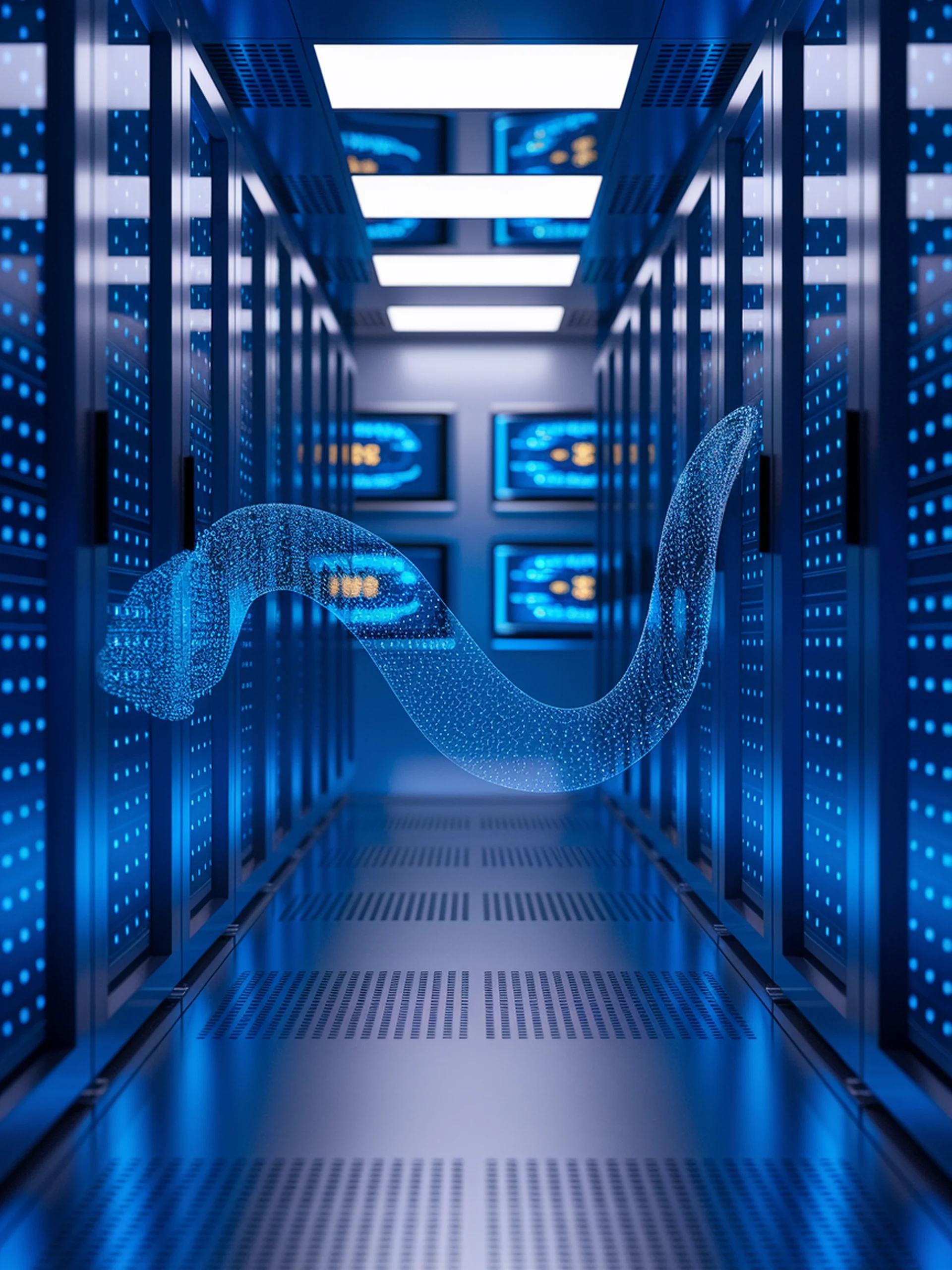
Accelerating LLMs with Separator Compression
Reducing computational demands by transforming segments into separators
SepLLM introduces a novel technique that drastically improves inference speed in large language models by compressing text segments into separator tokens.
- Leverages the discovery that separator tokens (like punctuation) contribute disproportionately to attention scores
- Reduces computational complexity by compressing semantically meaningful segments into single separator tokens
- Achieves faster inference while maintaining model performance and quality
- Offers a practical engineering solution to the challenge of LLM scalability
This research provides a significant engineering advancement for deploying more efficient LLMs in production environments, addressing critical concerns around computational resource demands.
SepLLM: Accelerate Large Language Models by Compressing One Segment into One Separator