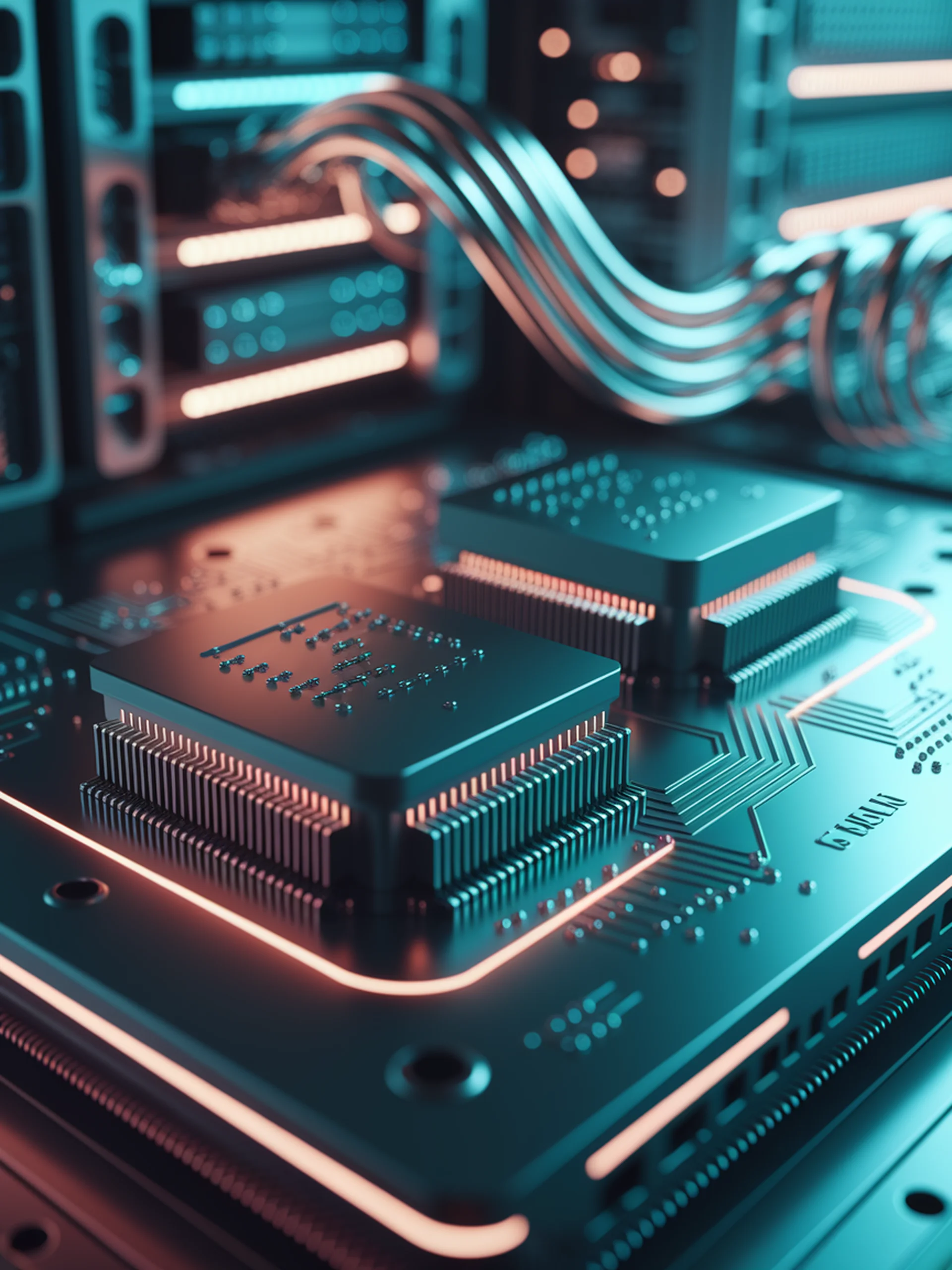
ResQ: Efficient LLM Quantization
Boosting 4-bit Quantization Performance with Low-Rank Residuals
ResQ introduces a novel post-training quantization (PTQ) technique that enables high-compression of LLMs while maintaining performance quality.
- Addresses the challenge of extreme outliers in activations that typically degrade model performance during quantization
- Employs low-rank residual approximation to efficiently represent complex weight and activation tensors
- Achieves 4-bit quantization of weights, activations, and KV cache without significant performance loss
- Delivers substantial memory reduction and inference acceleration for large language model deployment
This engineering advancement matters because it makes LLMs more accessible for deployment on resource-constrained devices and reduces computational costs during inference.
ResQ: Mixed-Precision Quantization of Large Language Models with Low-Rank Residuals