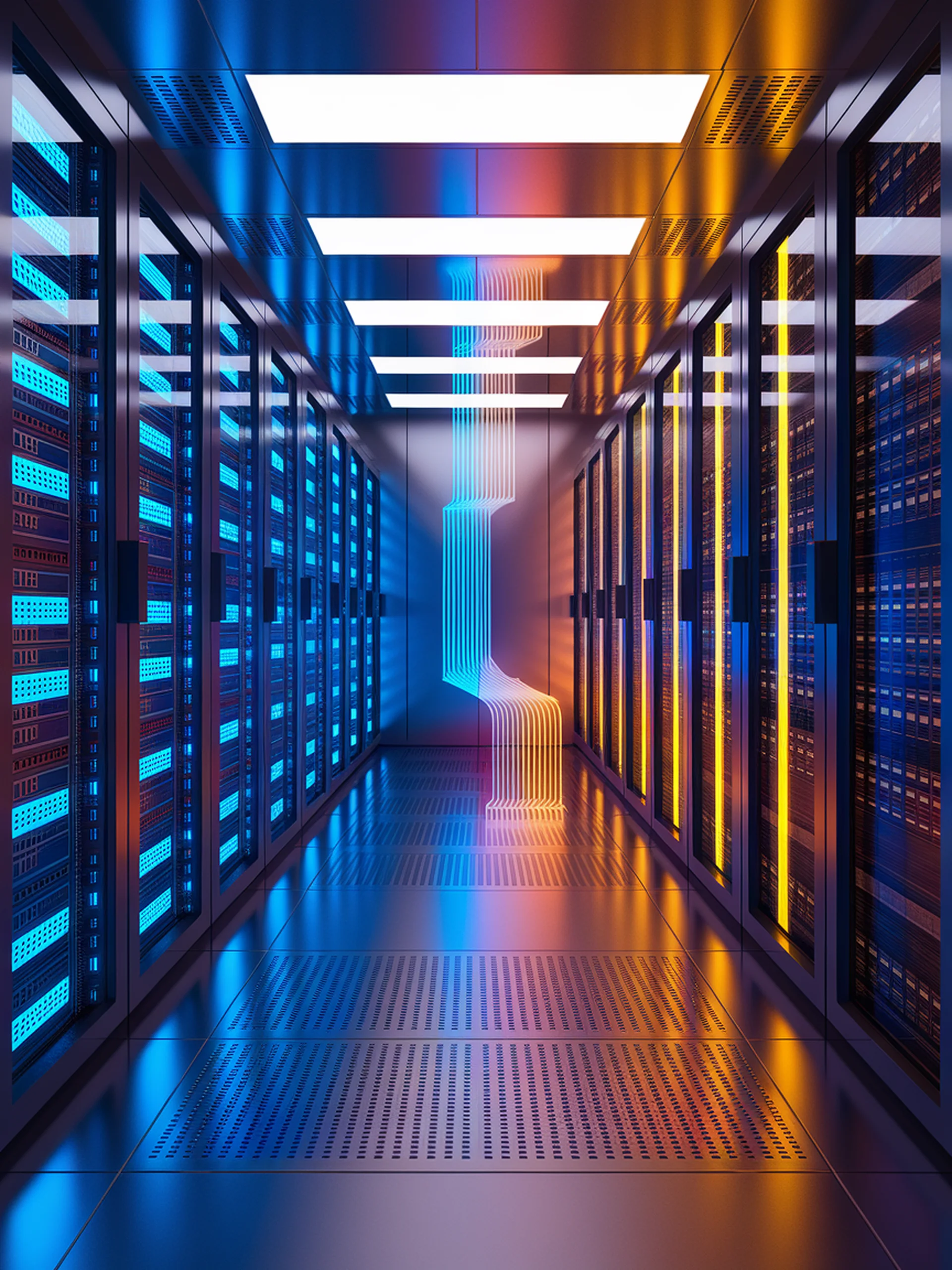
Optimizing LLM Inference with Hybrid KV Cache Management
A Dynamic Approach to Balance Computing and Loading for Better Performance
This research introduces a novel system that intelligently balances computation and loading of key-value (KV) caches to optimize large language model inference, particularly for long-context scenarios.
- Addresses the bottleneck of KV cache generation in the prefill stage
- Dynamically decides whether to compute KV caches or load them from storage
- Achieves significant latency reduction compared to traditional approaches
- Enables more efficient scaling of LLM-powered applications
This engineering advancement is crucial for deploying LLMs at scale in production environments, where performance optimization directly impacts user experience and operational costs.