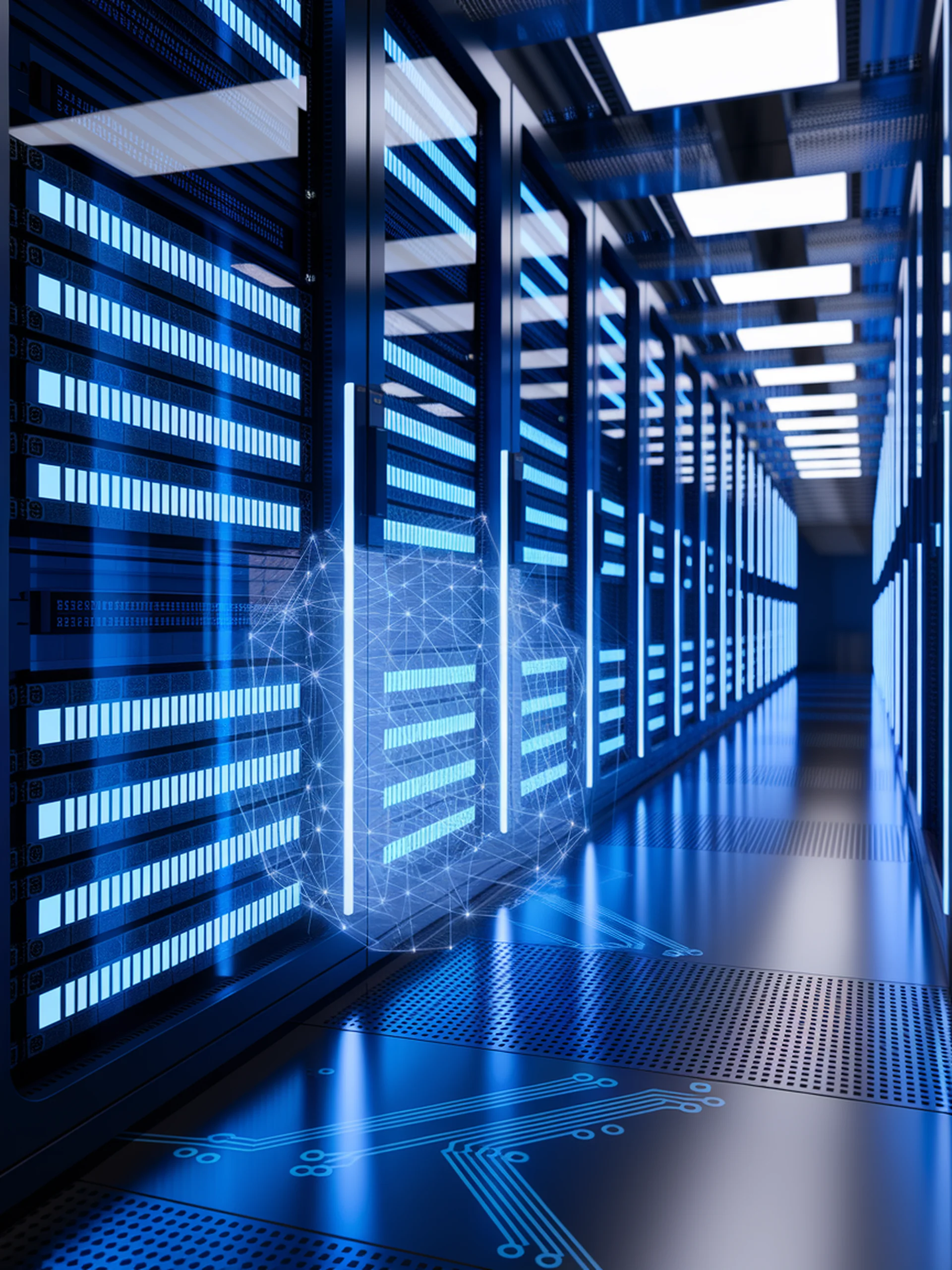
Smarter Memory Usage for AI Training
Correlation-Aware Projection Reduces Memory Needs While Preserving Performance
COAP introduces a new approach for memory-efficient training of large neural networks by intelligently projecting gradients based on parameter correlation.
- Reduces optimizer memory footprint by up to 8× compared to full fine-tuning
- Outperforms other memory-efficient methods like LoRA and GaLore
- Maintains performance comparable to full fine-tuning across vision and multimodal domains
- Particularly valuable for training large-scale models with limited computational resources
This engineering breakthrough enables more efficient development of large AI models on standard hardware, making advanced AI research more accessible to teams without massive computing infrastructure.
COAP: Memory-Efficient Training with Correlation-Aware Gradient Projection