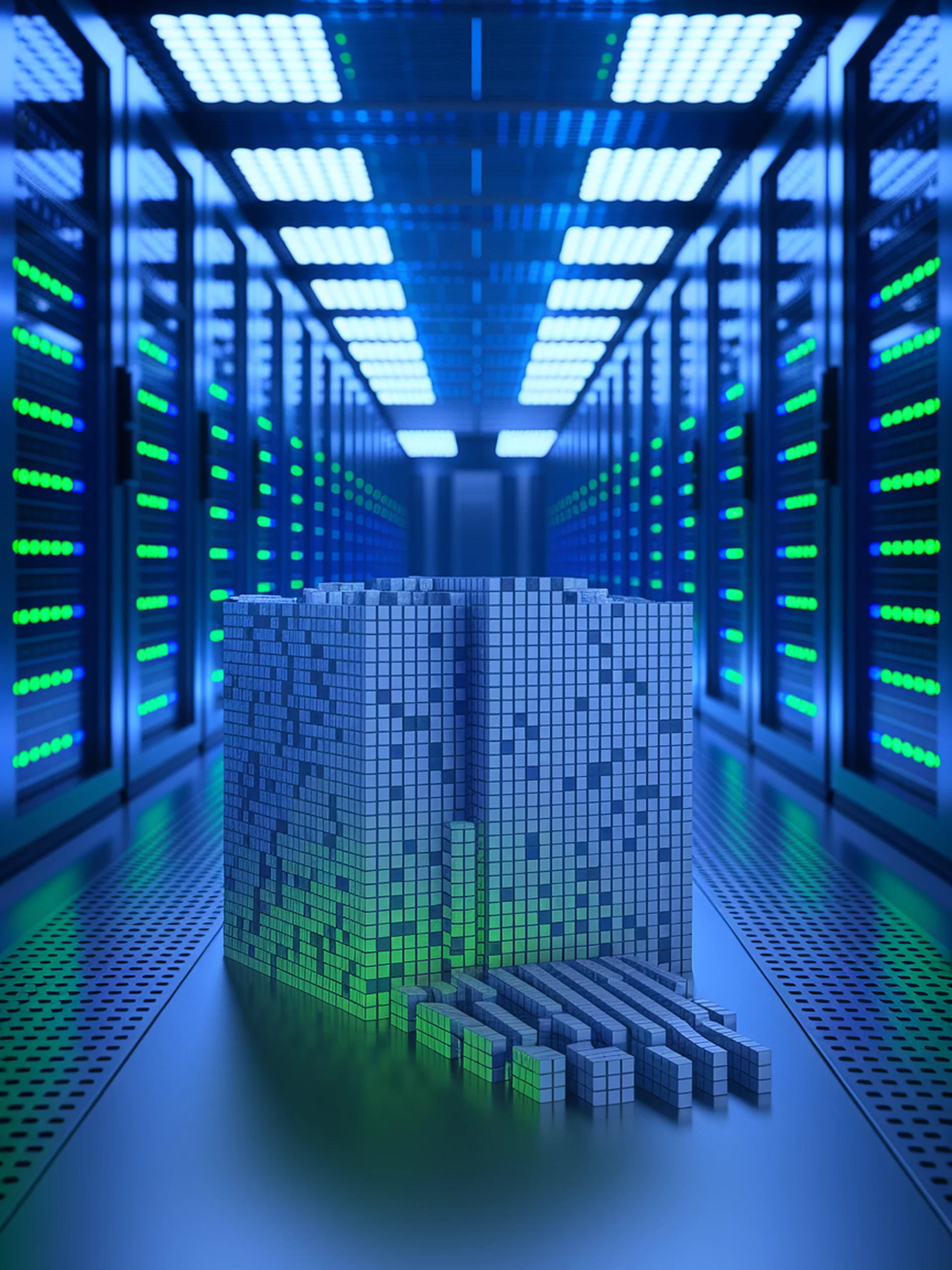
Optimizing KV Cache for Efficient LLMs
Finding the Sweet Spot Between Token Reduction and Precision
This research explores the optimal trade-off between token count and precision in KV cache compression to overcome memory bottlenecks in large language models.
- Identifies that jointly optimizing tokens and precision yields superior results compared to focusing on either dimension alone
- Introduces a comprehensive evaluation framework for token-precision trade-offs
- Demonstrates that optimal compression varies across different models and tasks
- Shows potential for significantly reduced memory usage while maintaining performance
For engineering teams, this work offers practical insights for deploying larger context windows in LLMs with limited computational resources, potentially enabling more efficient inference in production environments.
More Tokens, Lower Precision: Towards the Optimal Token-Precision Trade-off in KV Cache Compression