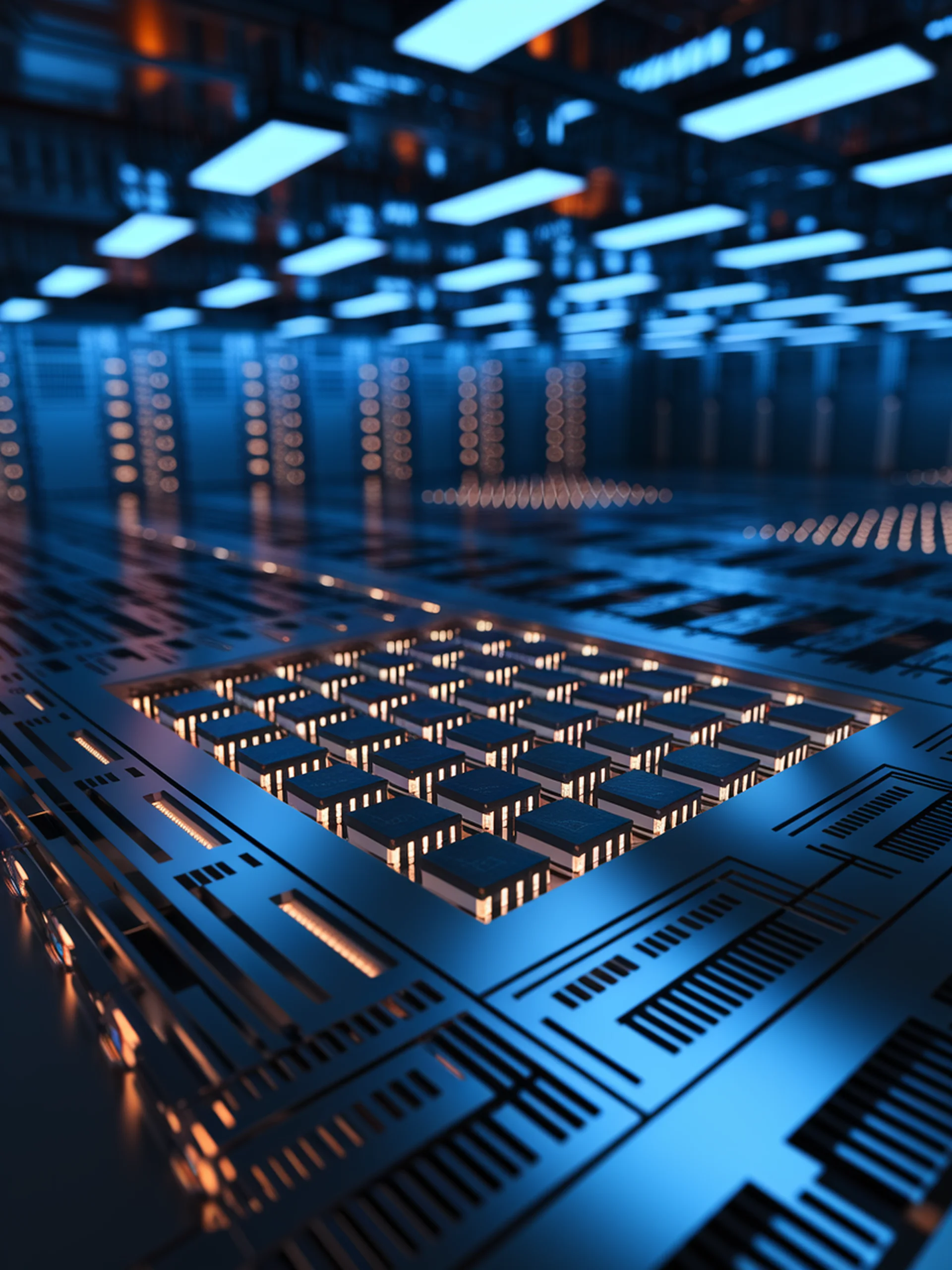
Streamlining MoE Language Models
Reducing redundancy and memory footprint in Mixture-of-Experts LLMs
This research addresses key efficiency challenges in Mixture-of-Experts (MoE) language models by introducing innovative compression techniques that maintain performance while reducing resource requirements.
- Mixture Compressor: Novel approach that intelligently compresses experts based on their activation patterns
- Parameter Reduction: Achieves significant memory savings through expert compression and redundancy elimination
- Performance Preservation: Maintains model capabilities despite reduced parameters
- Practical Application: Enables more efficient deployment of large language models in resource-constrained environments
This engineering advancement makes cutting-edge MoE models more practical for real-world deployment by addressing their two biggest limitations: excessive memory consumption and redundant expert activations.