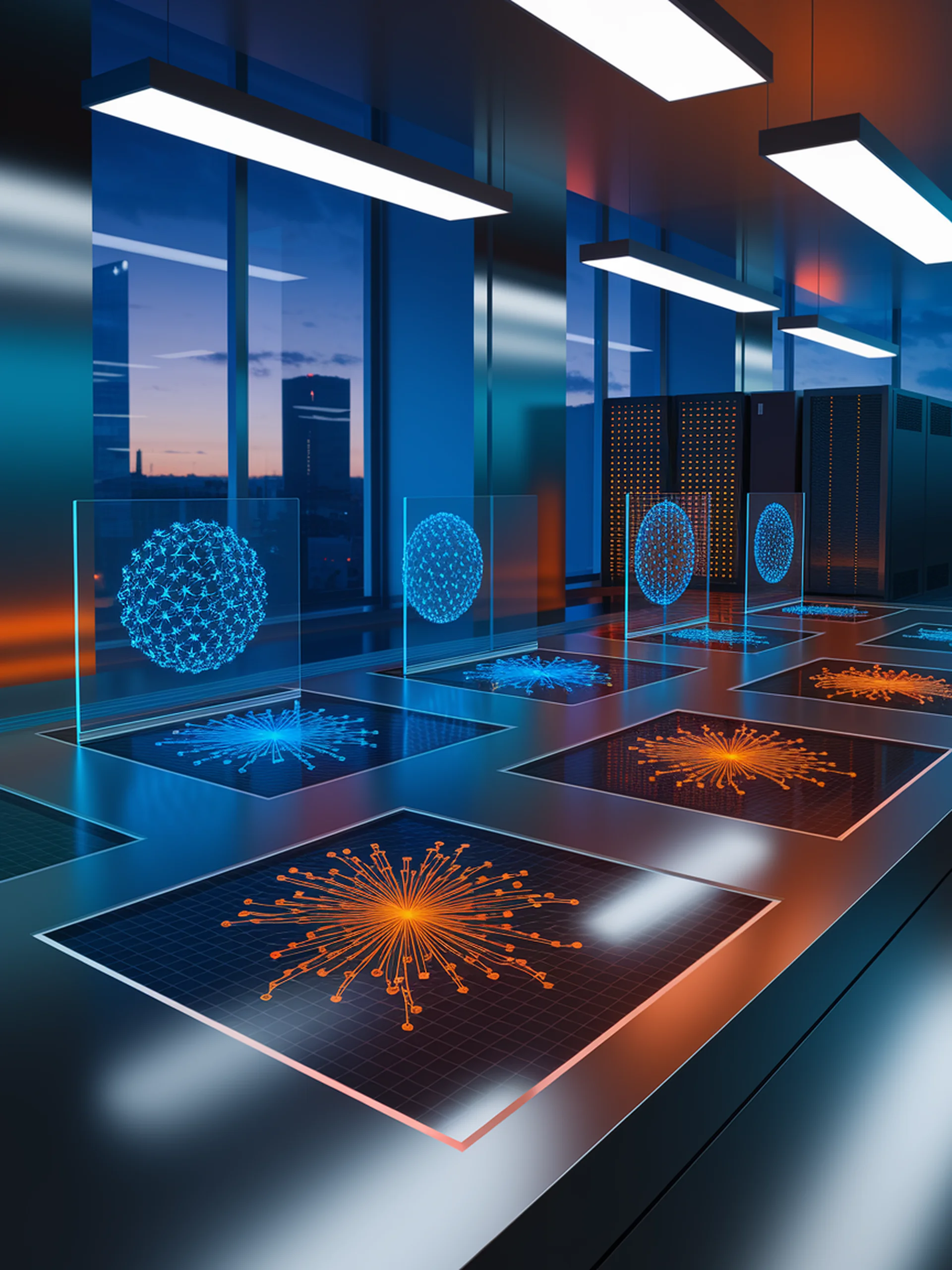
Optimizing LLM Reasoning with Sparse Attention
Reducing computational costs while maintaining reasoning quality
This research introduces a sparse attention mechanism that significantly reduces the computational demands of chain-of-thought reasoning in large language models.
- Focuses attention only on the most relevant tokens during reasoning tasks
- Demonstrated effectiveness on MIT linear algebra problems
- Achieves comparable accuracy with lower computational costs
- Uses custom GPT models (GiantRabbit) as experimental framework
For engineering teams, this approach represents a practical path to implementing sophisticated reasoning in LLMs without prohibitive computational overhead, potentially enabling more efficient deployment of reasoning-capable AI systems.