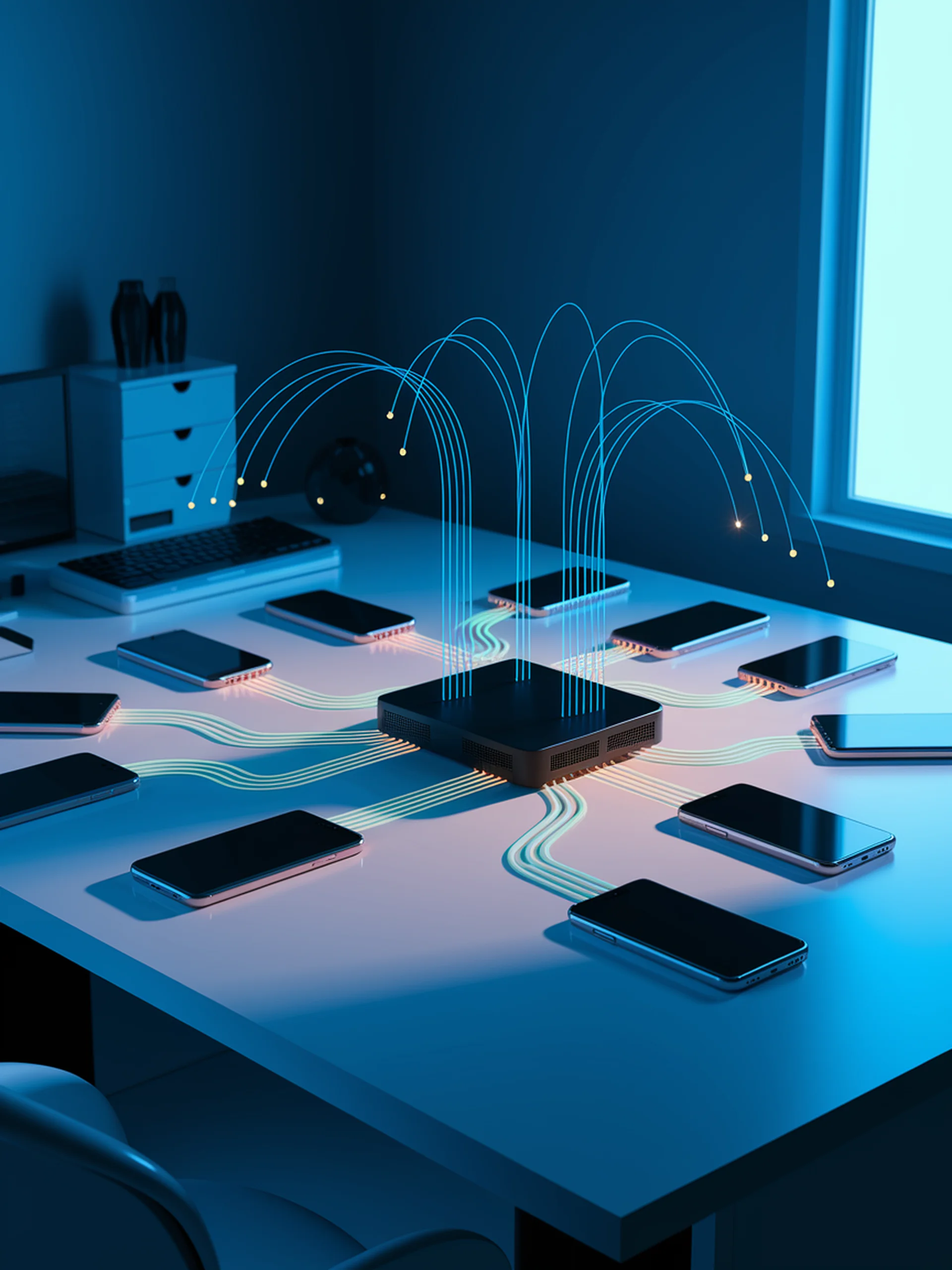
DistRL: Scaling Control Agents for Mobile Devices
Distributed Reinforcement Learning Framework for On-Device Intelligence
DistRL introduces an asynchronous distributed reinforcement learning framework specifically engineered for mobile control agents, enabling efficient training of Multimodal LLMs with limited data.
- Centralized training, decentralized data collection architecture that optimizes resource usage
- Asynchronous experience sharing between devices to accelerate learning
- Improved training efficiency through customized reinforcement learning algorithms tailored for mobile constraints
- Enhanced user experience by enabling devices to learn complex commands and control patterns
This engineering breakthrough addresses the critical challenges of deploying sophisticated AI control agents on resource-constrained mobile devices, making advanced device automation more accessible.
DistRL: An Asynchronous Distributed Reinforcement Learning Framework for On-Device Control Agents