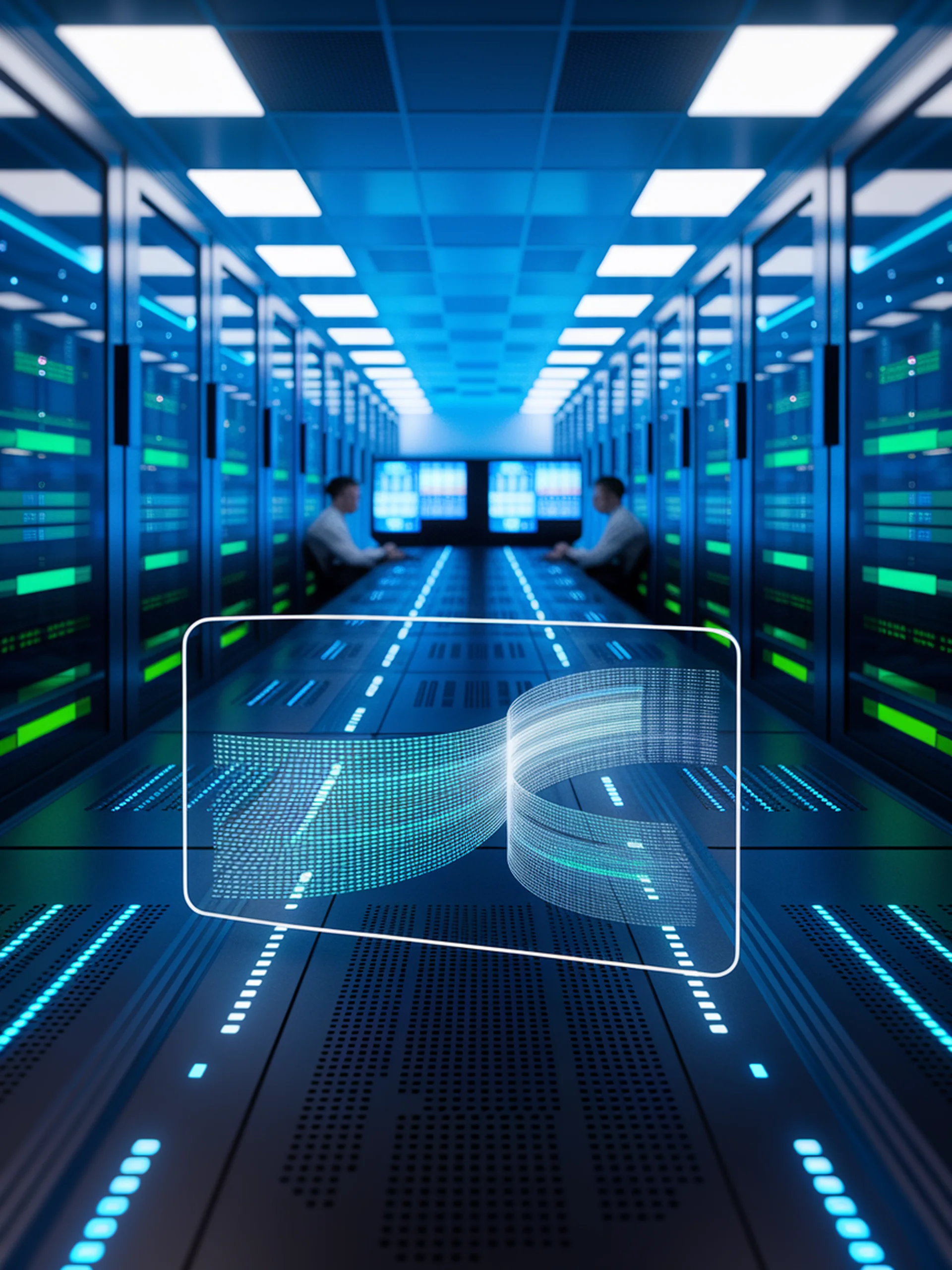
Memory-Efficient LLM Training
A stateless optimizer that reduces memory footprint while maintaining performance
SWAN is a novel stateless optimizer that eliminates the need to store optimizer states during LLM training, significantly reducing memory requirements without sacrificing model quality.
- Combines SGD with normalization and whitening techniques
- Achieves performance comparable to Adam while using significantly less memory
- Enables training of larger models with the same computational resources
- Improves scalability for distributed LLM training
This engineering breakthrough addresses a critical bottleneck in LLM development by making training more efficient and accessible, potentially accelerating innovation in AI by allowing researchers to train larger models with limited resources.
SWAN: SGD with Normalization and Whitening Enables Stateless LLM Training