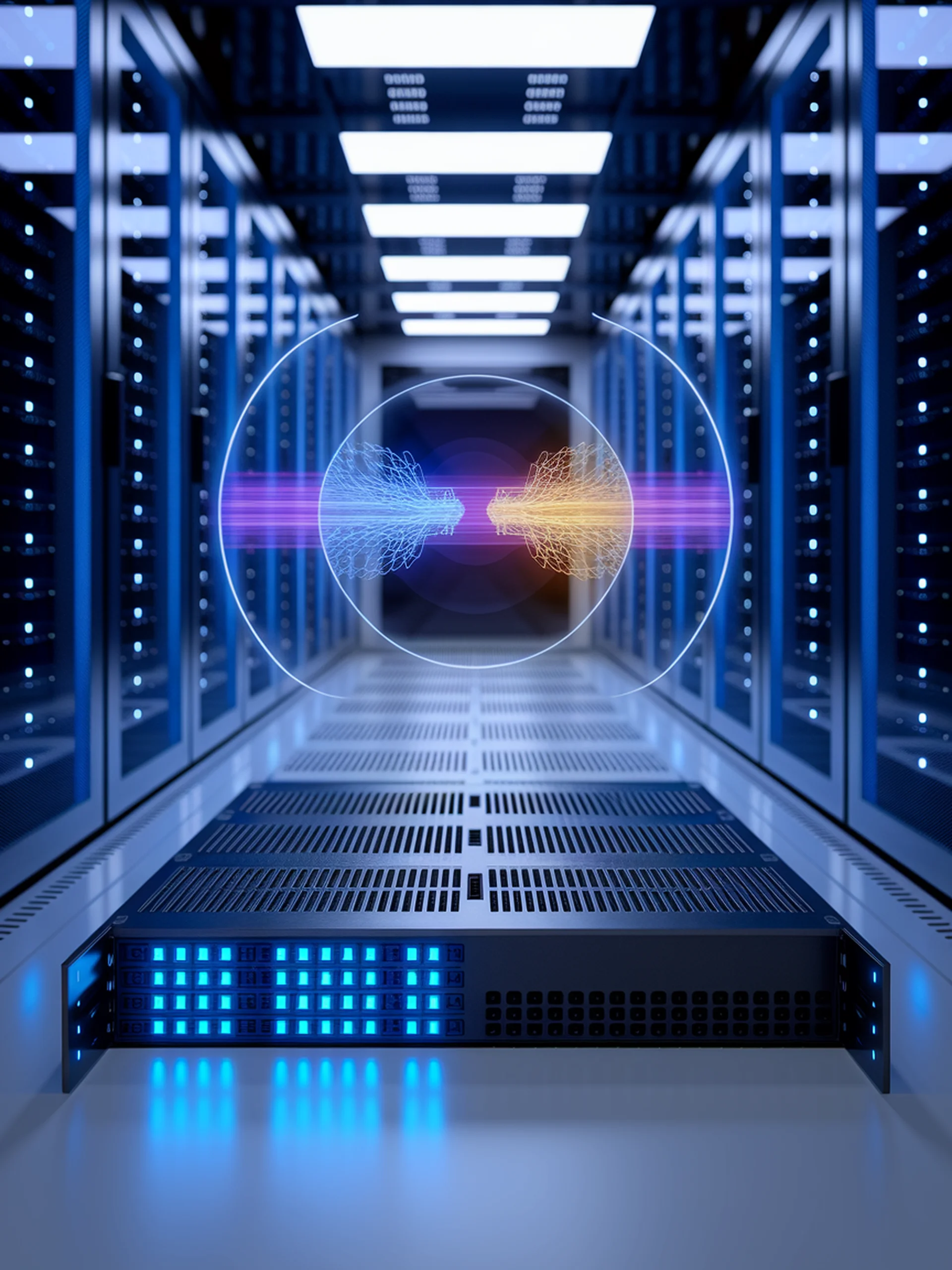
Efficient LLM Compression
Combining LoRA and Knowledge Distillation for Smarter AI Compression
LLM-NEO introduces a parameter-efficient approach that bridges knowledge distillation with Low-Rank Adaptation to significantly improve LLM compression.
- Reveals the shared paradigm between Knowledge Distillation and LoRA techniques
- Delivers parameter-efficient knowledge transfer requiring fewer resources
- Provides practical hyperparameter guidelines for optimizing compression
- Demonstrates how integrating these approaches yields better efficiency than either method alone
This engineering breakthrough enables smaller, more deployable AI systems while preserving performance, making advanced language models more accessible for real-world applications with limited computational resources.
LLM-NEO: Parameter Efficient Knowledge Distillation for Large Language Models