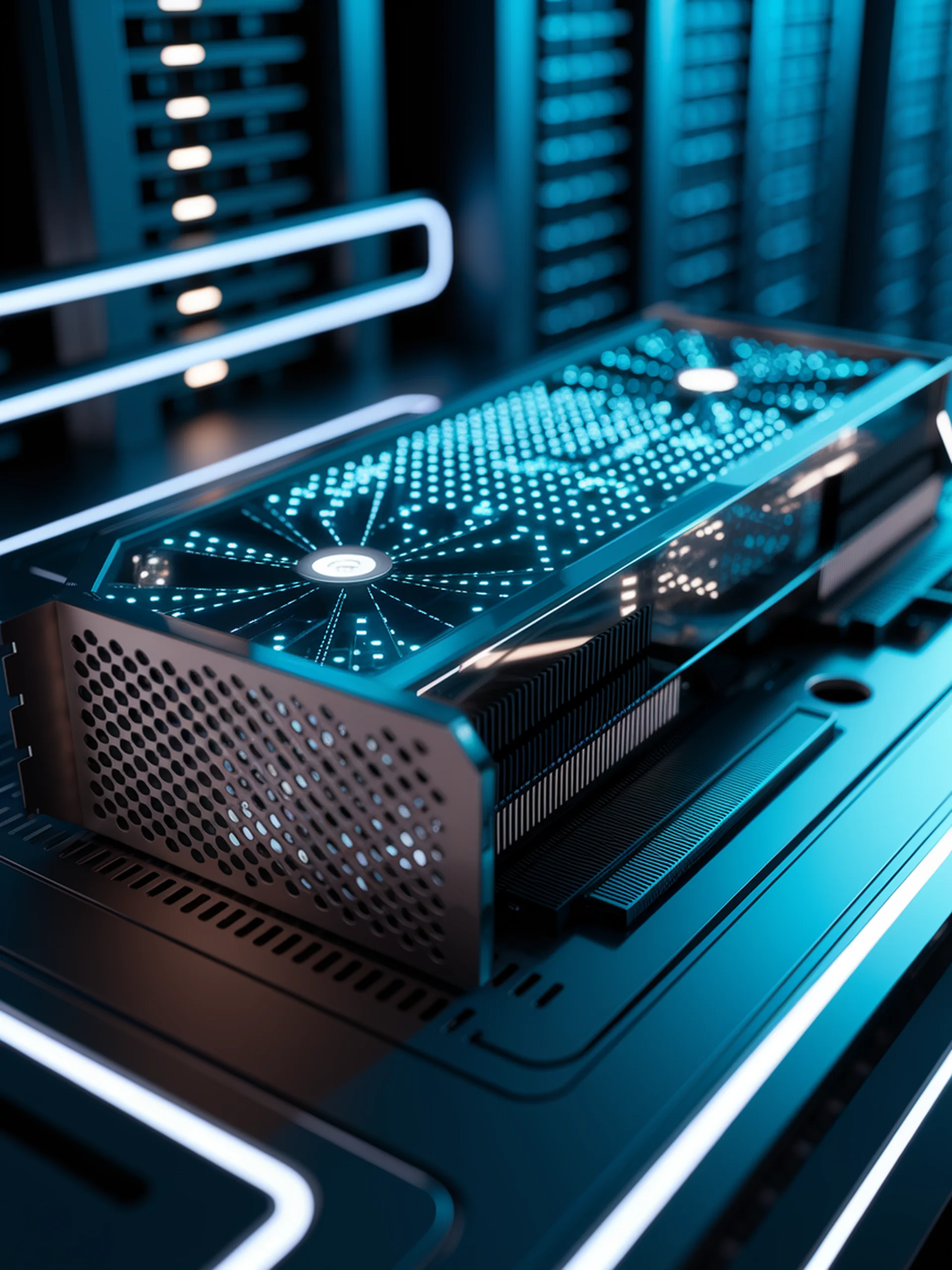
Breaking the 2:4 Barrier in GPU Sparsity
Unlocking V:N:M sparse patterns for faster transformer inference
This research explores V:N:M sparsity as an alternative to traditional 2:4 sparsity patterns, enabling more efficient transformer model inference on GPUs.
- Overcomes the limitations of 2:4 sparsity, which typically offers modest speedups (≤1.3×) and restricts sparse ratios
- Enables flexible sparse patterns beyond 50% sparsity (e.g., 4:8, 8:16)
- Achieves significant acceleration for transformer models through optimized sparse patterns
- Demonstrates practical implementation techniques for GPU hardware
For engineering teams, this work provides valuable patterns to improve inference efficiency on existing GPU infrastructure, potentially reducing compute costs while maintaining model performance.
Beyond 2:4: exploring V:N:M sparsity for efficient transformer inference on GPUs