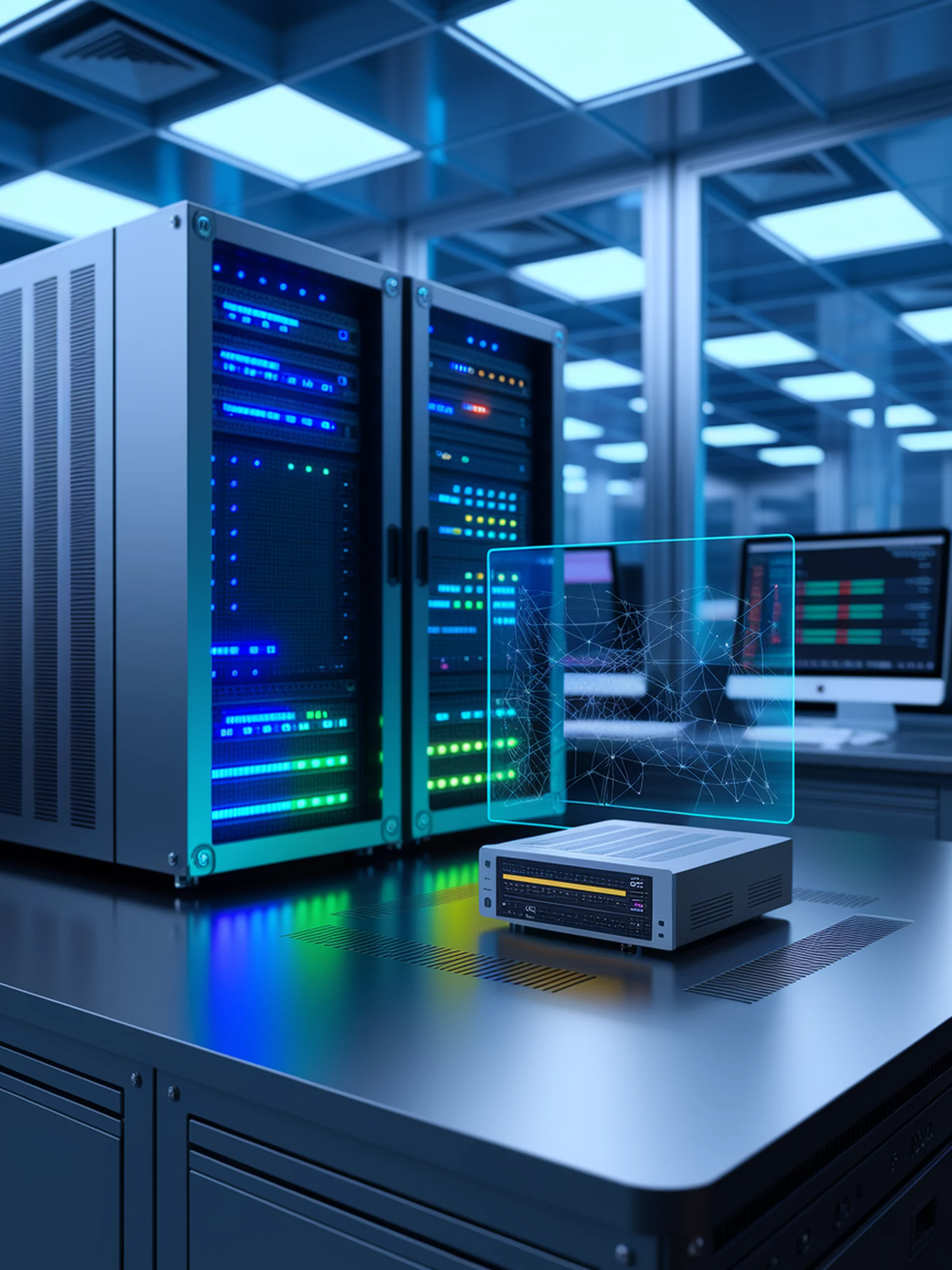
Shrinking LLMs without Sacrificing Performance
Automated techniques to make large language models smaller and faster
This research presents a novel automated compression approach that reduces the size of large language models while maintaining or improving their performance.
- Frames compression as a neural architecture search problem that automatically prunes less important model components
- Achieves significant size reduction while maintaining or improving downstream task performance
- Reduces inference costs which accumulate substantially over a model's lifecycle
- Makes powerful AI more accessible by lowering computational requirements
This engineering breakthrough addresses one of AI's critical challenges: making sophisticated models practical for wider deployment without sacrificing capabilities.
Compressing Large Language Models with Automated Sub-Network Search