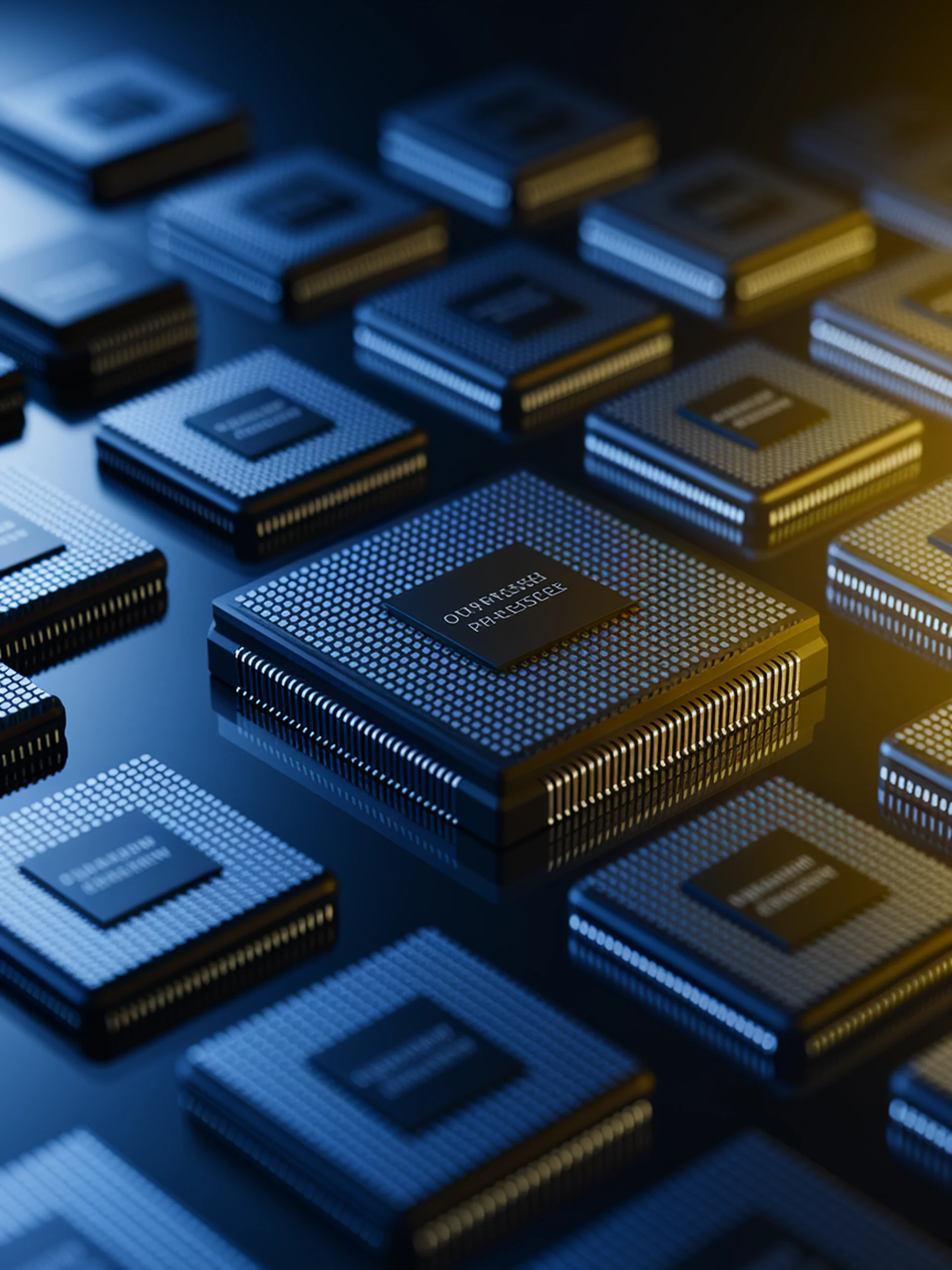
Smarter Model Compression with PrefixQuant
Eliminating token-wise outliers for superior LLM quantization
PrefixQuant introduces a novel approach to LLM compression that achieves state-of-the-art performance by targeting both channel-wise and token-wise outliers.
- Isolates problematic token-wise outliers to improve quantization accuracy
- Achieves exceptional performance across multiple precision levels (W4A4KV4, W4A8KV4)
- Works effectively with both dynamic and static quantization approaches
- Maintains model quality while significantly reducing computational requirements
This engineering breakthrough matters because it enables more efficient deployment of large language models on resource-constrained devices, expanding the practical applications of AI while reducing energy consumption and hardware costs.
PrefixQuant: Eliminating Outliers by Prefixed Tokens for Large Language Models Quantization