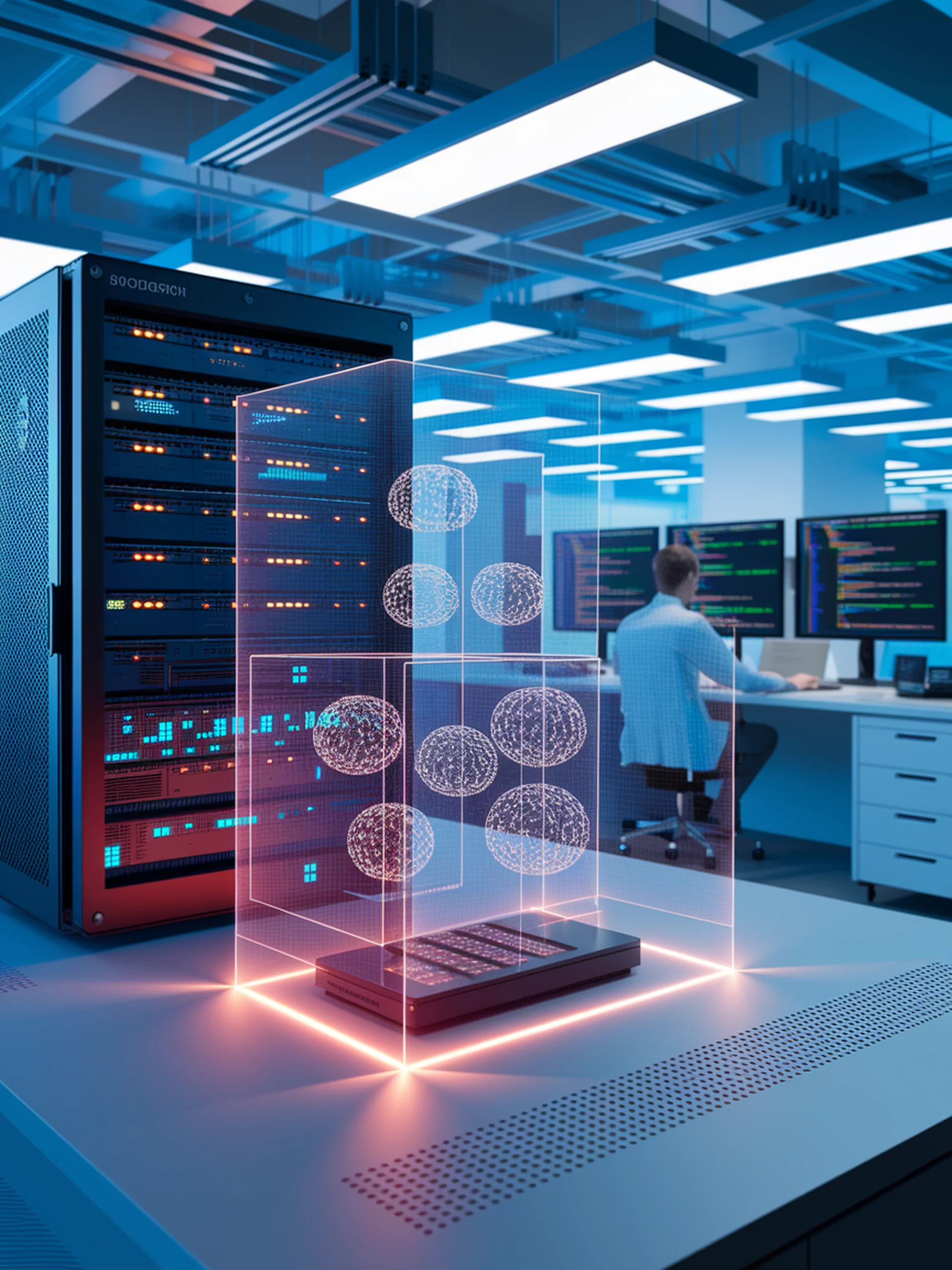
Optimizing Sparse MoE Models
Merging experts through hierarchical clustering without retraining
A novel approach that enables efficient compression of Sparse Mixture-of-Experts (SMoE) large language models for resource-constrained environments.
- Reduces memory requirements by clustering and merging similar experts
- Eliminates costly retraining after model compression
- Maintains performance while reducing parameter count
- Enables deployment of powerful SMoE models on devices with limited resources
This engineering breakthrough makes advanced LLMs more accessible across a wider range of deployment scenarios, potentially democratizing access to state-of-the-art AI capabilities.
Retraining-Free Merging of Sparse MoE via Hierarchical Clustering