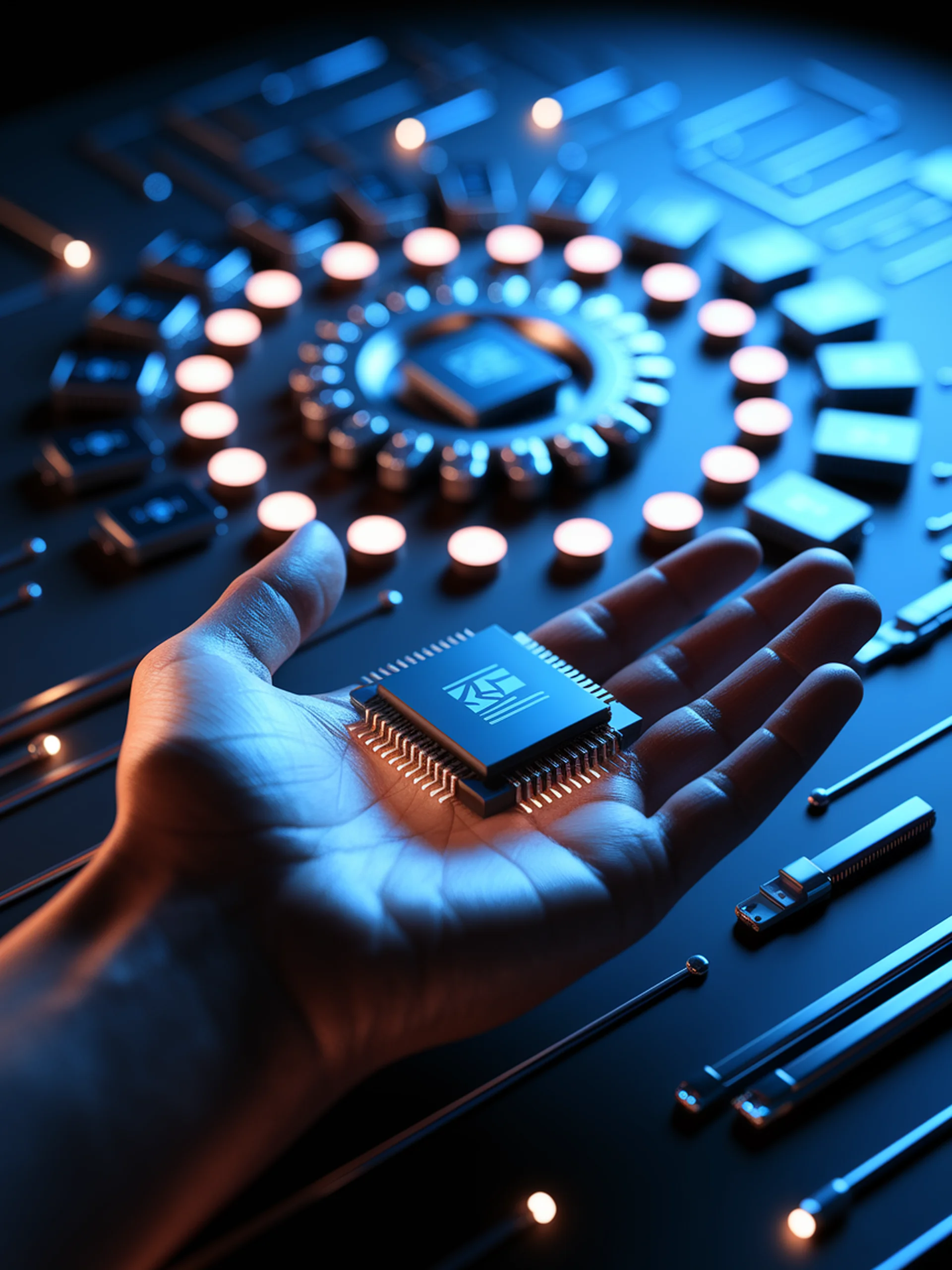
Bringing LLMs to Resource-Constrained Devices
Efficient federation learning techniques for tiny transformers
This research demonstrates how to effectively finetune large language models on devices with limited computational resources.
Key Innovations:
- Novel parameter-efficient techniques beyond Adapter and LoRA for resource-constrained devices
- Federated learning approach that preserves privacy while enabling collaborative model improvement
- Significantly reduced FLOPs and memory requirements for transformer finetuning
- Engineering solutions that make advanced AI accessible on everyday devices
Why It Matters: As AI capabilities expand, enabling powerful language models to run effectively on edge devices opens new possibilities for secure, private, and accessible AI applications without constant cloud connectivity.
Efficient Federated Finetuning of Tiny Transformers with Resource-Constrained Devices